Deep Reinforcement Learning Based Ontology Meta-Matching Technique.
IEICE Trans. Inf. Syst.(2023)
摘要
Ontologies are regarded as the solution to data hetero-geneity on the Semantic Web (SW), but they also suffer from the het-erogeneity problem, which leads to the ambiguity of data information. Ontology Meta-Matching technique (OMM) is able to solve the ontology heterogeneity problem through aggregating various similarity measures to find the heterogeneous entities. Inspired by the success of Reinforcement Learning (RL) in solving complex optimization problems, this work pro-poses a RL-based OMM technique to address the ontology heterogeneity problem. First, we propose a novel RL-based OMM framework, and then, a neural network that is called evaluated network is proposed to replace the Q table when we choose the next action of the agent, which is able to reduce memory consumption and computing time. After that, to bet-ter guide the training of neural network and improve the accuracy of RL agent, we establish a memory bank to mine depth information during the evaluated network's training procedure, and we use another neural network that is called target network to save the historical parameters. The experi-ment uses the famous benchmark in ontology matching domain to test our approach's performance, and the comparisons among Deep Reinforcement Learning(DRL), RL and state-of-the-art ontology matching systems show that our approach is able to effectively determine high-quality alignments.
更多查看译文
关键词
reinforcement learning,meta-matching
AI 理解论文
溯源树
样例
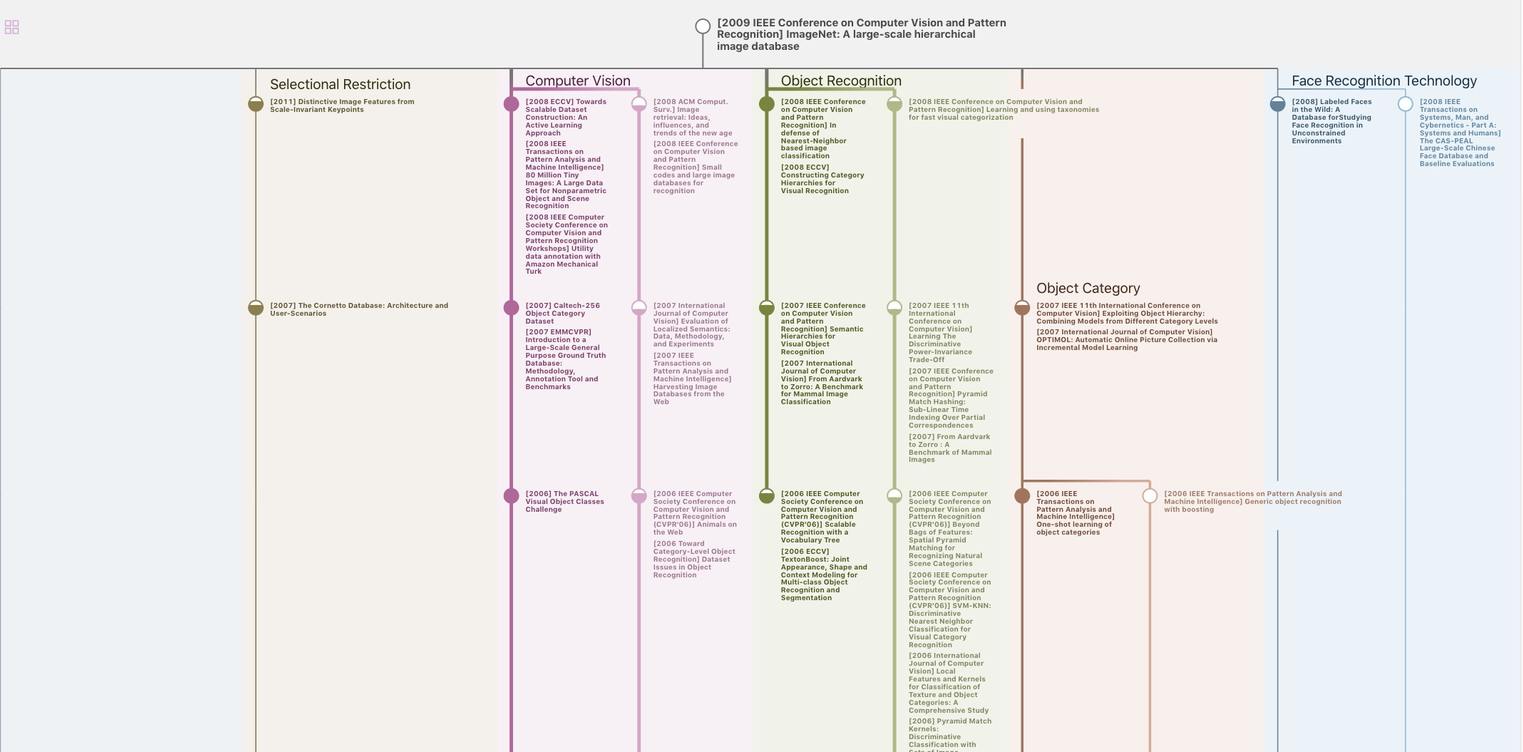
生成溯源树,研究论文发展脉络
Chat Paper
正在生成论文摘要