Indoor Point Cloud Segmentation Using a Modified Region Growing Algorithm and Accurate Normal Estimation.
IEEE Access(2023)
摘要
With the development of 3D sensors, 3D point cloud data can now be obtained conveniently. Therefore, it is crucial to process point cloud data automatically. Region growing is a commonly used algorithm to segment point clouds, which greatly depends on the accuracy of points' normals and requires tuning two thresholds; i.e., the increment of curvature (sigma th) and normal angles (theta th). In this paper, we improve the region growing algorithm in two ways: Accurate normal estimation and strengthening the region growing criteria. For the first aspect, principal component analysis (PCA) is utilized to estimate the initial normals of the point cloud. Then, the points are divided into regular points (RP) and sharp feature points (SFP), according to their initial normals. A robust estimator-based PCA is then applied to refine the SFP normals. For the latter aspect, non-connected and non-coplanar points are detected and ignored when region grows. Finally, the segmentation performance of the proposed method is evaluated using internal and external indices. The results indicate that the proposed method can accurately estimate the point normals within an acceptable time, and obtain a better result than the classic PCA-based region growing algorithm and advanced DetMM-based methods.
更多查看译文
关键词
Normal estimation, region growing, PCA, robust estimator, point cloud segmentation
AI 理解论文
溯源树
样例
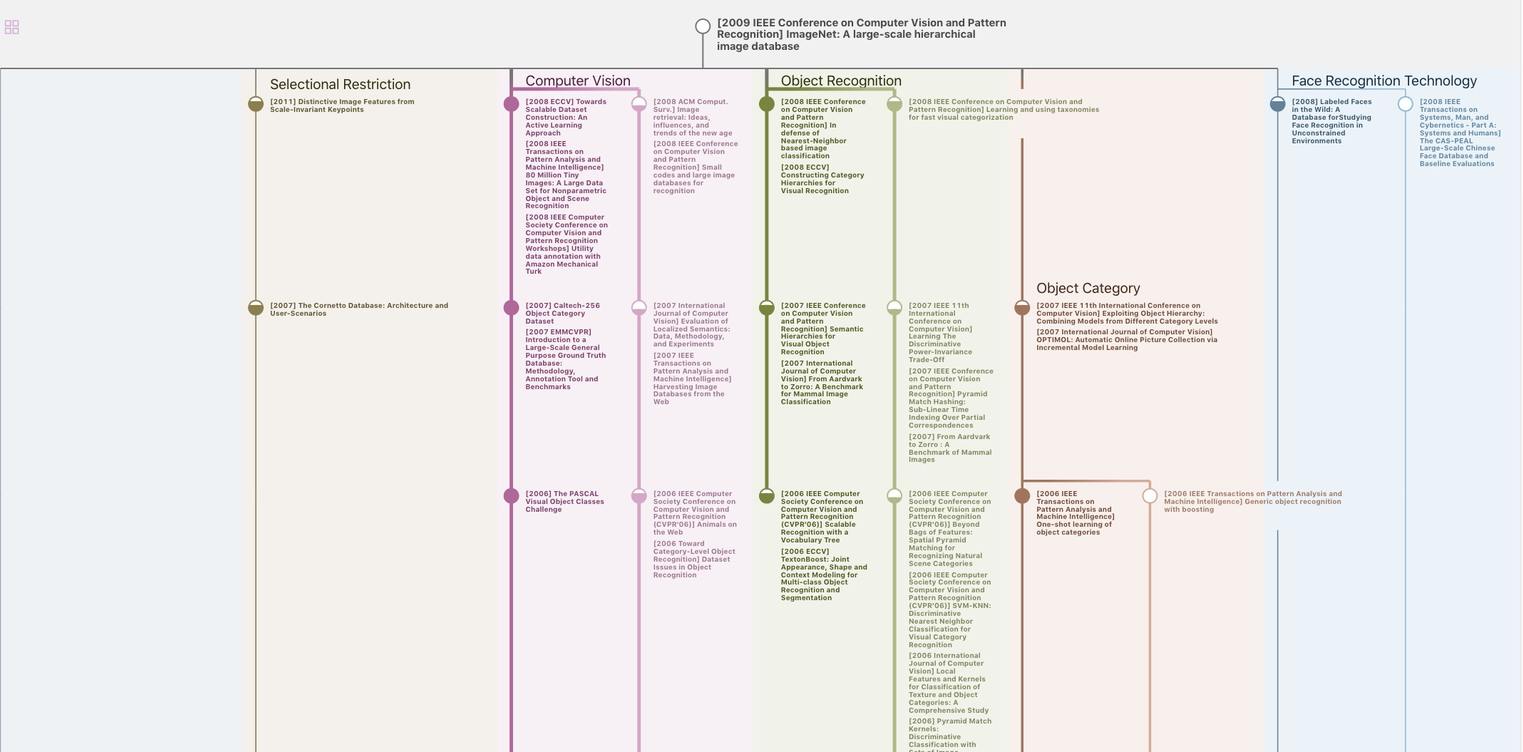
生成溯源树,研究论文发展脉络
Chat Paper
正在生成论文摘要