Multimodule Deep Learning Scheme for Elastic Wave Inversion of Inhomogeneous Objects With High Contrasts.
IEEE Trans. Geosci. Remote. Sens.(2023)
摘要
In elastic wave inverse scattering problems, the material-property reconstruction, e.g., distributions of mass density, compressional wave speed, and shear wave speed from a limited set of measurement data, has attracted considerable research interest. However, simultaneous inversion of multiple parameters endures high computation costs, and reconstructed compressional and shear speeds may become unrealistic if no physical constraint is imposed. Meanwhile, because large objects with high contrasts over the background medium tend to induce high nonlinearity during the inversion process, it is difficult to obtain high-quality high-contrast material properties. To overcome such difficulties, we have developed a multimodule deep learning scheme (MMDLS) with physical constraints for multiparameter elastic wave inversion of high-contrast objects in inhomogeneous media. This scheme consists of: 1) a preliminary imaging module (PIM), in which a deep residual network (ResNet) is employed to convert the scattered field data into the preliminary inversion images; 2) an image-enhancement module (IEM), in which a U-Net is employed to further enhance the image quality; and 3) a convolutional neural network (CNN) that is employed as the physical constraint module (PCM) to allow elastic wave parameters to satisfy the actual physical constraint. Numerical examples have demonstrated that the proposed scheme not only accurately achieves multiparameter elastic wave inversion but also has good generalizability. Finally, the scheme can be applied to complex objects with high contrasts in both noise-free and noisy environments.
更多查看译文
关键词
Elastic wave inversion,high contrast,machine learning,multiparameter
AI 理解论文
溯源树
样例
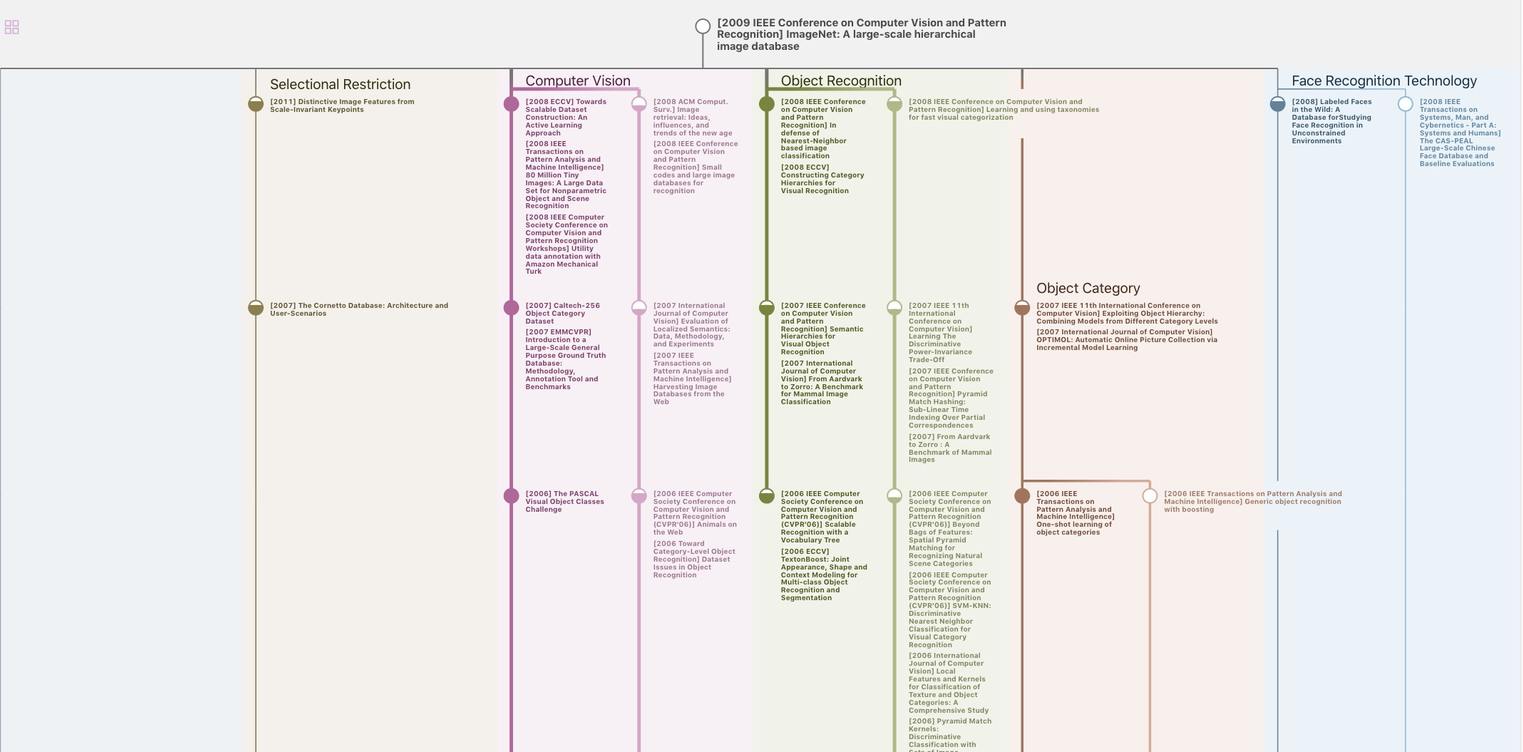
生成溯源树,研究论文发展脉络
Chat Paper
正在生成论文摘要