Instruct-Align: Teaching Novel Languages with to LLMs through Alignment-based Cross-Lingual Instruction
CoRR(2023)
Abstract
Instruction-tuned large language models (LLMs) have shown remarkable generalization capability over multiple tasks in multiple languages. Nevertheless, their generalization towards different languages varies especially to underrepresented languages or even to unseen languages. Prior works on adapting new languages to LLMs find that naively adapting new languages to instruction-tuned LLMs will result in catastrophic forgetting, which in turn causes the loss of multitasking ability in these LLMs. To tackle this, we propose the Instruct-Align a.k.a (IA)$^1$ framework, which enables instruction-tuned LLMs to learn cross-lingual alignment between unseen and previously learned languages via alignment-based cross-lingual instruction-tuning. Our preliminary result on BLOOMZ-560M shows that (IA)$^1$ is able to learn a new language effectively with only a limited amount of parallel data and at the same time prevent catastrophic forgetting by applying continual instruction-tuning through experience replay. Our work contributes to the progression of language adaptation methods for instruction-tuned LLMs and opens up the possibility of adapting underrepresented low-resource languages into existing instruction-tuned LLMs. Our code will be publicly released upon acceptance.
MoreTranslated text
AI Read Science
Must-Reading Tree
Example
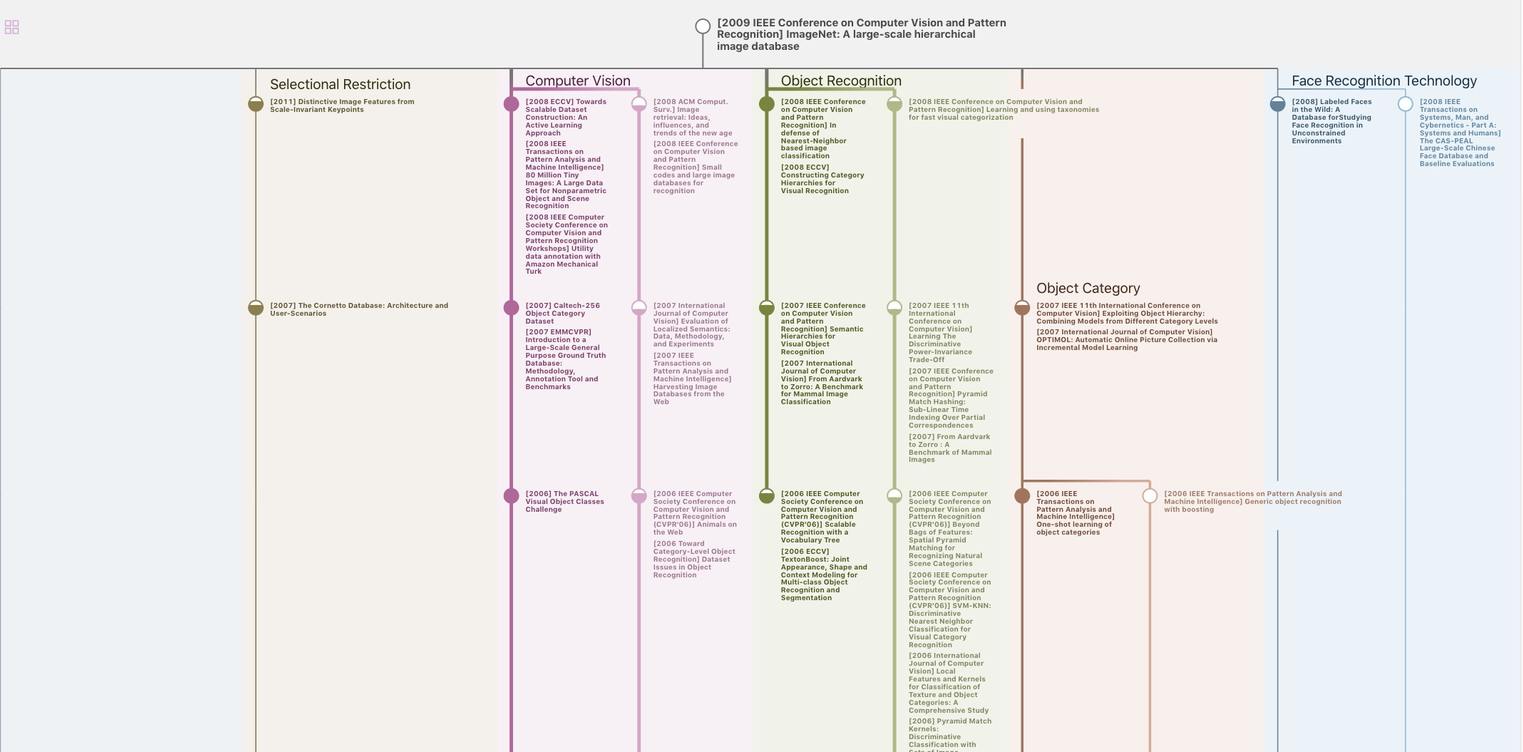
Generate MRT to find the research sequence of this paper
Chat Paper
Summary is being generated by the instructions you defined