Intelligent System for the Diagnosis of Schizophrenia featuring Brain Textures from EEG
2023 3rd International Conference on Artificial Intelligence (ICAI)(2023)
摘要
Schizophrenia (ScZ) is a harmful disorder of the brain often associated with anxiety, depression and sociopsychological problems. An accurate and timely diagnosis of SZ proves helpful in the efficient cure of the disease. This research presents a novel pattern recognition framework for the accurate diagnosis of SZ using non-invasive electroencephalography (EEG). The raw dataset contains 19 channel EEGs collected from fourteen patients. Each EEG recording was segmented into 60-second segments to increase the number of observations and increase the diagnosis system performance. These segmented EEG observations were preprocessed by passing them through Fast-Independent component analysis (Fast-K'A), followed by band pass filter, and Empirical Mode Decomposition (EMD). EMD splits the input signal into Intrinsic mode functions (IMFs). After manual analysis, only the first two IMFs were added together to form a reconstructed preprocessed signal. Next, novel Brain Texture features were extracted from each channel of preprocessed EEG signal. Brain texture features from each channel were serially fused to form a final feature vector. These features were used to train and test a broad range of machine learning classification methods and the best performance was obtained via Fine k-Nearest Neighbors (FKNN). The proposed framework achieved 94.9% accuracy using 10-fold cross-validation outperforming the existing techniques.
更多查看译文
关键词
Empirical Mode Decomposition,k-nearest neighbors,EEG,Brain Textures,Schizophrenia
AI 理解论文
溯源树
样例
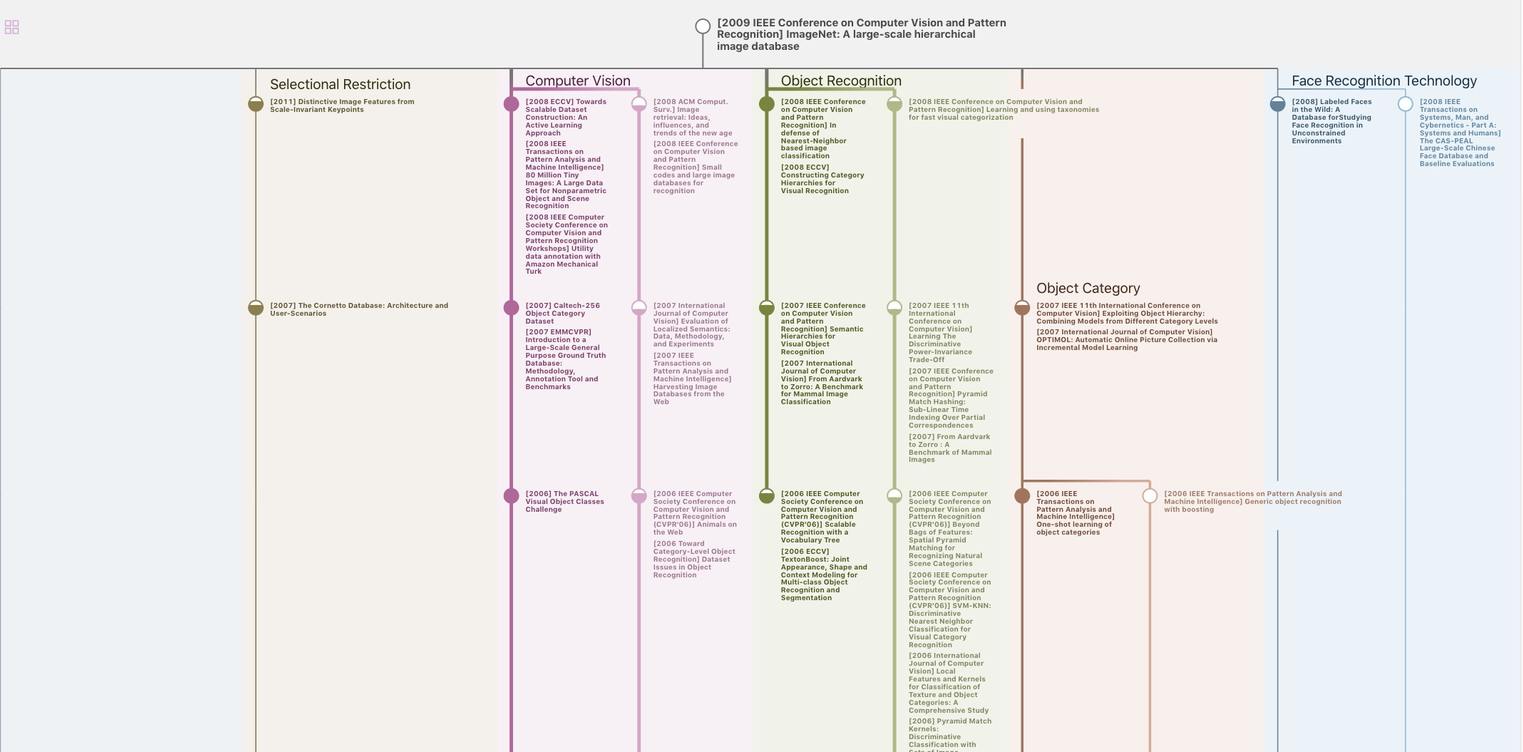
生成溯源树,研究论文发展脉络
Chat Paper
正在生成论文摘要