A novel extended multimodal AI framework towards vulnerability detection in smart contracts
Inf. Sci.(2023)
摘要
Current automatic data-driven vulnerability detection in smart contracts selects and processes features of interest under black box settings without empirical justification. In this paper, we propose a smart contract testing methodology that bestows developers with flexible, practical and customizable strategies to detect vulnerabilities. Our work enforces strong whitebox knowledge to a series of supervised multimodal tasks under static analysis. Each task encapsulates a vulnerability detection branch test and pipelines feature selection, dimension unification, feature fusion, model training and decision-making. We exploit multiple features made up of code and graph embeddings at the single modality level (intramodal settings) and across individual modalities (intermodal settings). We assign each task to either intramodal or intermodal settings, and show how to train state-of-the-art self-attentive bi-LSTM, textCNN, and random forest (RF) models to extract a joint multimodal feature representation per task. We evaluate our framework over 101,082 functions extracted from the SmartEmbed dataset, and rank each multimodal vulnerability mining strategy in terms of detection performance. Extensive experiments show that our work outperforms existing schemes, and the highest performance reaches 99.71%.
更多查看译文
关键词
Smart contract,Vulnerability detection,Multimodal,AI approach,White box
AI 理解论文
溯源树
样例
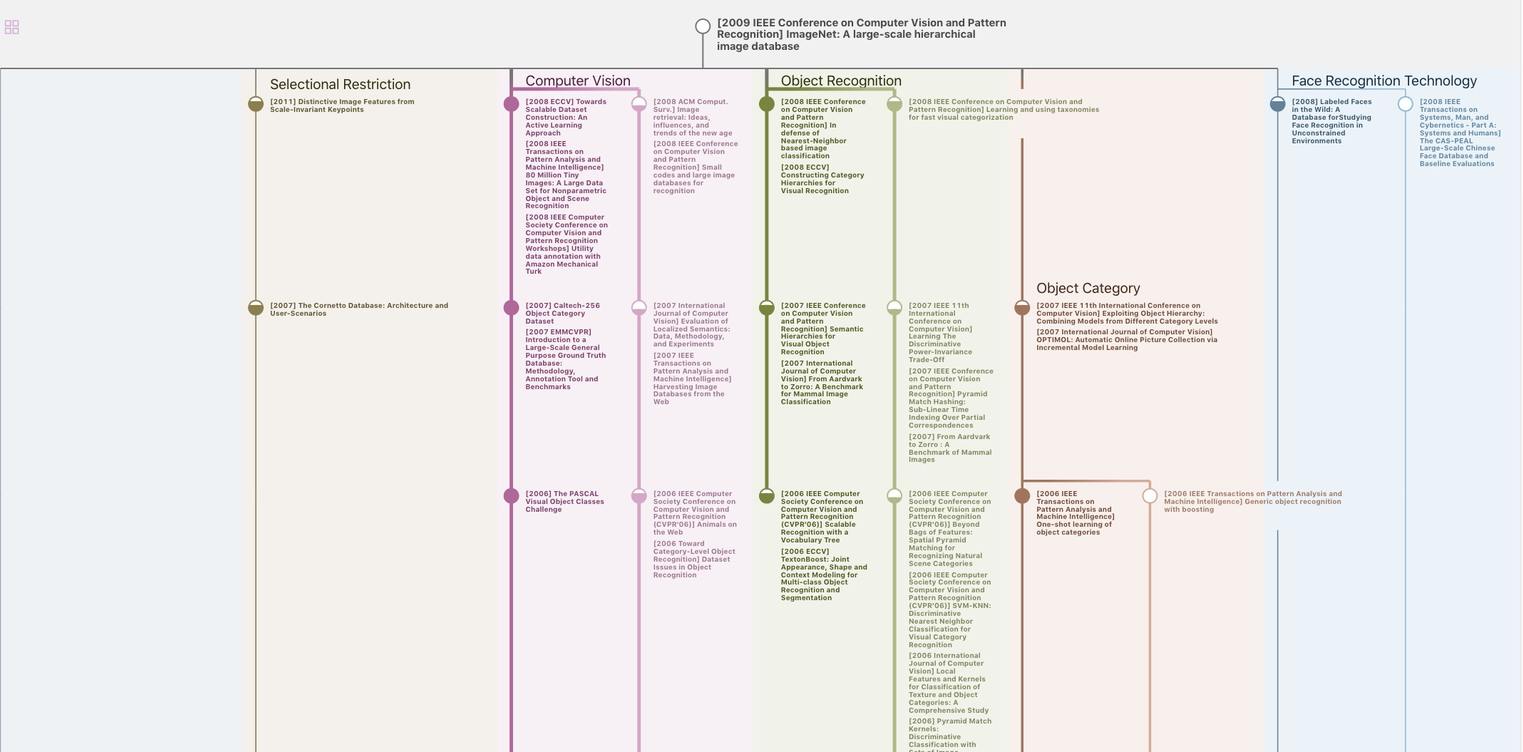
生成溯源树,研究论文发展脉络
Chat Paper
正在生成论文摘要