Large-language-model-based 10-year risk prediction of cardiovascular disease: insight from the UK biobank data
medRxiv (Cold Spring Harbor Laboratory)(2023)
摘要
Background: Conventional cardiovascular risk prediction models provide insights into population-level risk factors and have been widely adopted in clinical practice. However, these models have limited generalizability and flexibility. Large language models (LLMs) have demonstrated remarkable proficiency for use in various industries. Methods: In this study, we have investigated the feasibility of Large Language Models (LLMs) such as ChatGPT-3.5, ChatGPT-4, and Bard for predicting 10-year cardiovascular risk of a patient. We used data from the UK Biobank Cohort, a major biomedical database in the UK, and the Korean Genome and Epidemiology Study (KoGES), a large-scale prospective study in Korea, for additional validation and multi-institutional research. These databases provided a wide array of information including age, sex, medical history, lipid profile, blood pressure, and physical measurement. Based on these data, we generated language sentences for individual analysis and input these into the LLM to derive results. The performance of the LLMs was then compared with the Framingham Risk Score (FRS), a conventional risk prediction model, using this real-world data. We confirmed the model performance of both the LLMs and FRS, evaluating their accuracy, sensitivity, specificity, Positive Predictive Value (PPV), Negative Predictive Value (NPV), and F1 score. Their performance in predicting 10-year cardiovascular risk was compared through Kaplan–Meier survival analysis and Cox-hazard ratio analysis. Findings: GPT-4 achieved performance comparable to the FRS in cardiovascular risk prediction in both the UK Biobank {accuracy (0.834 vs 0.773) and F1 score (0.138 vs 0.132)} and KoGES {accuracy (0.902 vs 0.874)}. The Kaplan–Meier survival analysis of GPT-4 demonstrated distinct survival patterns among groups, which revealed a strong association between the GPT risk prediction output and survival outcomes. The additional analysis of limited variables using GPT-3.5 indicated that ChatGPT′s prediction performance was preserved despite the omission of a few variables in the prompt, especially without physical measurement data Interpretation: This study proposed that ChatGPT can achieve performance comparable to conventional models in predicting cardiovascular risk. Furthermore, ChatGPT exhibits enhanced accessibility, flexibility, and the ability to provide user-friendly outputs. With the evolution of LLMs, such as ChatGPT, studies should focus on applying LLMs to various medical scenarios and subsequently optimizing their performance.
### Competing Interest Statement
The authors have declared no competing interest.
### Funding Statement
This research was supported by a grant from the Korea Health Technology R&D Project through the Korea Health Industry Development Institute (KHIDI), funded by the Ministry of Health and Welfare, Republic of Korea (grant number: HI22C0452).
### Author Declarations
I confirm all relevant ethical guidelines have been followed, and any necessary IRB and/or ethics committee approvals have been obtained.
Yes
The details of the IRB/oversight body that provided approval or exemption for the research described are given below:
UK Biobank : https://www.ukbiobank.ac.uk/ Korean Genome and Epidemiology Study (KoGES) data : https://nih.go.kr/eng/main/main.do
I confirm that all necessary patient/participant consent has been obtained and the appropriate institutional forms have been archived, and that any patient/participant/sample identifiers included were not known to anyone (e.g., hospital staff, patients or participants themselves) outside the research group so cannot be used to identify individuals.
Yes
I understand that all clinical trials and any other prospective interventional studies must be registered with an ICMJE-approved registry, such as ClinicalTrials.gov. I confirm that any such study reported in the manuscript has been registered and the trial registration ID is provided (note: if posting a prospective study registered retrospectively, please provide a statement in the trial ID field explaining why the study was not registered in advance).
Yes
I have followed all appropriate research reporting guidelines, such as any relevant EQUATOR Network research reporting checklist(s) and other pertinent material, if applicable.
Yes
All data produced in the present work are contained in the manuscript.
更多查看译文
关键词
cardiovascular disease,risk,prediction,large-language-model-based
AI 理解论文
溯源树
样例
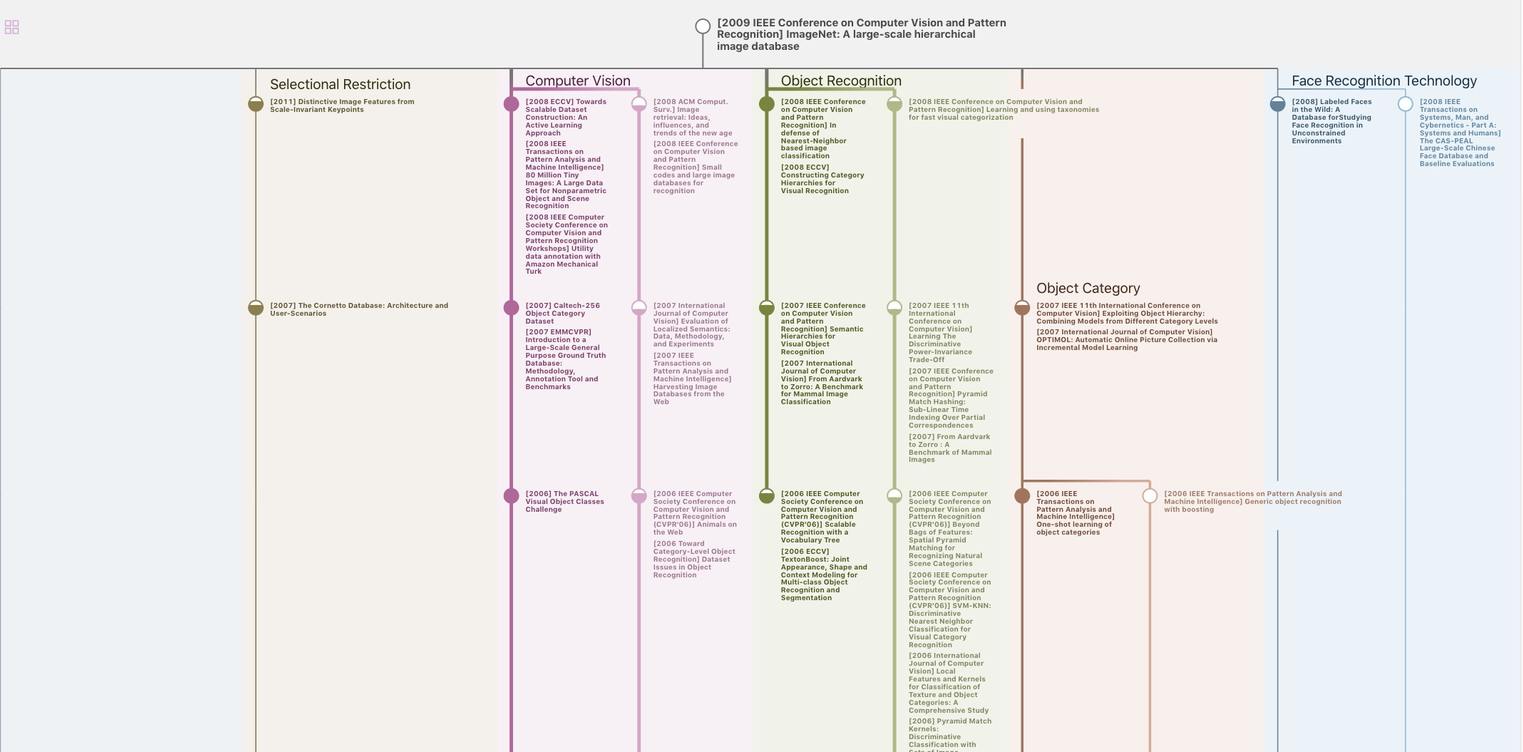
生成溯源树,研究论文发展脉络
Chat Paper
正在生成论文摘要