VP-Net: Voxels as Points for 3-D Object Detection.
IEEE Trans. Geosci. Remote. Sens.(2023)
摘要
The 3-D object detection with light detection and ranging (LiDAR) point clouds is a challenging problem, which requires 3-D scene understanding, yet this task is critical to autonomous driving. Existing voxel-based 3-D object detectors are becoming increasingly popular but have several shortcomings. For example, during voxelization, features of distant sparse point clouds are largely discarded, which leads to the missing detection of objects. In addition, the correlation of points between voxels and the importance of different voxels within a region are not well learned. Therefore, we present a robust network [voxel-as-point network (VP-Net)] that views voxels as points to accurately detect 3-D objects in LiDAR point clouds and can capture objects' internal relationships. The 3-D CNN processing shows the output features of VP-Net as key points. The relationship between key points is then constructed into local graphs to enhance object feature extraction via a self-attention mechanism. Finally, the Euclidean distance between the extracted features guides our model's weight reassignment for strengthening the importance of neighbor points, thereby enhancing the internal feature aggregation of objects. Experiments on KITTI and nuScenes 3-D object detection benchmarks demonstrate the efficiency of enhancing intervoxel validity within object features and show that the proposed VP-Net can achieve the state-of-the-art performance.
更多查看译文
关键词
voxels,detection,object,points,vp-net
AI 理解论文
溯源树
样例
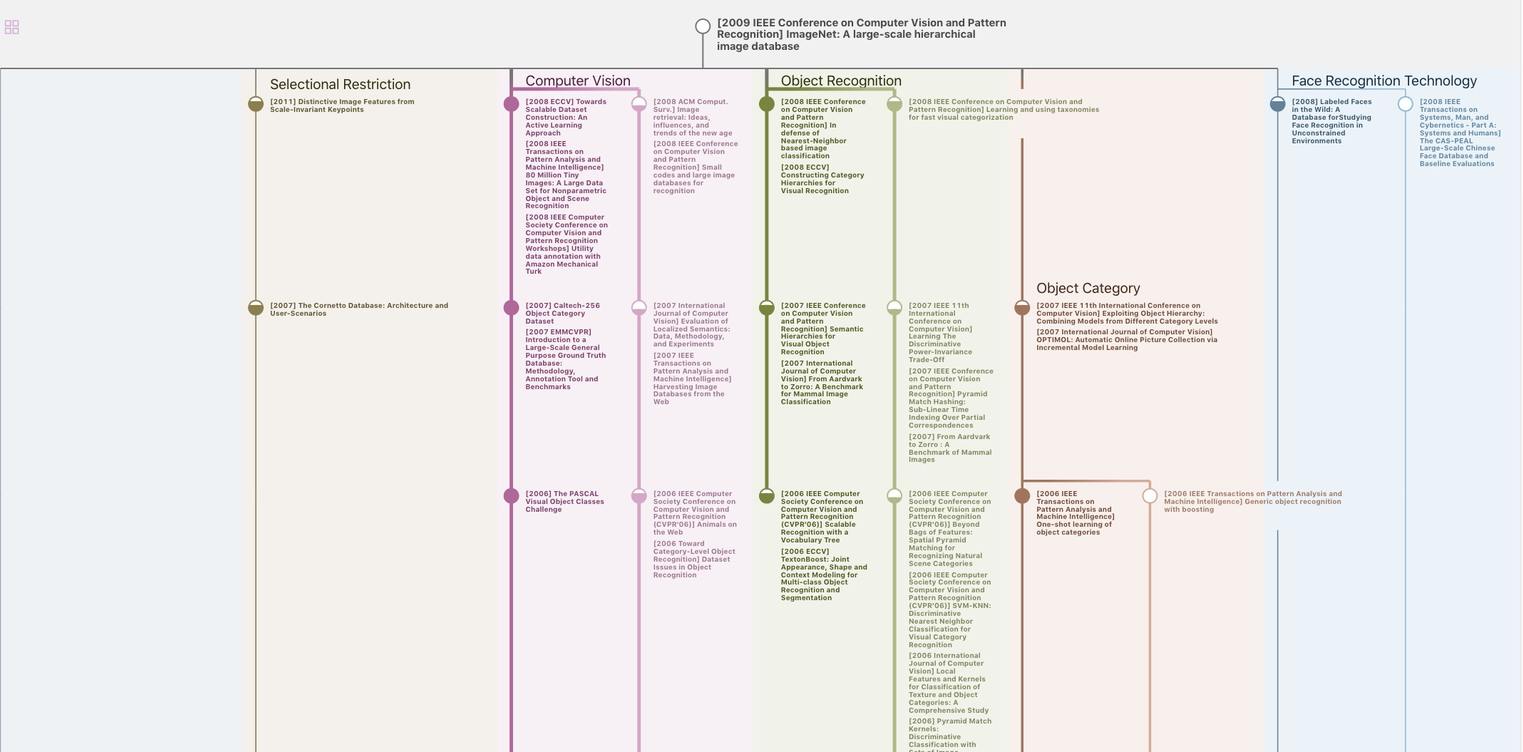
生成溯源树,研究论文发展脉络
Chat Paper
正在生成论文摘要