Learning networks hyper-parameter using multi-objective optimization of statistical performance metrics
2022 24th International Symposium on Symbolic and Numeric Algorithms for Scientific Computing (SYNASC)(2022)
摘要
Deep Learning has enabled remarkable progress over the last years on a several objectives, such as image and speech recognition, and machine translation. Deep neural architectures are a main contribution for this progress. Current architectures have mostly been developed manually by engineers, which is a time-consuming and error-prone process. Because of this, there is growing interest in automated neural architecture search methods. In this paper we present a strategy for the optimization of network hyper-parameters using a multi-objective Non-dominated Sorting Genetic Algorithm combined with a nested cross-validation to optimize statistical metrics of the performance of networks. In order to illustrate the proposed hyper-parameter optimization, we have applied it to a use case that uses transformers to map abstract radiomic features to specific radiological annotations. Results obtained with the LUNA16 public data base show generalization power of the proposed optimization strategy for hyper-parameter setting.
更多查看译文
关键词
meta-learning, optimization, deep learning, network hyper-parameters, multi-objective, HPO
AI 理解论文
溯源树
样例
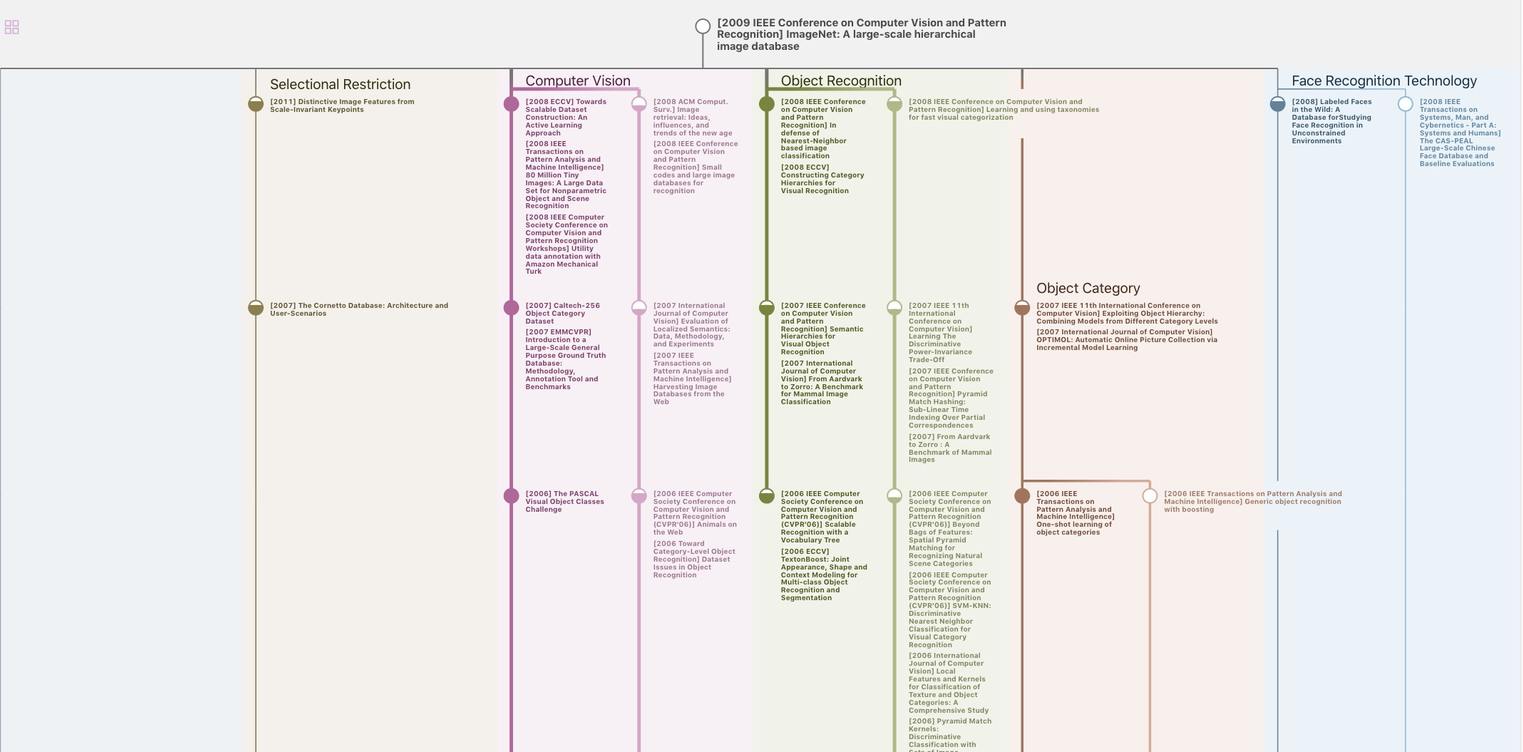
生成溯源树,研究论文发展脉络
Chat Paper
正在生成论文摘要