A Lifelong Learning Method Based on Generative Feature Replay for Bearing Diagnosis With Incremental Fault Types
IEEE Trans. Instrum. Meas.(2023)
摘要
Deep learning (DL)-based fault diagnosis models need to collect sufficient fault information for each fault type to ensure high-precision diagnosis. Some unexpected and new fault types will inevitably appear in actual conditions, which are called incremental fault types or class increments. Traditional DL models require the costly collection of all known data for retraining; while the use of new fault data may lead to catastrophic forgetting of old tasks. To solve the problem of bearing diagnosis with incremental fault types, a lifelong learning method based on generative feature replay (LLMGFR) is proposed in this study. A feature distillation method is put forward in this method to avoid forgetting in the feature extractor. The generator is trained to produce old task features. The generated features are mixed with real features of the current task to solve the imbalance problem and catastrophic forgetting of the classifier effectively. According to incremental fault diagnosis cases, LLMGFR can learn constantly and adaptively in dynamic environments with incremental fault types.
更多查看译文
关键词
Task analysis,Feature extraction,Fault diagnosis,Generators,Training,Learning systems,Generative adversarial networks,Catastrophic forgetting,class increment,fault diagnosis,lifelong learning
AI 理解论文
溯源树
样例
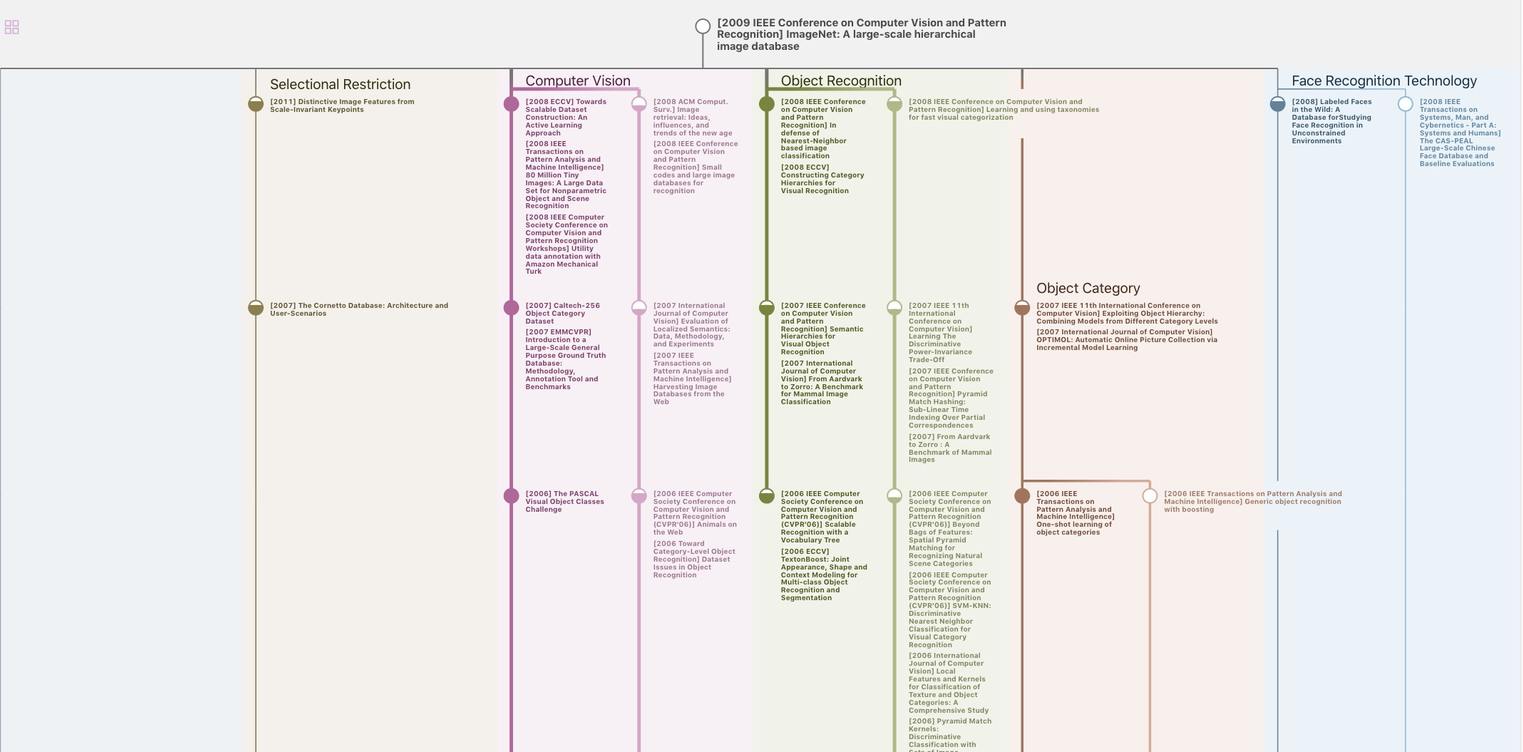
生成溯源树,研究论文发展脉络
Chat Paper
正在生成论文摘要