Hybrid Weighted Retrieval of Twitter Users for Temporally Relevant Full-Text Querying in the Media Industry
2022 13th International Congress on Advanced Applied Informatics Winter (IIAI-AAI-Winter)(2022)
摘要
Barriers to the delivery of journalistic content to suitable media outlets present difficulties to both journalists and publishing houses. These may take the form of barriers to the identification of key individuals to whom the content is relevant, and who may be influential within their domain topic. To address this problem, we present a methodological approach to automated recommender systems for journalists or content-writers, which enables the identification of social media accounts, based upon information retrieval techniques. Firstly, this work investigates three concepts, which to our knowledge have yet to be applied to such a domain; firstly, how user-defined descriptions, commonly associated with user profiles on the Twitter platform, may impact upon the perceived accuracy of recommendations. Secondly, the application of a full-text query facility to accept journalistic-content queries. Finally, an investigation into time-decay, as part of the recommendation-ranking methodology. This results in a proposed novel hybrid weighting methodology, which provides advantages to baseline information retrieval techniques, and demonstrates the influence of temporal decay ranking upon model performance. As part of this work, a vectorised tweet dataset is provided, entailing 390,750 unique tweets, for use in wider research. Finally, we demonstrate an implementation in a real-world commercial setting, and provide an anonymised data set to assist in future research into this domain.
更多查看译文
关键词
Information Retrieval,Social Media,Recommender Systems,Nearest- Neighbour
AI 理解论文
溯源树
样例
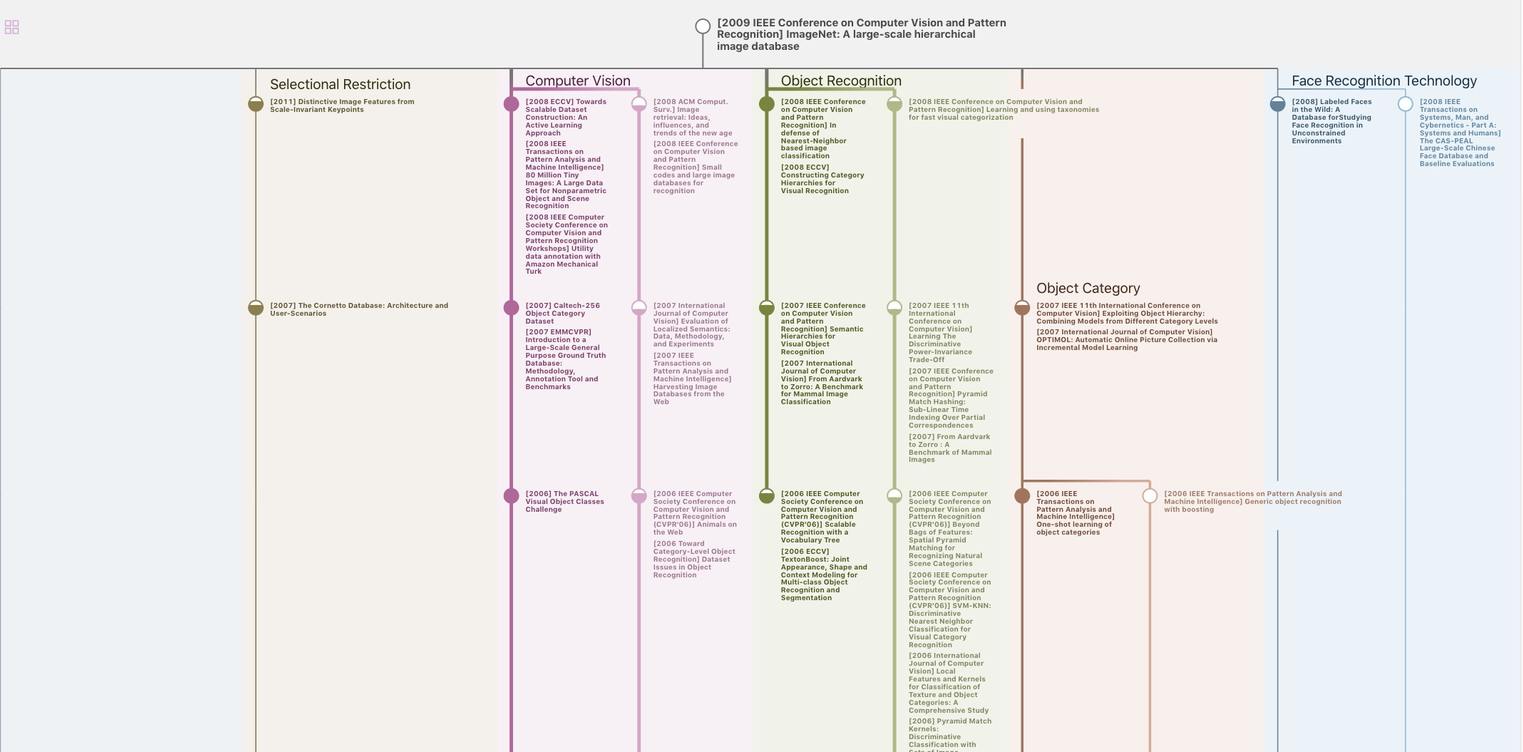
生成溯源树,研究论文发展脉络
Chat Paper
正在生成论文摘要