On Stochastic Codebook Generation for Markov Sources
2023 Data Compression Conference (DCC)(2023)
摘要
This paper proposes an effective universal (on-the-Hy’’ mechanism for stochastic codebook generation in lossy coding of Markov sources. Earlier work has shown that the ratedistortion bound can be asymptotically achieved by a “natural type selection” (NTS) mechanism that iteratively considers asymptotically long source strings (from an unknown distribution P) and regenerates the codebook from a distribution obtained within a maximum likelihood distribution estimation framework, based on observation of a set of K codewords that “d-match’’ (i.e., satisfy the distortion constraint for) a respective set of K independently generated source words. This result was later generalized, in a straightforward manner, to account for source memory, by considering the source as a vector source, i.e., a sequence of super-symbols from a corresponding super-alphabet. While ensuring asymptotic optimality, this extension suffered from a significant practical flaw: it requires asymptotically long vectors or super-symbols, hence exponentially large super-alphabet, in order to approach the rate-distortion bound, even for finite memory sources, e.g., Markov sources. Such exponentially large super-alphabet implies that even a single NTS iteration is intractable, thus compromising the promise of NTS to approach the rate-distortion function, in practice, for sources with memory. This work describes a considerably more efficient and tractable mechanism to achieve asymptotically optimal performance given a prescribed memory constraint, within a practical framework tailored to Markov sources. Specifically, the algorithm finds, asymptotically, the optimal codebook reproduction distribution, within a constrained set of distributions satisfying a prescribed Markovian property, e.g., of the same order as the source, which achieves the minimum per letter coding rate while maintaining a specified distortion level.
更多查看译文
关键词
Natural Type Selection,Random Coding,Rate Distortion Theory,Markov Sources
AI 理解论文
溯源树
样例
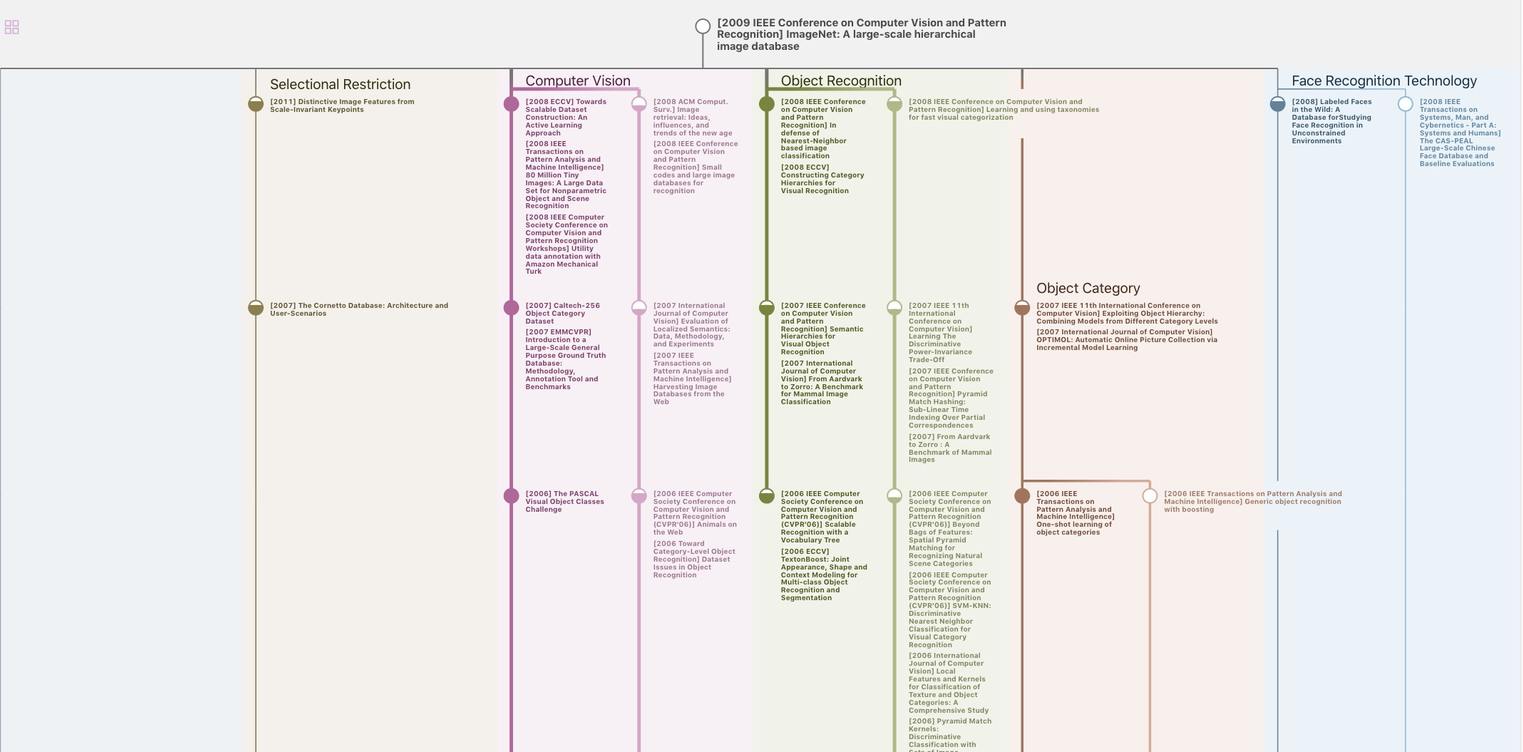
生成溯源树,研究论文发展脉络
Chat Paper
正在生成论文摘要