Pathformer: biological pathway informed Transformer model integrating multi-modal data of cancer
crossref(2023)
摘要
Multi-modal biological data integration can provide comprehensive views of gene regulation and cell development. However, conventional integration methods rarely utilize prior biological knowledge and lack interpretability. To address these challenges, we developed Pathformer, a biological pathway informed deep learning model based on Transformer with bias to integrate multi-modal data. Pathformer leverages criss-cross attention mechanism to capture crosstalk between different biological pathways and between different modalities (i.e., multi-omics). It also utilizes SHapley Additive Explanation method to reveal key pathways, genes, and regulatory mechanisms. Through benchmark studies on 28 TCGA datasets, we demonstrated the superior performance and interpretability of Pathformer on various cancer classification tasks, compared to other integration models. Furthermore, we applied Pathformer to liquid biopsy multi-modal data integration with high accuracy in cancer diagnosis. Meanwhile, Pathformer revealed interesting molecularly altered pathways in cancer patients’ body fluid, such as ligand binding of scavenger receptors, iron transport, and DAP12 signaling transmission, which are related to extracellular vesicle transport, platelet, and immune response.
### Competing Interest Statement
The authors have declared no competing interest.
更多查看译文
AI 理解论文
溯源树
样例
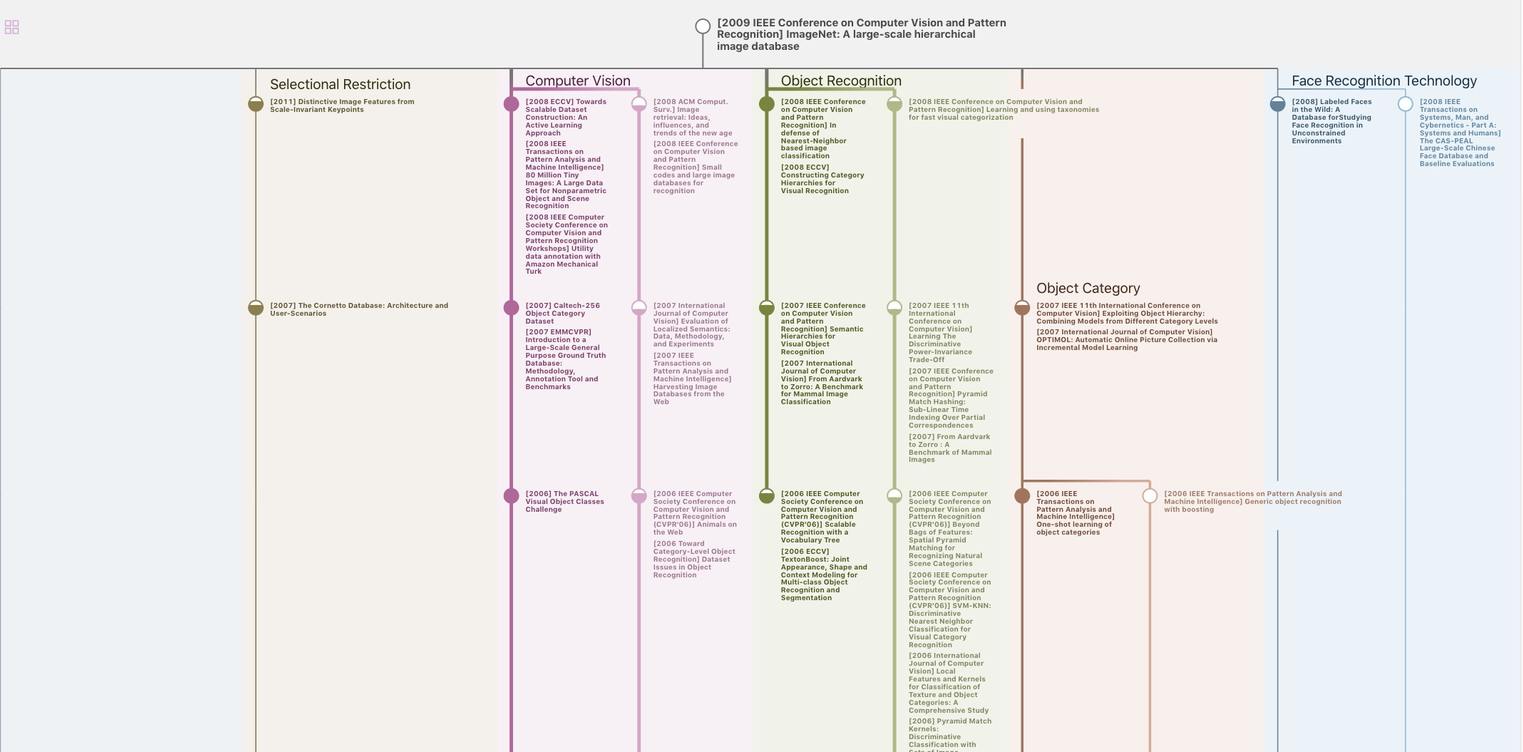
生成溯源树,研究论文发展脉络
Chat Paper
正在生成论文摘要