Eco-friendly weeding through precise detection of growing points via efficient multi-branch convolutional neural networks.
Comput. Electron. Agric.(2023)
摘要
Weeds can give negative effects for plant growth, and effectively controlling them is a significant challenge. Traditional methods, such as herbicides, may not be environmentally friendly, and manual weeding can be costly. The laser-based weeding is a potentially alternative way of weeding in an environmentally friendly way. Growing point detection and their pin-point striking would be more desirable in the laser-based weeding technology. In this study, we propose an encoder-decoder-based convolutional neural network with a dual decoder branch to detect the growing point of weeds. The decoder incorporates spatial and channel attention, as well as a novel activation gate mechanism to control the attention. We also present a simple yet effective strategy for combining the outputs of the decoder branches. The proposed method was tested on a real field dataset containing various growing stages of weeds and was compared to state-of-the-art methods using point -based metrics. The results show that the proposed method outperforms existing approaches, with a detection rate of 0.8505, precision of 0.8641, miss rate of 0.1391, RMSE of 22.68, and MAE of 17.95. The implementation code can be accessed at https://github.com/dewamsa/WGPdetection.git.
更多查看译文
关键词
Convolutional neural network, Growing point, Semantic graphics, Precision weeding
AI 理解论文
溯源树
样例
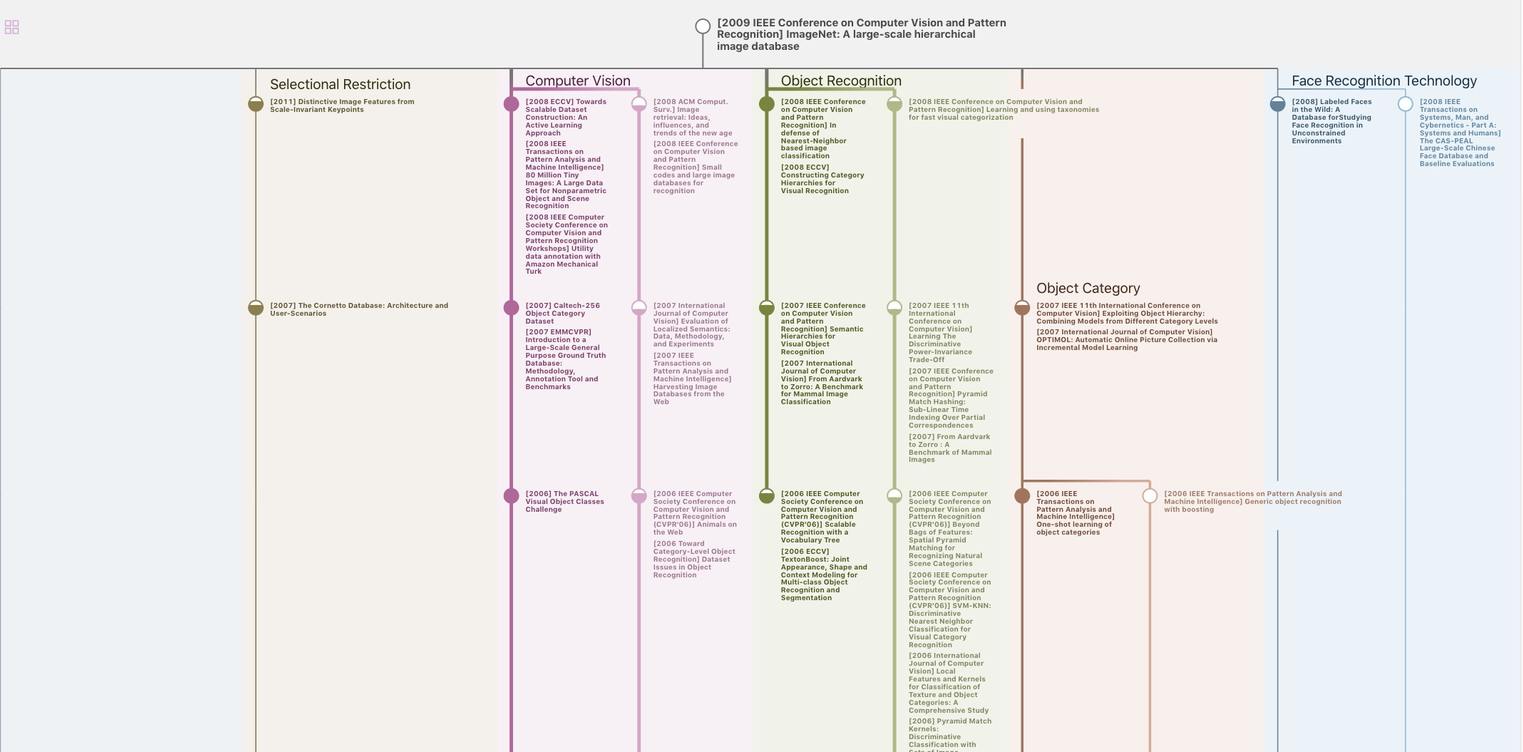
生成溯源树,研究论文发展脉络
Chat Paper
正在生成论文摘要