Suppression of chaos in a partially driven recurrent neural network
PHYSICAL REVIEW RESEARCH(2024)
摘要
The dynamics of recurrent neural networks (RNNs), and particularly their response to inputs, play a critical role in information processing. In many applications of RNNs, only a specific subset of the neurons generally receive inputs. However, it remains to be theoretically clarified how the restriction of the input to a specific subset of neurons affects the network dynamics. Considering RNNs with such restricted input, we investigate how the proportion, p, of the neurons receiving inputs (the "input neurons") and the strength of the input signals affect the dynamics by analytically deriving the conditional maximum Lyapunov exponent. Our results show that for sufficiently large p, the maximum Lyapunov exponent decreases monotonically as a function of the input strength, indicating the suppression of chaos, but if p is smaller than a critical threshold, pc, even significantly amplified inputs cannot suppress spontaneous chaotic dynamics. Furthermore, although the value of pc is seemingly dependent on several model parameters, such as the sparseness and strength of recurrent connections, it is proved to be intrinsically determined solely by the strength of chaos in spontaneous activity of the RNN. This is to say, despite changes in these model parameters, it is possible to represent the value of pc as a common invariant function by appropriately scaling these parameters to yield the same strength of spontaneous chaos. Our study suggests that if p is above pc, we can bring the neural network to the edge of chaos, thereby maximizing its information processing capacity, by amplifying inputs.
更多查看译文
AI 理解论文
溯源树
样例
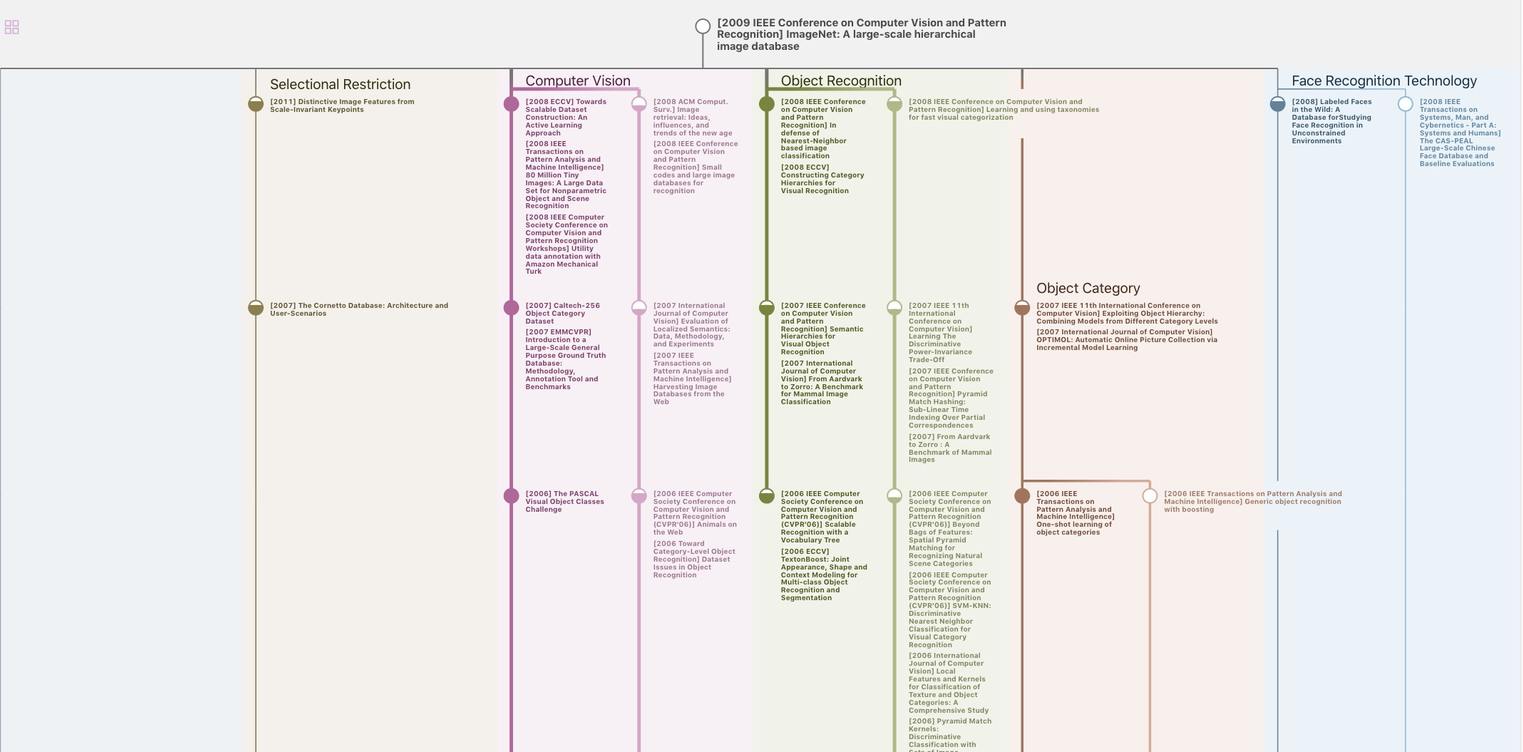
生成溯源树,研究论文发展脉络
Chat Paper
正在生成论文摘要