Hierarchical prompt learning for compositional zero-shot recognition
IJCAI 2023(2023)
摘要
Compositional Zero-Shot Learning (CZSL) aims to imitate the powerful generalization ability of human beings to recognize novel compositions of known primitive concepts that correspond to a state and an object, e.g ., "purple apple". To fully capture the intra- and inter-class correlations between compositional concepts, in this paper, we propose to learn them in a hierarchical manner. Specifically, we set up three hierarchical embedding spaces that respectively model the states, the objects, and their compositions, which serve as three "experts" that can be combined in inference for more accurate predictions. We achieve this based on the recent success of large-scale pretrained Vision-Language Models, e.g ., CLIP, which provides a strong initial knowledge of image-text relationships. To better adapt this knowledge to CZSL, we propose to learn three hierarchical prompts by explicitly fixing the unrelated word tokens in the three embedding spaces. Despite its simplicity, our proposed method consistently yields superior performance over current state-of-the-art approaches on three widely-used CZSL benchmarks.
更多查看译文
AI 理解论文
溯源树
样例
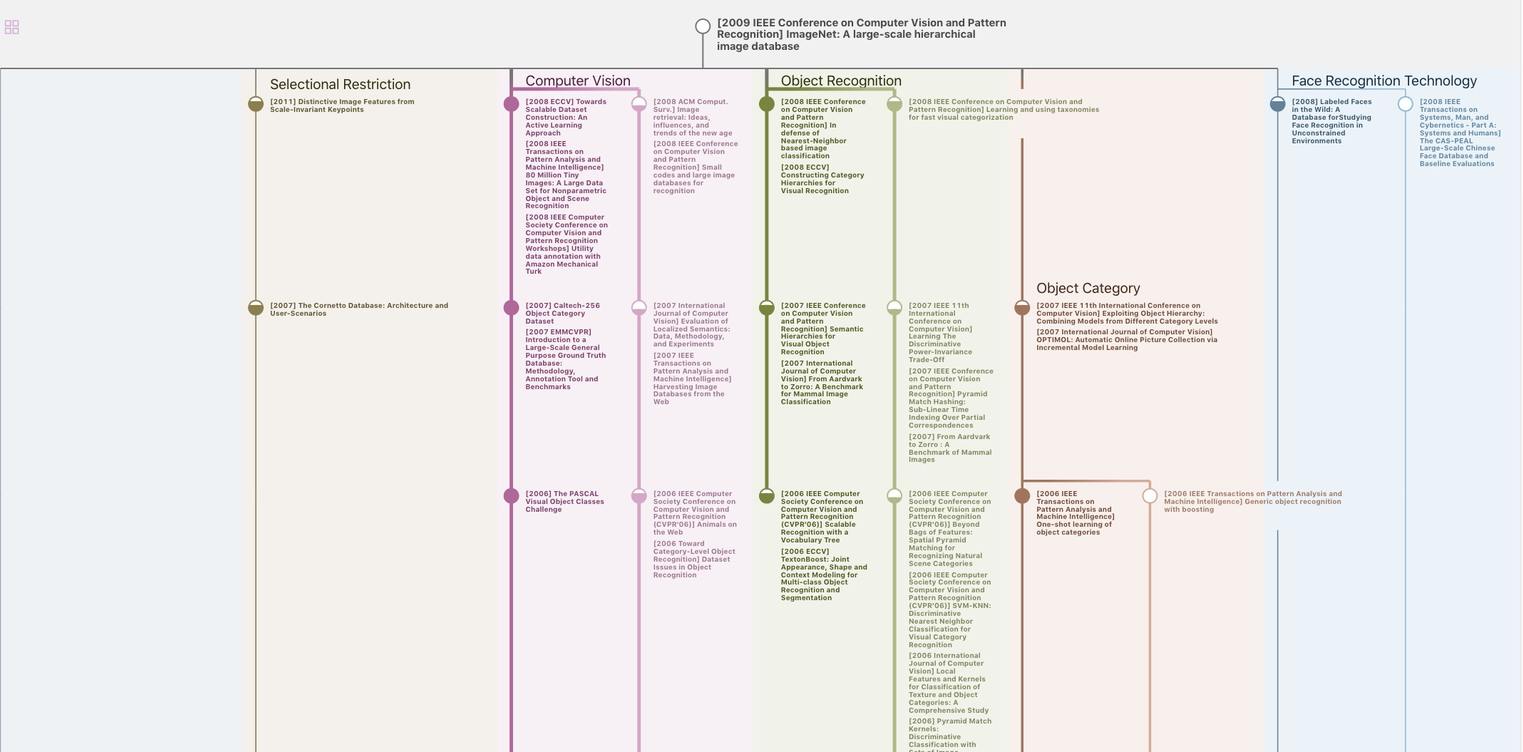
生成溯源树,研究论文发展脉络
Chat Paper
正在生成论文摘要