Fusion representation learning for foreground moving object detection.
Digit. Signal Process.(2023)
摘要
This paper investigates the problem of effective and robust fusion representation for foreground moving object detection. Many deep learning-based approaches pay attention to network architecture based on a single modality image, ignoring the complementary mechanism of cross-modal images or only considering single-frame prediction without temporal association. We tackle these problems by proposing a fusion representation learning method for the foreground moving object detection task, which consists of two major modules: the upstream fusion representation module (FRM) and the downstream foreground moving object detection module (FODM). Unlike traditional feature aggregate methods, the FRM module is a quality-aware and online learnable fusion module which can aggregate valuable features while rejecting the harmful information in the source images. Specifically, the FODM module is a siamese convolutional neural network to detect foreground moving objects by aggregating the time-sequence images generated by FRM. Moreover, a new aligned foreground moving object detection dataset of infrared and visible images is constructed to provide a new option for benchmark evaluation. Experimental results and comparisons with the state-of-the-art on three public datasets validate the effectiveness, robustness, and overall superiority of our method.
更多查看译文
关键词
object detection,fusion representation,foreground
AI 理解论文
溯源树
样例
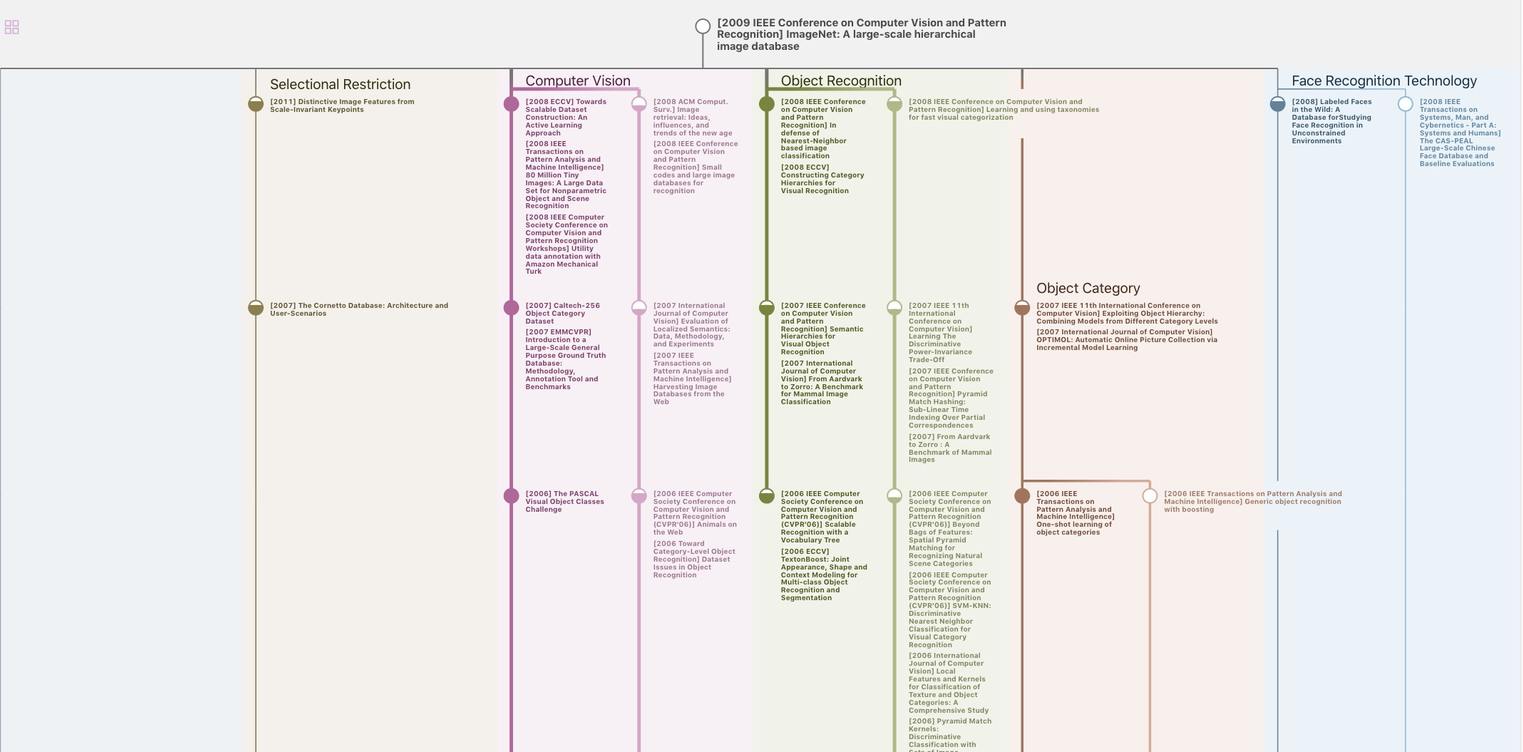
生成溯源树,研究论文发展脉络
Chat Paper
正在生成论文摘要