Learning from Multiple Datasets for Recognizing Human Actions.
ICVGIP(2022)
摘要
Action recognition has evolved as an important research problem in the computer vision community. Majority of the human action recognition methods focus mainly on training from a single dataset. Scarcity of labelled data in a single dataset often leads to over-fitting for recognizing each and every action. So, use of multiple datasets is expected to alleviate this situation. However, in the real world, labelled data is frequently obtained from a variety of origins which can exhibit substantial variations. In this paper, we demonstrate how human actions can be accurately recognized by using multiple datasets based on the concept of unsupervised domain adaptation. We propose a novel approach termed as Multi-Dataset Human Action Recognition (MDHAR) for recognizing human actions using two or more publicly available benchmark datasets. Common and heterogeneous features for a dataset pair are aligned. Distributions across a dataset pair are also balanced to reduce class imbalance. We further show that our unsupervised domain adaptation approach can be extended to more than two datasets. Extensive experimentation on three publicly available benchmark datasets clearly reveal the efficacy of the proposed solution for cross dataset human action recognition from multiple datasets.
更多查看译文
AI 理解论文
溯源树
样例
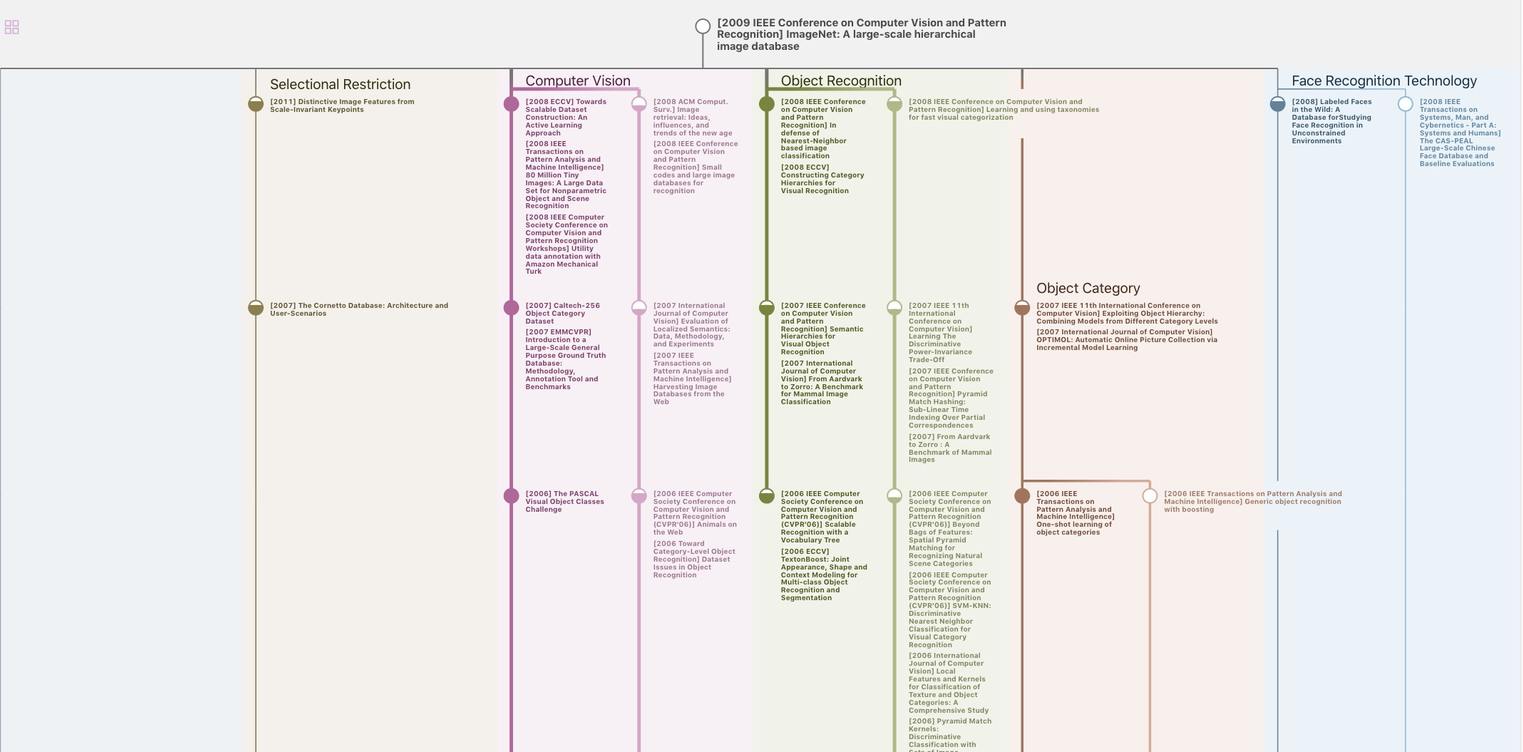
生成溯源树,研究论文发展脉络
Chat Paper
正在生成论文摘要