Graph-Based Model-Agnostic Data Subsampling for Recommendation Systems
PROCEEDINGS OF THE 29TH ACM SIGKDD CONFERENCE ON KNOWLEDGE DISCOVERY AND DATA MINING, KDD 2023(2023)
摘要
Data subsampling is widely used to speed up the training of large-scale recommendation systems. Most subsampling methods are model-based and often require a pre-trained pilot model to measure data importance via e.g. sample hardness. However, when the pilot model is misspecified, model-based subsampling methods deteriorate. Since model misspecification is persistent in real recommendation systems, we instead propose model-agnostic data subsampling methods by only exploring input data structure represented by graphs. Specifically, we study the topology of the user-item graph to estimate the importance of each user-item interaction (an edge in the user-item graph) via graph conductance, followed by a propagation step on the network to smooth out the estimated importance value. Since our proposed method is model-agnostic, we can marry the merits of both model-agnostic and model-based subsampling methods. Empirically, we show that combing the two consistently improves over any single method on the used datasets. Experimental results on KuaiRec and MIND datasets demonstrate that our proposed methods achieve superior results compared to baseline approaches.
更多查看译文
关键词
Recommender systems,Data subsampling,Network analysis
AI 理解论文
溯源树
样例
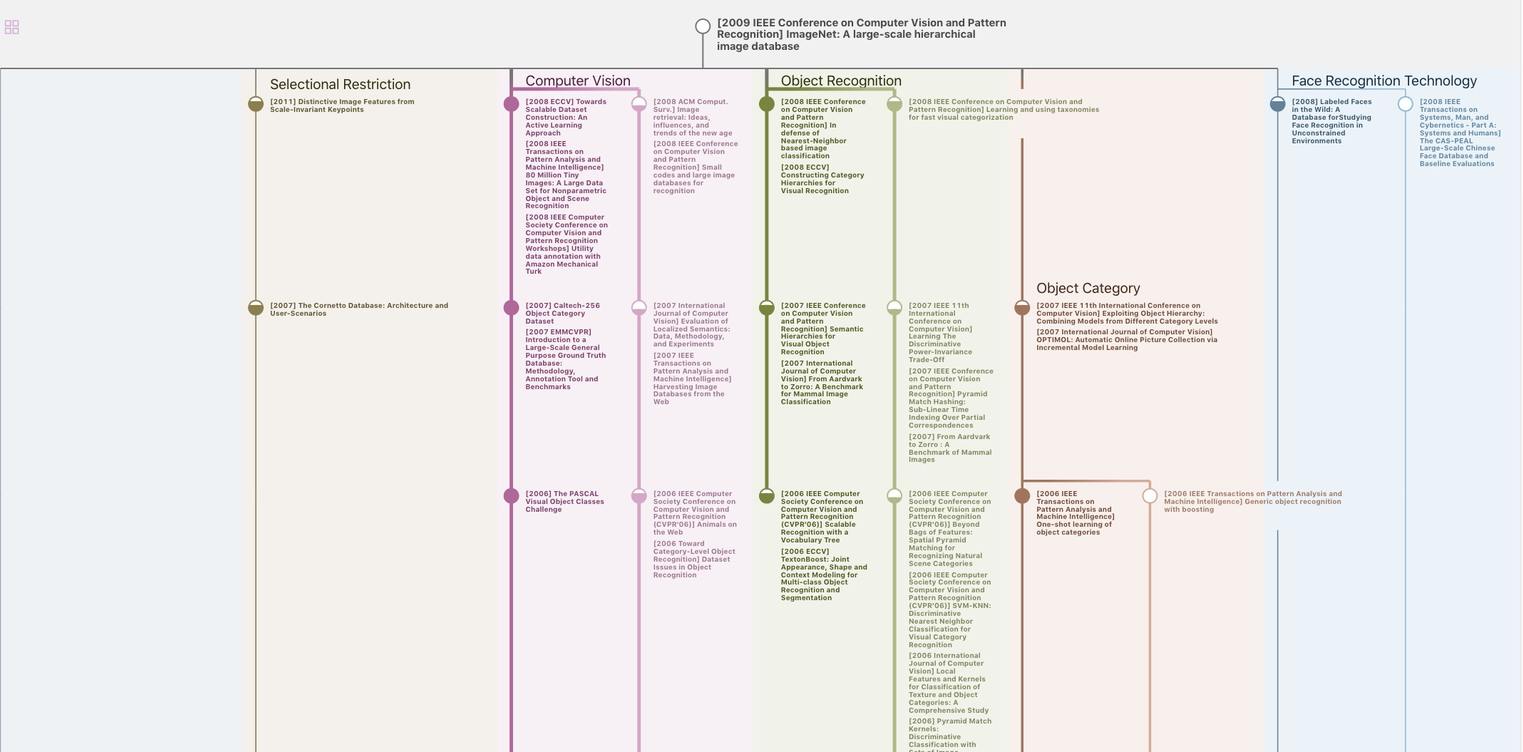
生成溯源树,研究论文发展脉络
Chat Paper
正在生成论文摘要