Deep Learning Aided Sound Source Localization: A Nonsynchronous Measurement Approach.
IEEE Trans. Instrum. Meas.(2023)
摘要
Nonsynchronous measurement (NSM) is able to improve the spatial resolution of beamforming and localization accuracy at the cost of longer recording time. This trade-off can be tolerated in various applications of sound source localization (SSL), such as machine fault diagnosis. Therefore, the NSM is a promising localization technique. For stationary acoustic field, the state-of-the-art NSM methods can well localize the stationary sources. However, when the sound sources are nonstationary, these conventional NSM algorithms fail to work effectively. In this work, we propose deep-learning-aided NSM (DL-NSM) to address the nonstationary source localization. Similar to the conventional NSM methods, the DL-NSM utilizes the diagonal blocks of an incomplete covariance matrix of the NSM to determine the nondiagonal elements of this matrix but differs in the fact that the DL-NSM can achieve the approximation to the covariance matrix of the corresponding synchronous measurement (SM). In the corresponding SM, a prototype array with a larger size and denser array elements is utilized to localize the sound sources, i.e., the covariance matrix of the corresponding SM can give the correlations of all array elements. This nonlinear approximation of the covariance matrix in the proposed DL-NSM can be learned by the proper training procedure. Therefore, the correlations of the NSM could be recovered, and the array aperture might be expanded, providing high-resolution localization. The simulation and experiment validate the superiority of the proposed DL-NSM.
更多查看译文
关键词
localization,deep learning,sound,non-synchronous
AI 理解论文
溯源树
样例
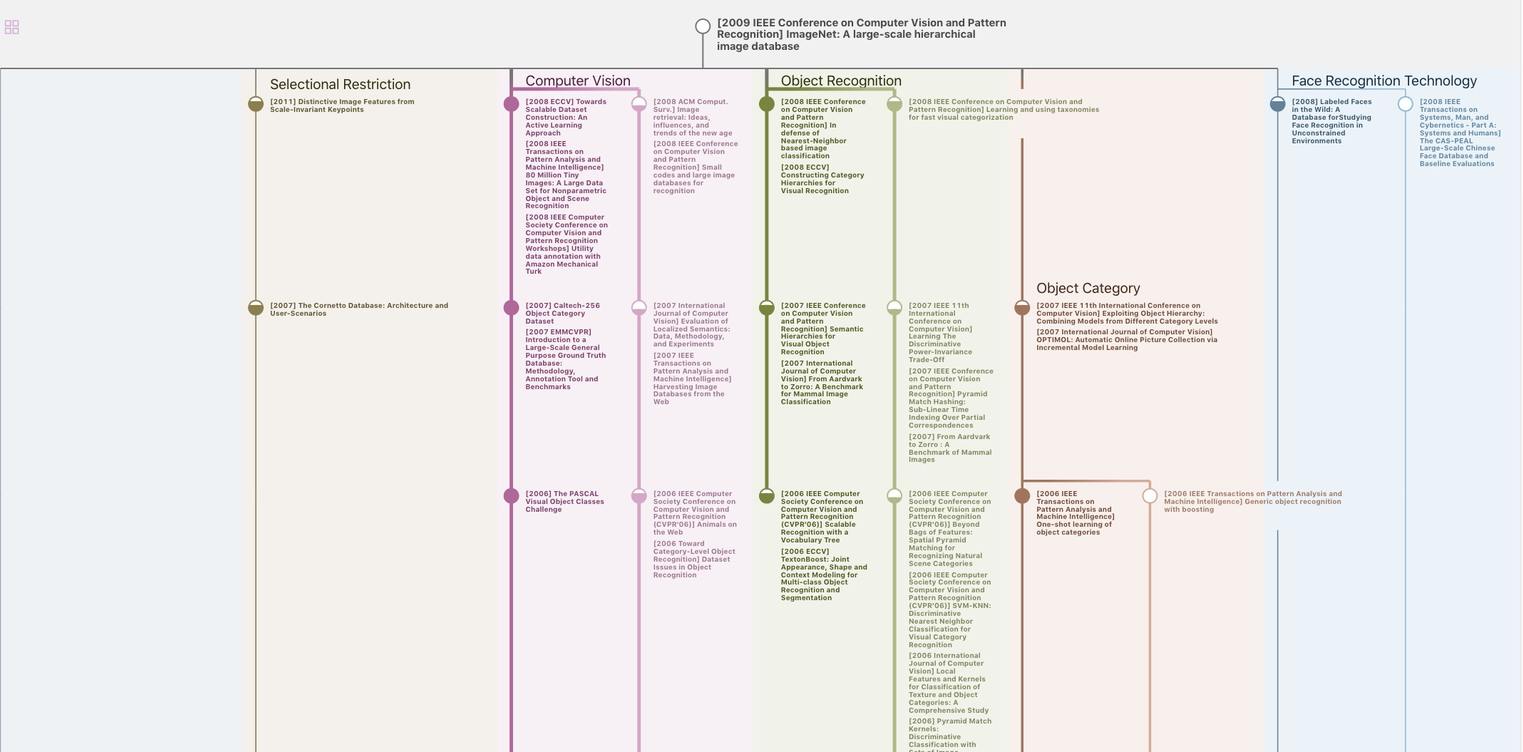
生成溯源树,研究论文发展脉络
Chat Paper
正在生成论文摘要