A Novel Approach for Enhancing Customer Retention Using Machine Learning Techniques in Email Marketing Application
2023 2nd International Conference on Paradigm Shifts in Communications Embedded Systems, Machine Learning and Signal Processing (PCEMS)(2023)
摘要
Customers are becoming more concerned about the QoS (Quality of Service) that businesses can give them in today’s modern world. Because the services offered by many vendors are not very distinguishable, businesses have increased rivalry to maintain and improve their quality of service. Customer Relationship Management (CRM) systems are used to provide businesses with the capacity to boost their profitability by acquiring new consumers, establishing a continuous relationship with existing customers, as well as keeping more of their existing customers. Machine Learning (ML) algorithms are used in CRM (Customer Relationship Management) systems to evaluate personal & behavioral data from clients. This gives a company a competitive edge by improving the percentage of consumers they keep as clients. This research attempts to evaluate and assess the performance of several machine learning (ML) approaches to solve the subscriber prediction issue in email marketing. Different analytical machine learning methods that belong to diverse types of learning are selected for this work, especially classification and regressor techniques. Models were used on the dataset of emails that comprises twenty-three features. The experimental outcome demonstrates that RF (Random Forest) & Adaboost outperform all other machine learning methods with an almost similar accuracy of 95%. KNN and ensemble approach achieved the highest 89.8% and 91% R2 scores. The comparison found that the ensemble approach outperforms state-of-arts machine learning methods regarding accuracy, error value, and R2 score.
更多查看译文
关键词
data mining (DM),customer retention,email marketing,machine learning,regressors
AI 理解论文
溯源树
样例
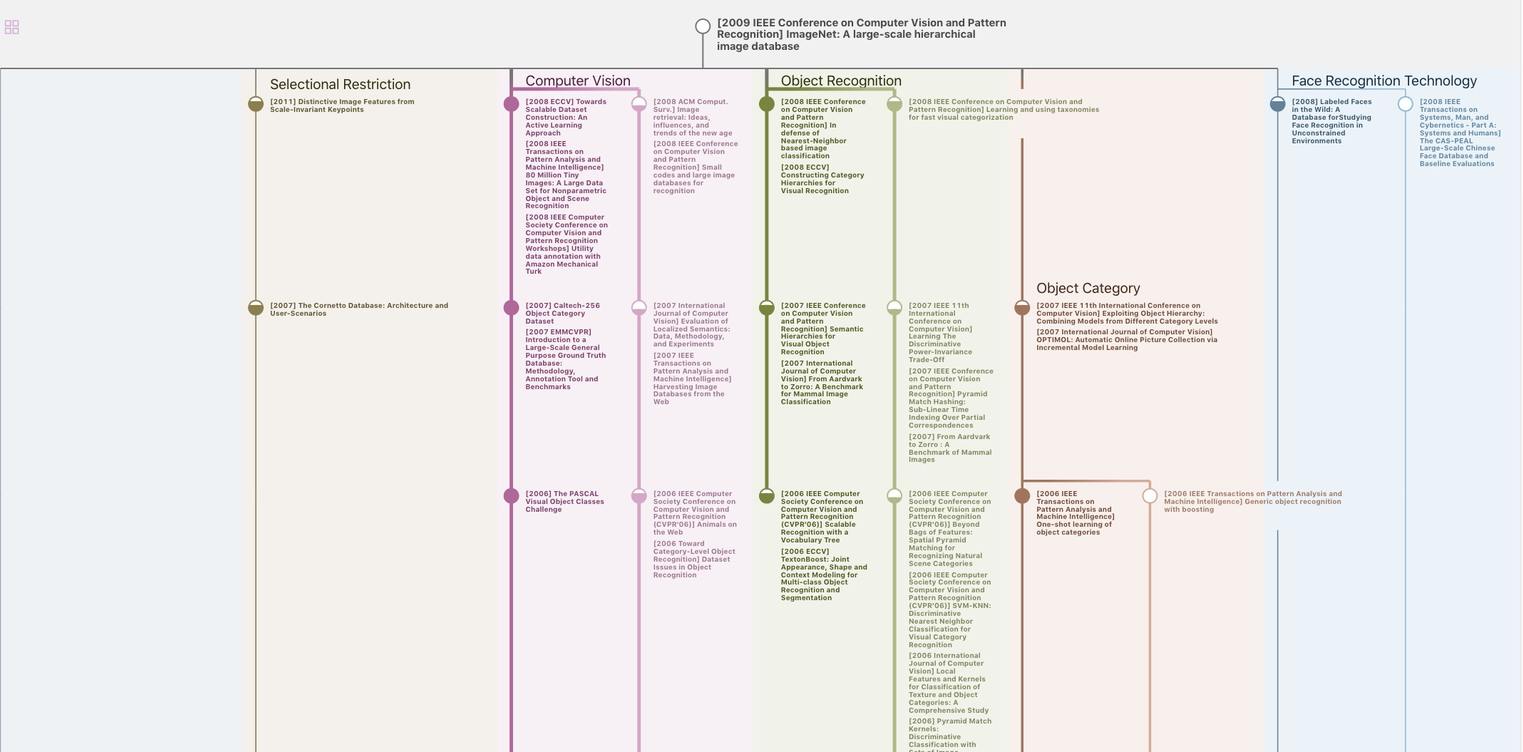
生成溯源树,研究论文发展脉络
Chat Paper
正在生成论文摘要