Deep Reinforcement Learning of UAV Tracking Control Under Wind Disturbances Environments
IEEE Trans. Instrum. Meas.(2023)
摘要
Aiming at the problems of strong nonlinearity, strong coupling, and unknown interference encountered in the flight control process of unmanned aerial vehicles (UAVs) in a complex dynamic environment and reinforcement-learning-based algorithm generalization, this study presents an innovative incremental reinforcement-learning-based algorithm for UAV tracking control in a dynamic environment. The main goal is to make a UAV able to adjust its control policy in a dynamic environment. The UAV tracking control task is transformed into a Markov decision process (MDP) and further investigated using an incremental reinforcement-learning-based method. First, a policy relief (PR) method is used to make UAVs capable of performing an appropriate exploration in a new environment. In this way, a UAV controller can mitigate the conflict between a new environment and the current knowledge to ensure better adaptability to a dynamic environment. In addition, a significance weighting (SW) method is developed to improve the utilization of episodes with higher importance and richer information. In the proposed method, learning episodes that include more useful information are assigned with higher importance weights. The numerical simulation, hardware-in-the-loop (HITL) experiments, and real-world flight experiments are conducted to evaluate the performance of the proposed method. The results demonstrate high accuracy and effectiveness and good robustness of the proposed control algorithm in a dynamic flight environment.
更多查看译文
关键词
uav tracking control,reinforcement learning,wind disturbances environments
AI 理解论文
溯源树
样例
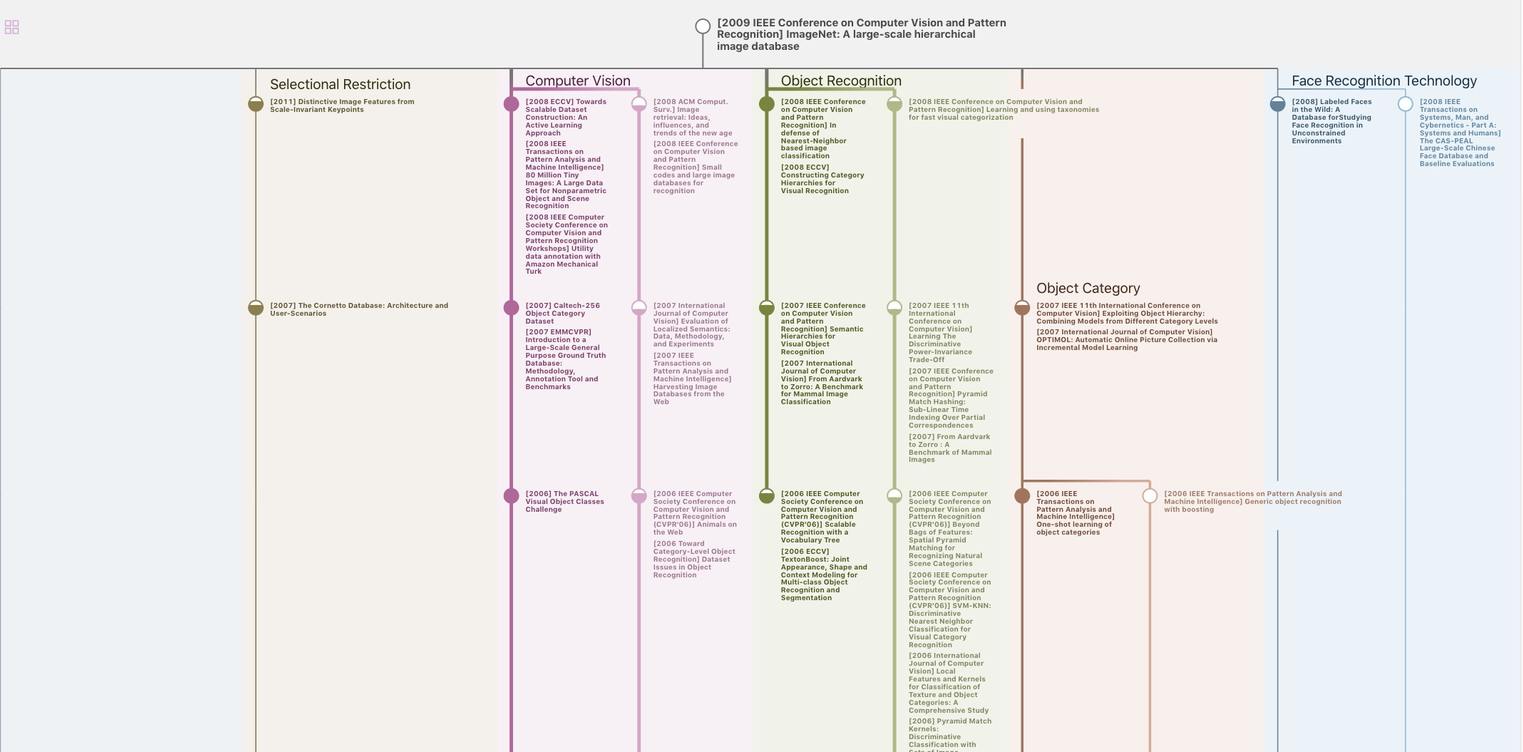
生成溯源树,研究论文发展脉络
Chat Paper
正在生成论文摘要