A Novel Chinese Resume Named Entity Recognition Model Based on Lexical Enhancement.
ICCPR(2022)
摘要
The resume's popularity on the Internet has greatly increased with the development of the communication form. It is a concern of researchers to analyze the resumes of job applicants using the Named Entity Recognition (NER) method. The difficulty of Chinese Resume NER rests with word segmentation ambiguity and domain knowledge complexity. To tackle the issue, a novel lexical enhancement Long Short-Term Memory (LSTM) model with the average encoding strategy (LEAE-LSTM) is proposed. First, through the pre-trained models, the representations of characters and words are encoded separately. The lexical features with complementary information are introduced for the character sequence by matching the lexicon. Furthermore, to improve contextual awareness, the multi-metadata embeddings are combined as the input of the LSTM layer. The sentence's implicit correlations are picked up by the self-attention mechanism. Experiments on the benchmark resume dataset demonstrate that LEAE-LSTM surpasses other state-of-the-art methods. For the Chinese resume dataset, LEAE-LSTM gains a 1.8% improvement in F1 score over the baseline model Lattice LSTM.
更多查看译文
AI 理解论文
溯源树
样例
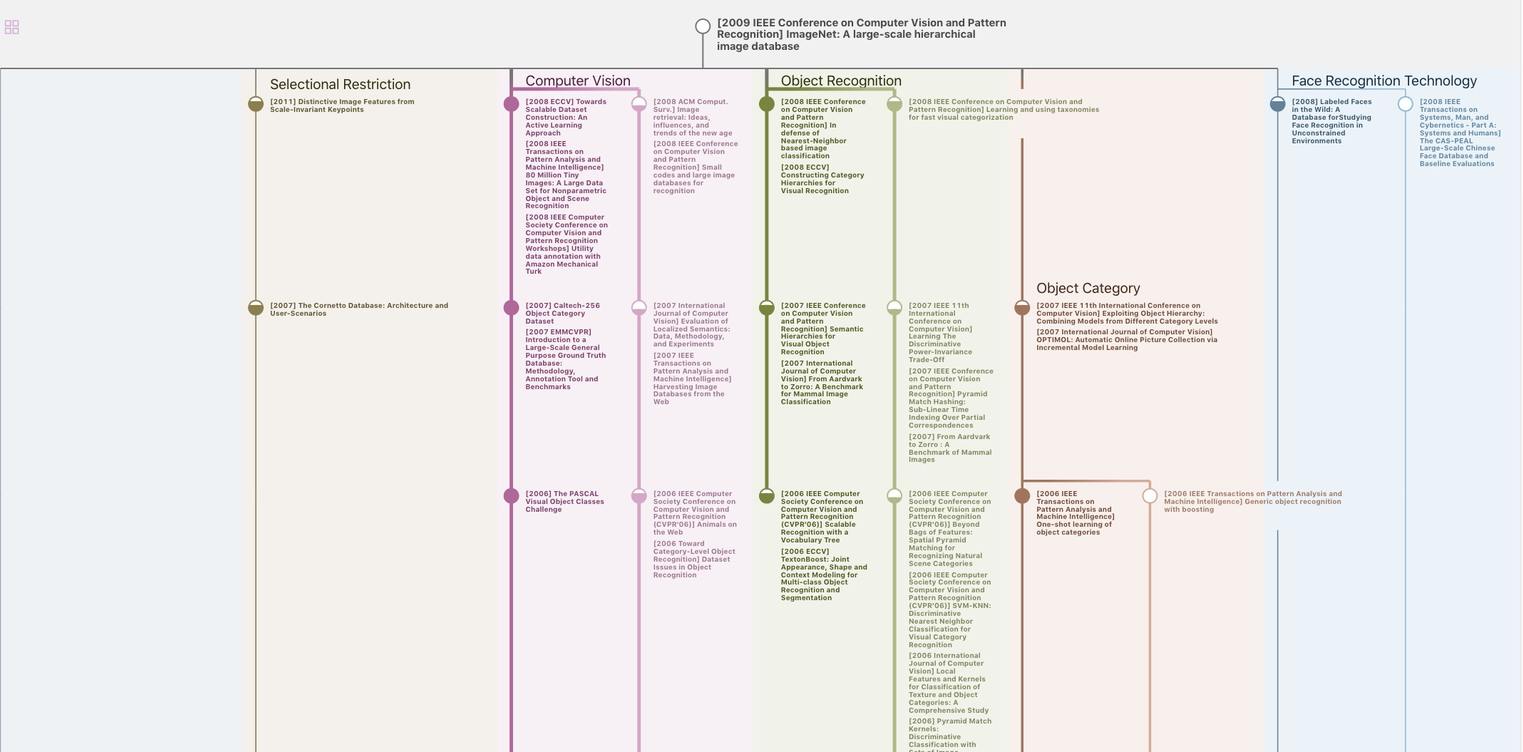
生成溯源树,研究论文发展脉络
Chat Paper
正在生成论文摘要