OntoType: Ontology-Guided Zero-Shot Fine-Grained Entity Typing with Weak Supervision from Pre-Trained Language Models
CoRR(2023)
摘要
Fine-grained entity typing (FET), which assigns entities in text with context-sensitive, fine-grained semantic types, will play an important role in natural language understanding. A supervised FET method, which typically relies on human-annotated corpora for training, is costly and difficult to scale. Recent studies leverage pre-trained language models (PLMs) to generate rich and context-aware weak supervision for FET. However, a PLM may still generate a mixture of rough and fine-grained types, or tokens unsuitable for typing. In this study, we vision that an ontology provides a semantics-rich, hierarchical structure, which will help select the best results generated by multiple PLM models and head words. Specifically, we propose a novel zero-shot, ontology-guided FET method, OntoType, which follows a type ontological structure, from coarse to fine, ensembles multiple PLM prompting results to generate a set of type candidates, and refines its type resolution, under the local context with a natural language inference model. Our experiments on the Ontonotes, FIGER, and NYT datasets using their associated ontological structures demonstrate that our method outperforms the state-of-the-art zero-shot fine-grained entity typing methods. Our error analysis shows that refinement of the existing ontology structures will further improve fine-grained entity typing.
更多查看译文
关键词
ontotype,language models,supervision,ontology-guided,zero-shot,fine-grained,pre-trained
AI 理解论文
溯源树
样例
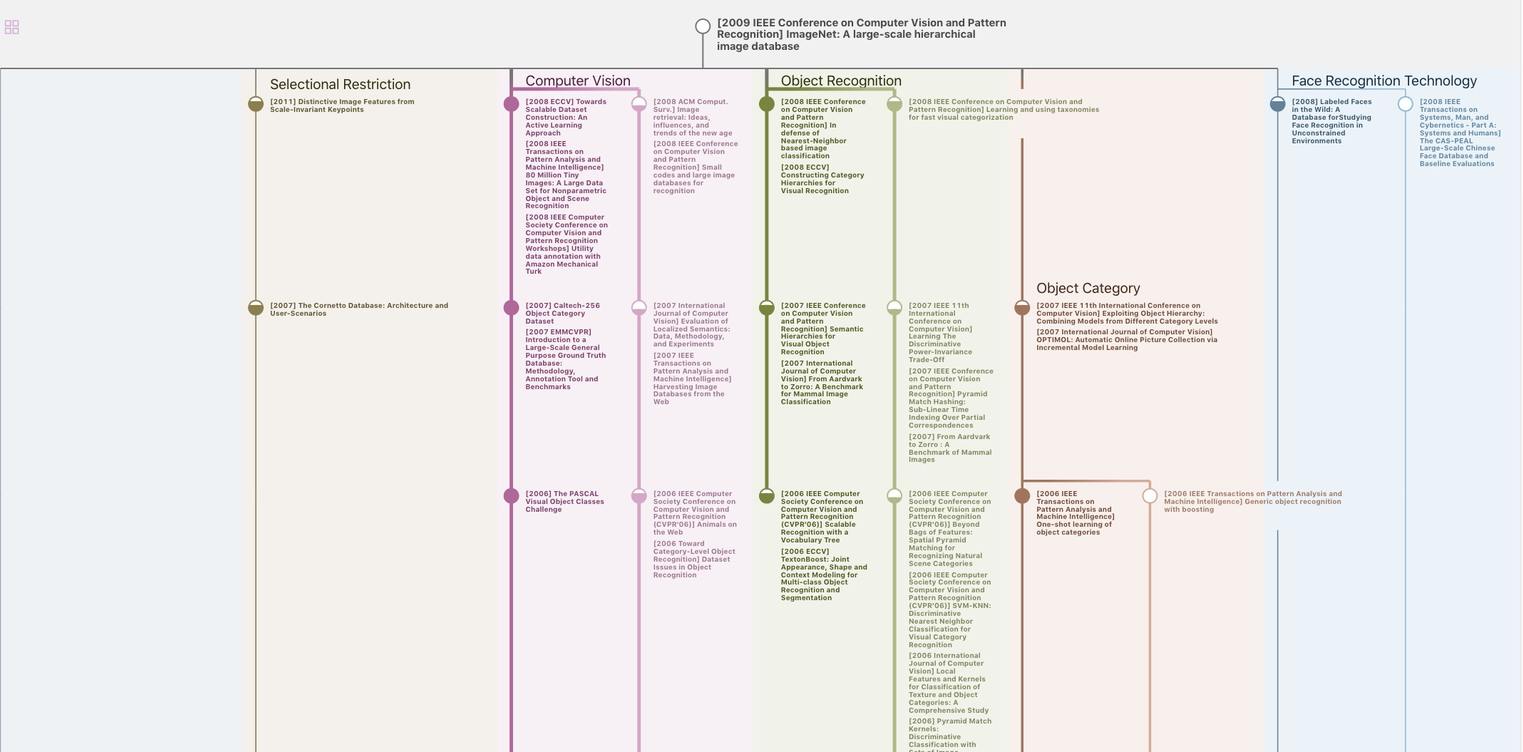
生成溯源树,研究论文发展脉络
Chat Paper
正在生成论文摘要