Pooling critical datasets with Federated Learning
2023 31st Euromicro International Conference on Parallel, Distributed and Network-Based Processing (PDP)(2023)
摘要
Federated Learning (FL) is becoming popular in different industrial sectors where data access is critical for security, privacy and the economic value of data itself. Unlike traditional machine learning, where all the data must be globally gathered for analysis, FL makes it possible to extract knowledge from data distributed across different organizations that can be coupled with different Machine Learning paradigms. In this work, we replicate, using Federated Learning, the analysis of a pooled dataset (with AdaBoost) that has been used to define the PRAISE score, which is today among the most accurate scores to evaluate the risk of a second acute myocardial infarction. We show that thanks to the extended-OpenFL framework, which implements AdaBoost.F, we can train a federated PRAISE model that exhibits comparable accuracy and recall as the centralised model. We achieved F1 and F2 scores which are consistently comparable to the PRAISE score study of a 16-parties federation but within an order of magnitude less time.
更多查看译文
关键词
Federated Learning,Machine Learning,Cardiology,Healthcare,Performance Analysis,Decentralized machine learning,Distributed machine learning,PRAISE score
AI 理解论文
溯源树
样例
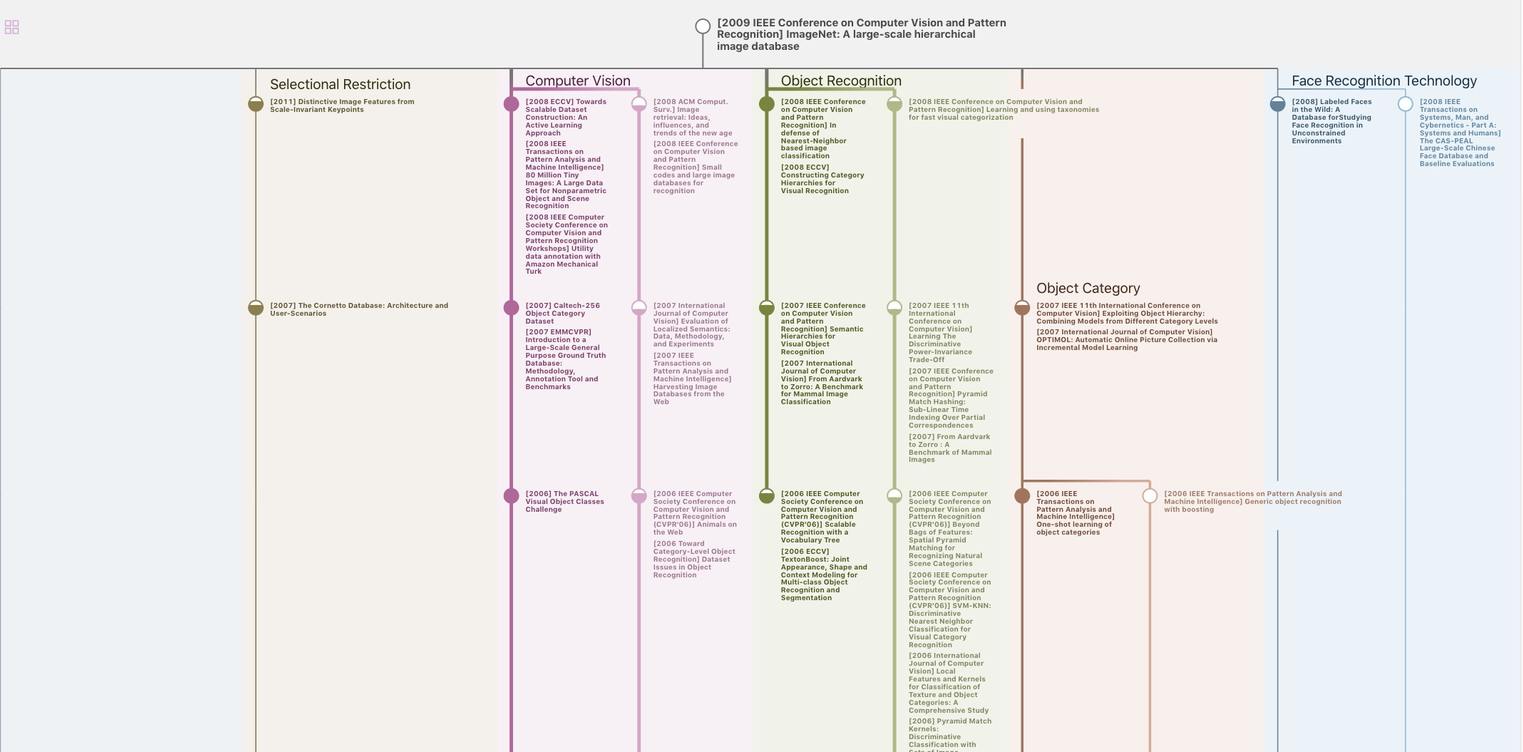
生成溯源树,研究论文发展脉络
Chat Paper
正在生成论文摘要