Deep Labeling of fMRI Brain Networks.
CoRR(2023)
摘要
Resting State Networks (RSNs) of the brain extracted from Resting State functional Magnetic Resonance Imaging (RS-fMRI) are used in the pre-surgical planning to guide the neurosurgeon. This is difficult, though, as expert knowledge is required to label each of the RSNs. There is a lack of efficient and standardized methods to be used in clinical workflows. Additionally, these methods need to be generalizable since the method needs to work well regardless of the acquisition technique. We propose an accurate, fast, and lightweight deep learning approach to label RSNs. Group Independent Component Analysis (ICA) was used to extract large scale functional connectivity patterns in the cohort and dual regression was used to back project them on individual subject RSNs. We compare a Multi-Layer Perceptron (MLP) based method with 2D and 3D Convolutional Neural Networks (CNNs) and find that the MLP is faster and more accurate. The MLP method performs as good or better than other works despite its compact size. We prove the generalizability of our method by showing that the MLP performs at 100% accuracy in the holdout dataset and 98.3% accuracy in three other sites' fMRI acquisitions.
更多查看译文
关键词
brain,networks
AI 理解论文
溯源树
样例
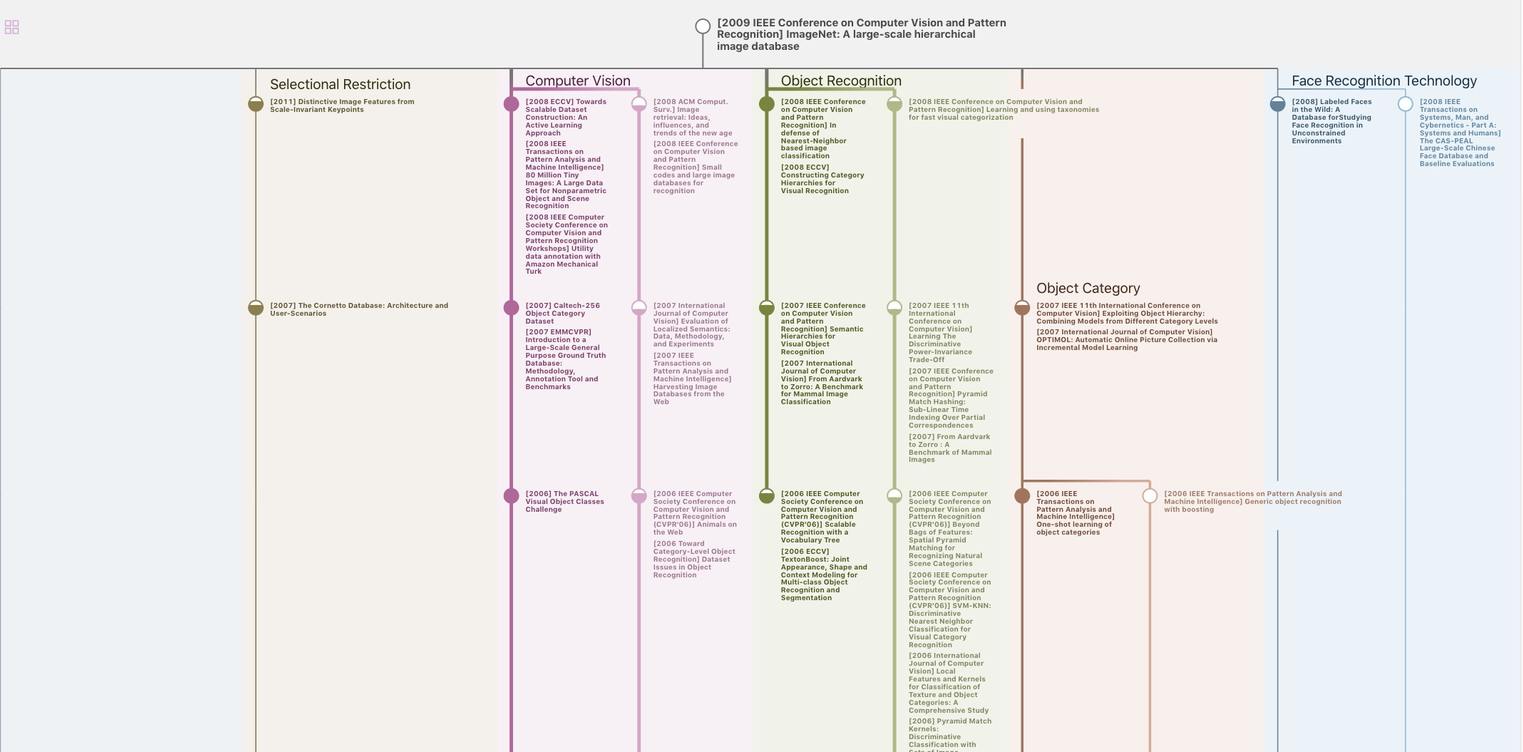
生成溯源树,研究论文发展脉络
Chat Paper
正在生成论文摘要