Long-Tailed Time Series Classification via Feature Space Rebalancing.
DASFAA (1)(2023)
摘要
Learning unbiased decision boundaries is crucial for time series classification. Real-world datasets typically exhibit long-tailed natures of class distributions, which results in an imbalanced feature space after training, i.e., decision boundaries will be easily biased towards dominant classes that dominate the feature space. However, existing methods mostly train models from artificially balanced datasets, making it still unclear how to deal with the long-tailed natures of time series data in real-world scenarios. Motivated by this question, we analyze the similarities and differences between long-tailed time series classification and general long-tailed recognition, and propose a Feature Space Rebalancing (FSR) strategy for time series classification, which works jointly from both representation and data perspectives. Specifically, from the representation perspective, we design Balanced Contrastive Learning (BCL), which avoids excessive intra-class compaction of tail classes by introducing a balanced supervised contrastive loss with hierarchical prototypes, resulting in a balanced feature space and better generalization. From the data perspective, we explore the effectiveness of traditional data augmentation on long-tailed distributions and propose an Adaptive Temporal Augmentation (ATA) to rebalance the potential feature space at the temporal level. Extensive experiments on multiple long-tailed time series datasets demonstrate its superiority, including different class distributions and imbalance ratios.
更多查看译文
关键词
time series classification,feature space rebalancing,time series,long-tailed
AI 理解论文
溯源树
样例
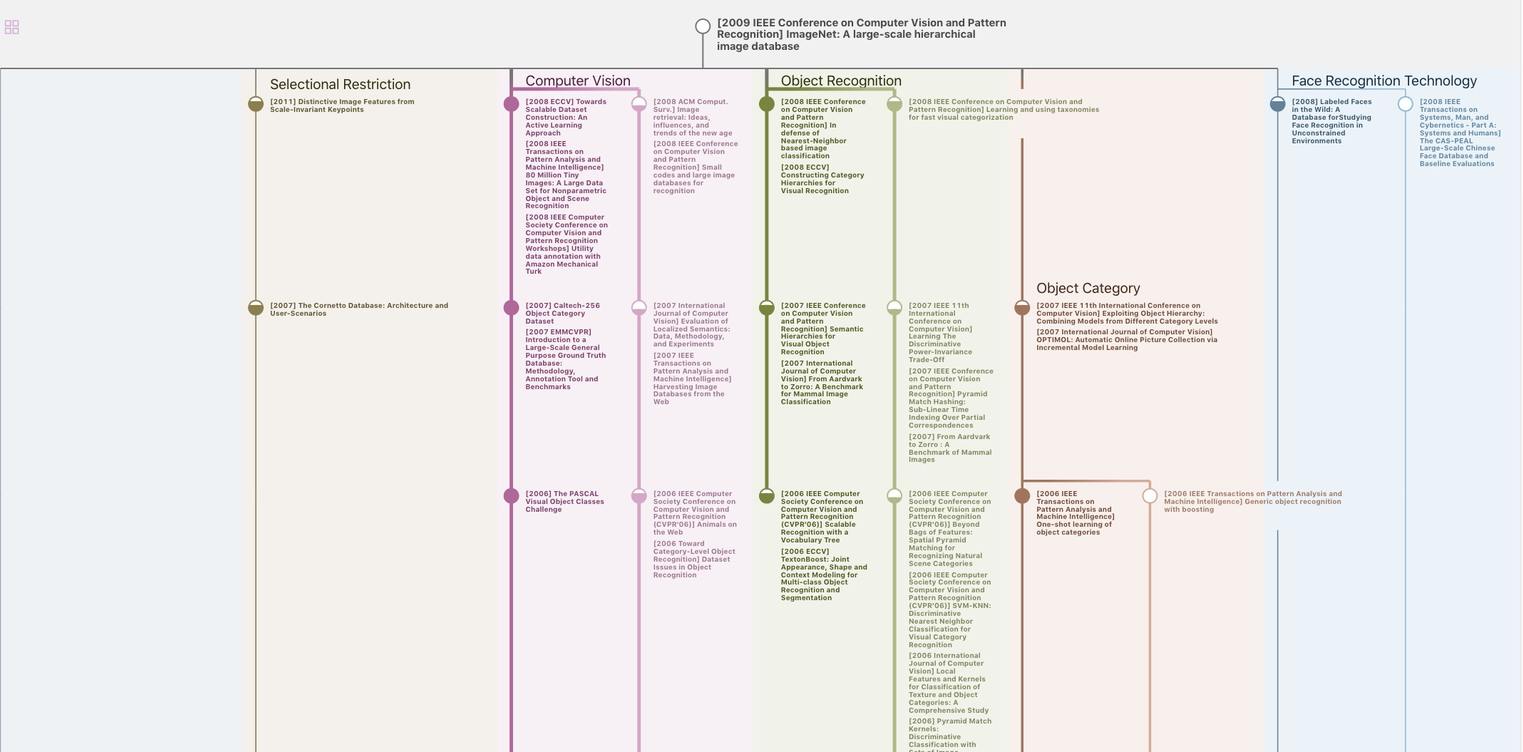
生成溯源树,研究论文发展脉络
Chat Paper
正在生成论文摘要