Induction motor short circuit diagnosis and interpretation under voltage unbalance and load variation conditions.
Expert Syst. Appl.(2023)
摘要
Predictions from machine learning algorithms have often supported decision-making in industrial processes. Despite this, complex models can be challenging to interpret, sometimes shrouding the entire prediction process in an undesirable mystery. Understanding how the classifiers’ recommendations are made helps human experts understand the phenomenon and develop better data-driven solutions. Therefore, this study takes advantage of Shapley additive explanations to explain the predictions obtained by the classifier and select the most appropriate features for the approaches. The experiments use extreme gradient boosting to evaluate temporal, spectral, and wavelet features of three-phase induction motor current signals. The proposed approach effectively reduces the number of attributes without losing performance, provides an understanding of how each feature affects the model over a wide range of voltage unbalances and torque values, and detects early inter-turn short circuits with severity of 1%. The results show that combining the intelligent model with Shapley explanations improves stator winding fault diagnosis in these highly problematic situations.
更多查看译文
关键词
Explainable artificial intelligence,Machine learning,Fault diagnosis,Induction machines,Inter-turn short circuit,Shapley additive explanations
AI 理解论文
溯源树
样例
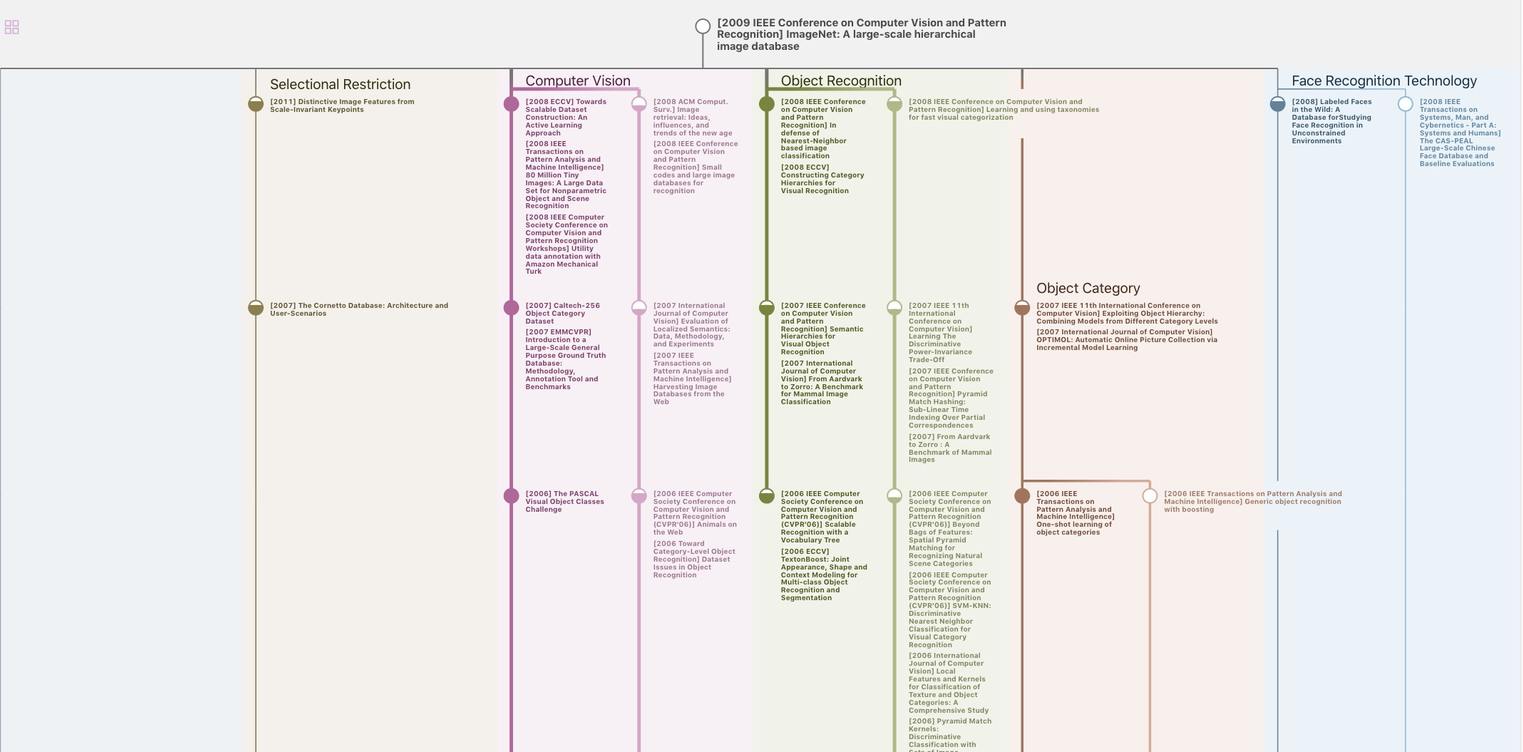
生成溯源树,研究论文发展脉络
Chat Paper
正在生成论文摘要