Adaptive Class Center Generalization Network: A Sparse Domain-Regressive Framework for Bearing Fault Diagnosis Under Unknown Working Conditions
IEEE Trans. Instrum. Meas.(2023)
摘要
Fault diagnosis is essential to ensure the bearing safety in smart manufacturing. As the rotating bearings usually work under variable working conditions, there may exist differences between the data distributions of the training and test domains. Domain adaptation fault diagnosis (DAFD) has been adopted to handle this domain shift phenomenon. But DAFD relies heavily on the target domain during its training process, while the target domain is always unavailable in real-world scenarios. To handle this situation, this article proposed a new adaptive class center generalization network (ACCGN). ACCGN is used to learn invariant feature representations of orientation signals from multiple source domains. First, ACCGN is used to learn the discriminative invariant fault feature from multisource domains, and it combines the sparse domain regression framework and central loss to optimize the data features from interclass and intraclass simultaneously. Second, a new adaptive method is proposed to update the center in central loss, and it can diminish the effect on the initialization center location. Third, a sparse domain regression framework is used to learn the interclass invariant features. The proposed ACCGN has been tested on two famous bearing datasets, and the results have shown the effectiveness of the proposed ACCGN on the CWRU and JNU datasets.
更多查看译文
关键词
Fault diagnosis,Feature extraction,Adaptation models,Training,Adaptive systems,Mathematical models,Employee welfare,Adaptive central loss,discriminative feature,domain generalization,fault diagnosis,sparse representation
AI 理解论文
溯源树
样例
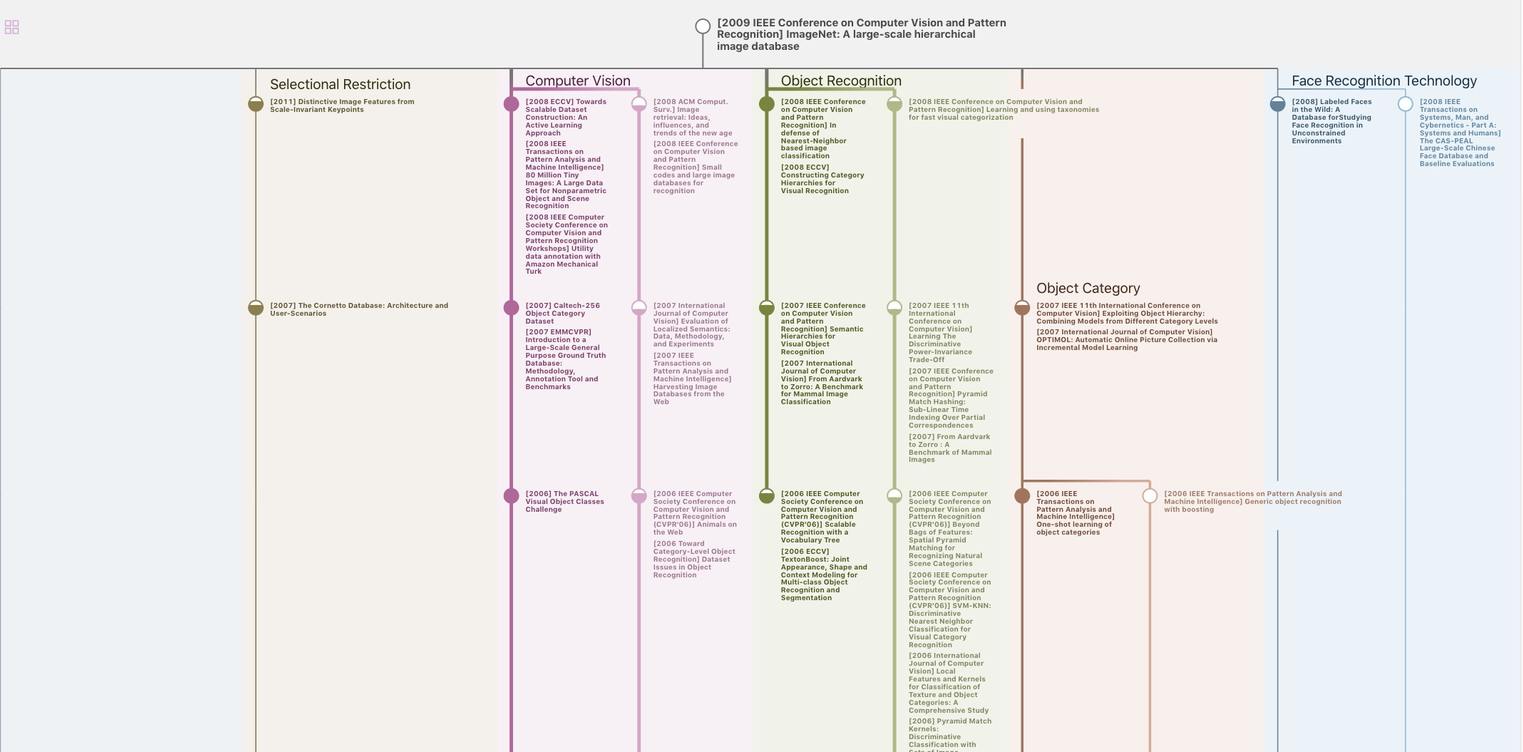
生成溯源树,研究论文发展脉络
Chat Paper
正在生成论文摘要