Extrapolating Multilingual Understanding Models as Multilingual Generators.
CoRR(2023)
摘要
Multilingual understanding models (or encoder-based), pre-trained via masked language modeling, have achieved promising results on many language understanding tasks (e.g., mBERT). However, these non-autoregressive (NAR) models still struggle to generate high-quality texts compared with autoregressive (AR) models. Considering that encoder-based models have the advantage of efficient generation and self-correction abilities, this paper explores methods to empower multilingual understanding models the generation abilities to get a unified model. Specifically, we start from a multilingual encoder (XLM-R) and propose a \textbf{S}emantic-\textbf{G}uided \textbf{A}lignment-then-Denoising (SGA) approach to adapt an encoder to a multilingual generator with a small number of new parameters. Experiments show that the proposed approach is an effective adaption method, outperforming widely-used initialization-based methods with gains of 9.4 BLEU on machine translation, 8.1 Rouge-L on question generation, and 5.5 METEOR on story generation on XLM-R$_{large}$. On the other hand, we observe that XLM-R is still inferior to mBART in supervised settings despite better results on zero-shot settings, indicating that more exploration is required to make understanding models strong generators.
更多查看译文
关键词
multilingual understanding models
AI 理解论文
溯源树
样例
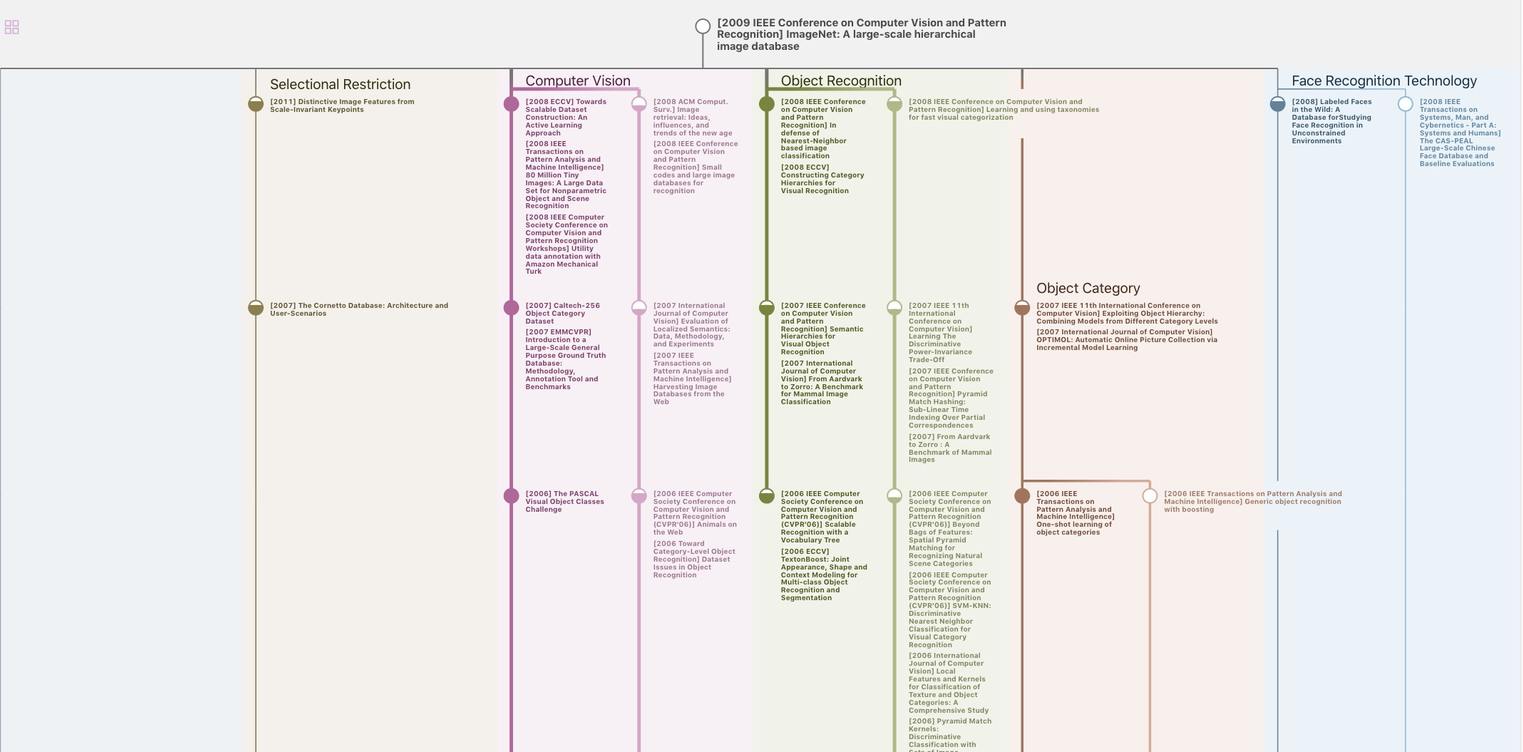
生成溯源树,研究论文发展脉络
Chat Paper
正在生成论文摘要