ML-based Traffic Steering for Heterogeneous Ultra-dense beyond-5G Networks.
WCNC(2023)
摘要
As networks become denser and more heterogeneous different paths can be considered in order to reach each multihomed UE, offering optimal performance. 5G and beyond networks feature contributions related to the dynamic programming of the network, from the operator side, in order to optimally allocate resources in the network. In this work, we consider such a case, where network access is provided to the end-users via heterogeneous (3GPP and non-3GPP) Distributed Units (DUs), converging to a single Central Unit (CU), and programmable on the fly with external interfaces. We employ Machine Learning (ML) methods in order to forecast the Quality of Service (QoS) that a wireless client will get from the network in the near future based on the Channel State Information (CSI) metric. Subsequently, we appropriately steer the traffic over the different heterogeneous DUs for ensuring that the network meets the needs of the UEs. We design, develop, deploy and evaluate our method in a real testbed environment, using emulated mobility. Our results show that the overall throughput of each UE can be drastically improved compared to existing allocation mechanisms.
更多查看译文
关键词
5G, Artificial Intelligence, Disaggregated RAN, HetNets, Neural Networks, Traffic Steering
AI 理解论文
溯源树
样例
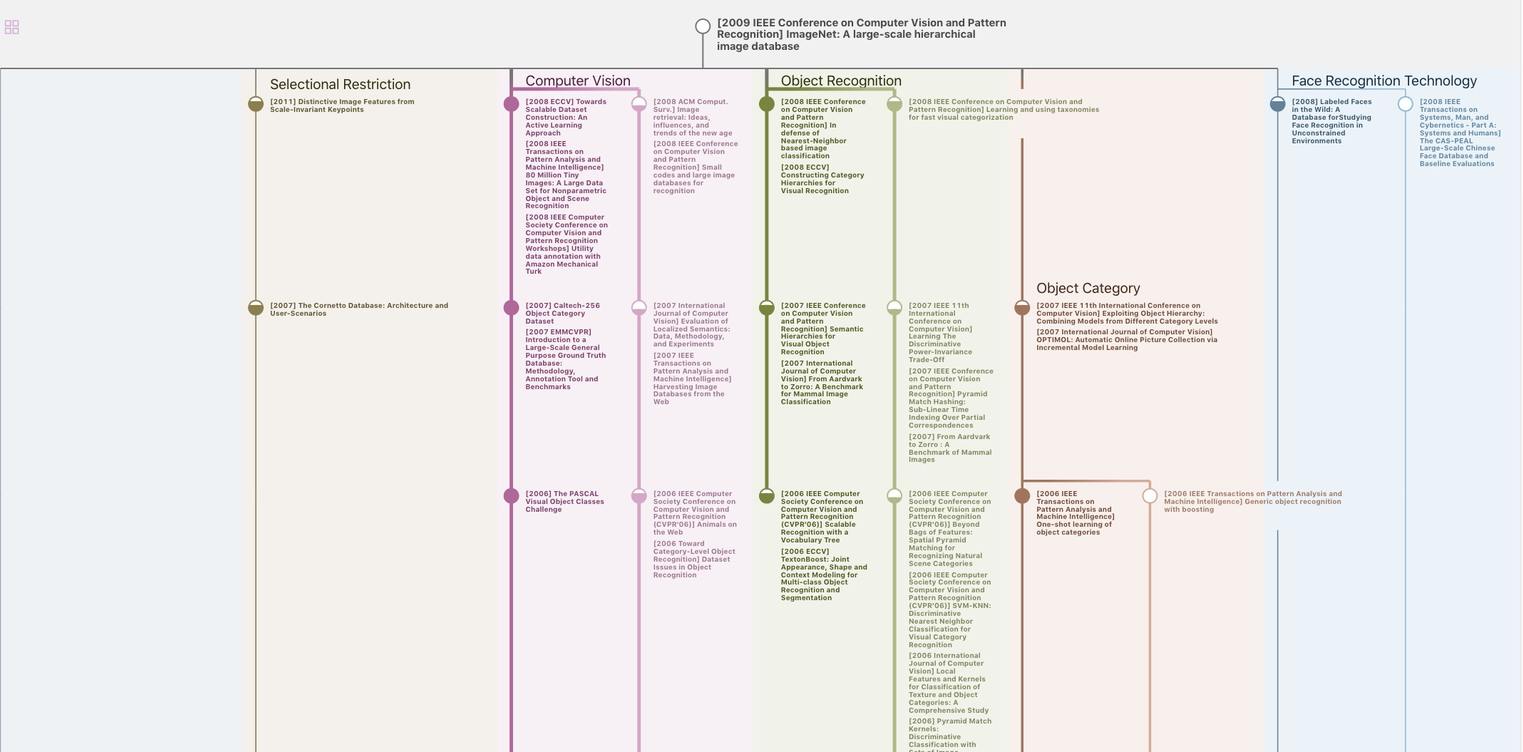
生成溯源树,研究论文发展脉络
Chat Paper
正在生成论文摘要