Multiobject Tracking via Discriminative Embeddings for the Internet of Things
IEEE Internet of Things Journal(2023)
摘要
Multiobject tracking (MOT) technology can be deployed to the Internet of Things (IoT) devices to enhance the security and reliability of some video analysis applications, such as video surveillance and intelligent security system. However, since the IoT devices with limited computing capacity and storage, most existing MOT methods are difficult to deploy to IoT devices and exhibit poor tracking robustness in scenes with frequent occlusions, severe crowded, and scale variations. To alleviate the aforementioned issues, we propose a regression-based online MOT method. First, an object-aware embedding extraction module (OAEM) is designed to extract preliminary discriminative embedding of the object in the current frame. Then, an embedding aggregation module (EAM) is proposed to obtain high-quality aggregated embedding in temporal. Finally, the temporal embedding combined with the preliminary embedding extracted from the current frame to obtain a refined embedding feature for subsequent position prediction and association. Importantly, we achieve a beneficial interaction between embedding extraction, position prediction and association task. The proposed method does not suffer from significant memory consumption. Therefore, our method is a potential solution for intelligent video analysis on IoT devices. To evaluate the proposed method, we have conducted numerous experiments on the MOT16, MOT17, and MOT20 benchmark data sets. Results demonstrate that the proposed method can provide a more robust tracking performance compared to other optimal methods.
更多查看译文
关键词
Feature extraction, Internet of Things, Task analysis, Robustness, Data mining, Trajectory, Predictive models, Embedding extraction, Internet of Things (IoT), joint optimization, multiobject tracking (MOT), position prediction
AI 理解论文
溯源树
样例
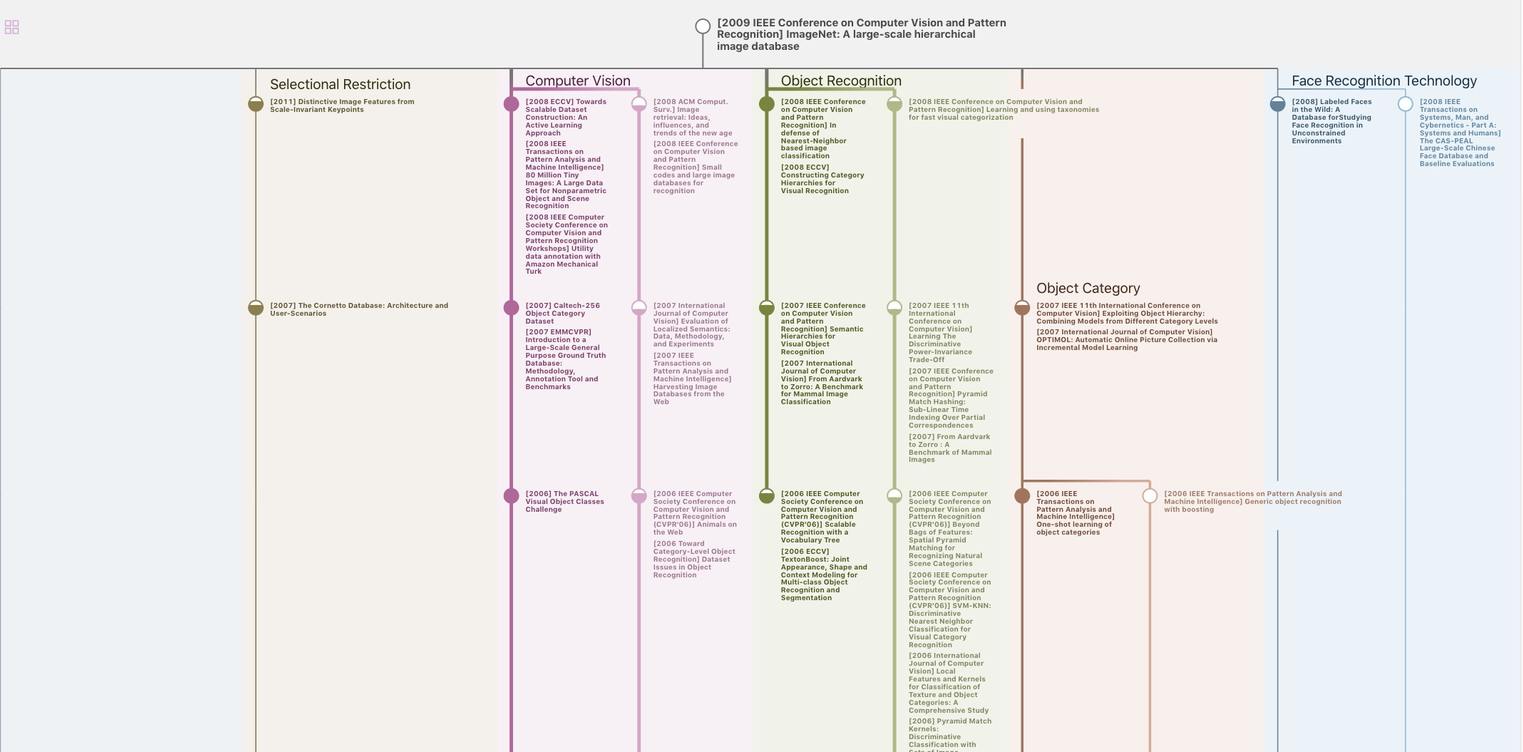
生成溯源树,研究论文发展脉络
Chat Paper
正在生成论文摘要