Isolation Forest Based Anomaly Detection Approach for Wireless Body Area Networks
Hybrid Intelligent Systems Lecture Notes in Networks and Systems(2023)
摘要
Anomalous data detection is an important task for ensuring the quality of data in many real-world applications. Medical healthcare services are one such application where Wireless Body Area Networks (WBAN) is used to track human health situations. Such tracking is achieved by collecting and monitoring the basic physiological vital signs and making them available to the healthcare givers to assess the criticality status of patients, especially in Intensive care units (ICU). Various anomaly detection approaches have been proposed for detecting anomalies collected in WBAN such as statistical, machine learning and deep learning techniques. However, the lack of ground truth data made the job of training such models a difficulty in supervised settings. In this paper, an Isolation Forest-based anomaly detection approach for WBAN (iForestBAN-AD) model is proposed. The iForest technique is fully unsupervised and does not employ any distance measure or density function like most existing techniques and rather detects anomalies based on the concept of isolation. To evaluate the proposed approach, experiments on data samples from real world physiological network records (Physionet) were conducted. The results show the viability of the proposed approach as it achieves around 95% AUC and outperforms many of the existing baseline unsupervised techniques on multivariate dataset samples.
更多查看译文
关键词
Anomaly Detection, Wireless Body Area Network, Isolation Forest, Unsupervised Learning, Internet of Medical Things
AI 理解论文
溯源树
样例
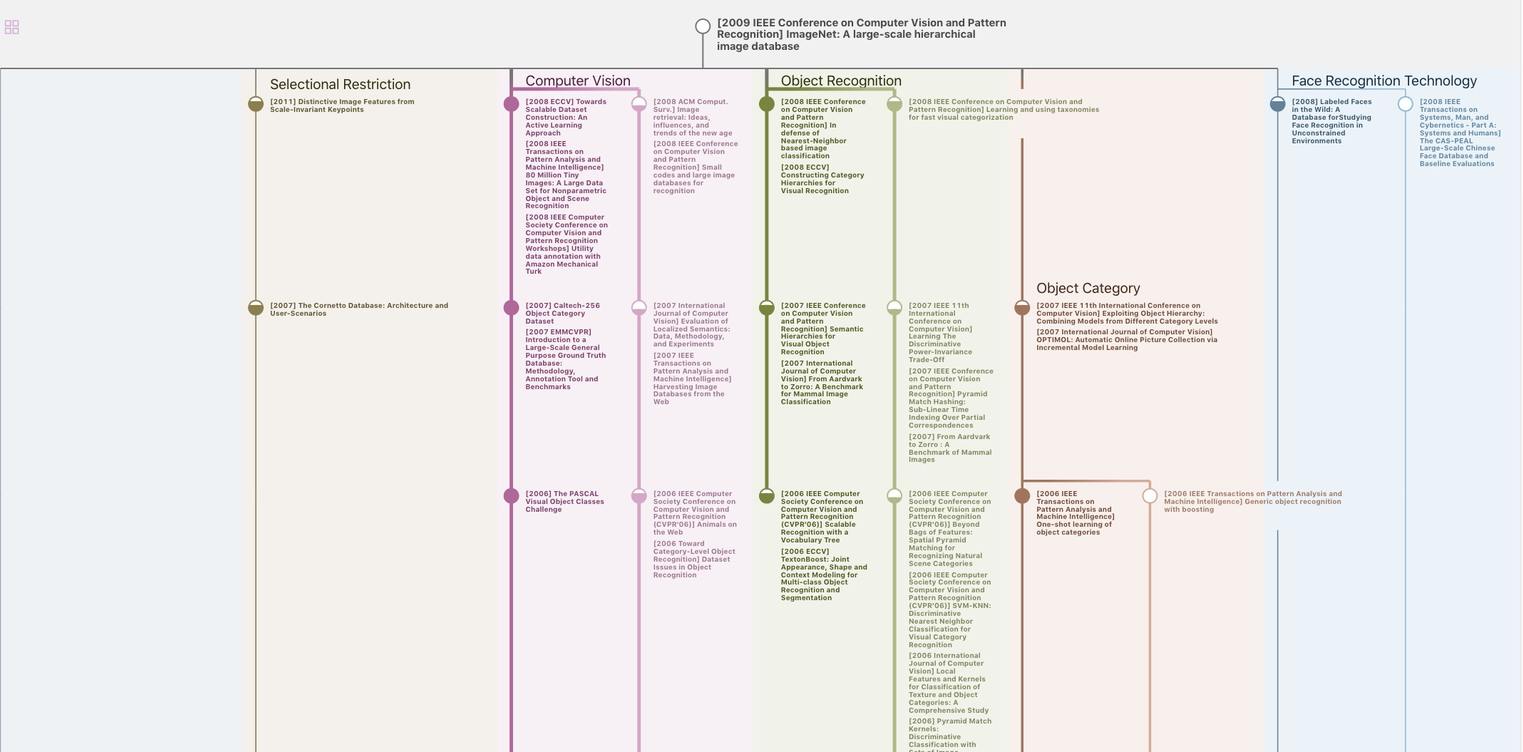
生成溯源树,研究论文发展脉络
Chat Paper
正在生成论文摘要