A Novel Exploitative and Explorative GWO-SVM Algorithm for Smart Emotion Recognition
IEEE Internet of Things Journal(2023)
摘要
Emotion recognition or detection is broadly utilized in patient–doctor interactions for diseases, such as schizophrenia and autism and the most typical techniques are speech detection and facial recognition. However, features extracted from these behavior-based emotion recognitions are not reliable since humans can disguise their emotions. Recording voices or tracking facial expressions for a long term is also not efficient. Therefore, our aim is to find a reliable and efficient emotion recognition scheme, which can be used for nonbehavior-based emotion recognition in real time. This can be solved by implementing a single-channel electrocardiogram (ECG)-based emotion recognition scheme in a lightweight embedded system. However, existing schemes have relatively low accuracy. For instance, the accuracy is about 82.78% by using a least squares support vector machine (SVM). Therefore, we propose a reliable and efficient emotion recognition scheme—exploitative and explorative gray wolf optimizer-based SVM (X-GWO-SVM) for ECG-based emotion recognition. Two data sets, one raw self-collected iRealcare data set, and the widely used benchmark WESAD data set are used in the X-GWO-SVM algorithm for emotion recognition. Leave-single-subject-out cross-validation yields a mean accuracy of 93.37% for the iRealcare data set and a mean accuracy of 95.93% for the WESAD data set. This work demonstrates that the X-GWO-SVM algorithm can be used for emotion recognition and the algorithm exhibits superior performance in reliability compared to the use of other supervised machine learning methods in earlier works. It can be implemented in a lightweight embedded system, which is much more efficient than existing solutions based on deep neural networks.
更多查看译文
关键词
emotion,gwo-svm
AI 理解论文
溯源树
样例
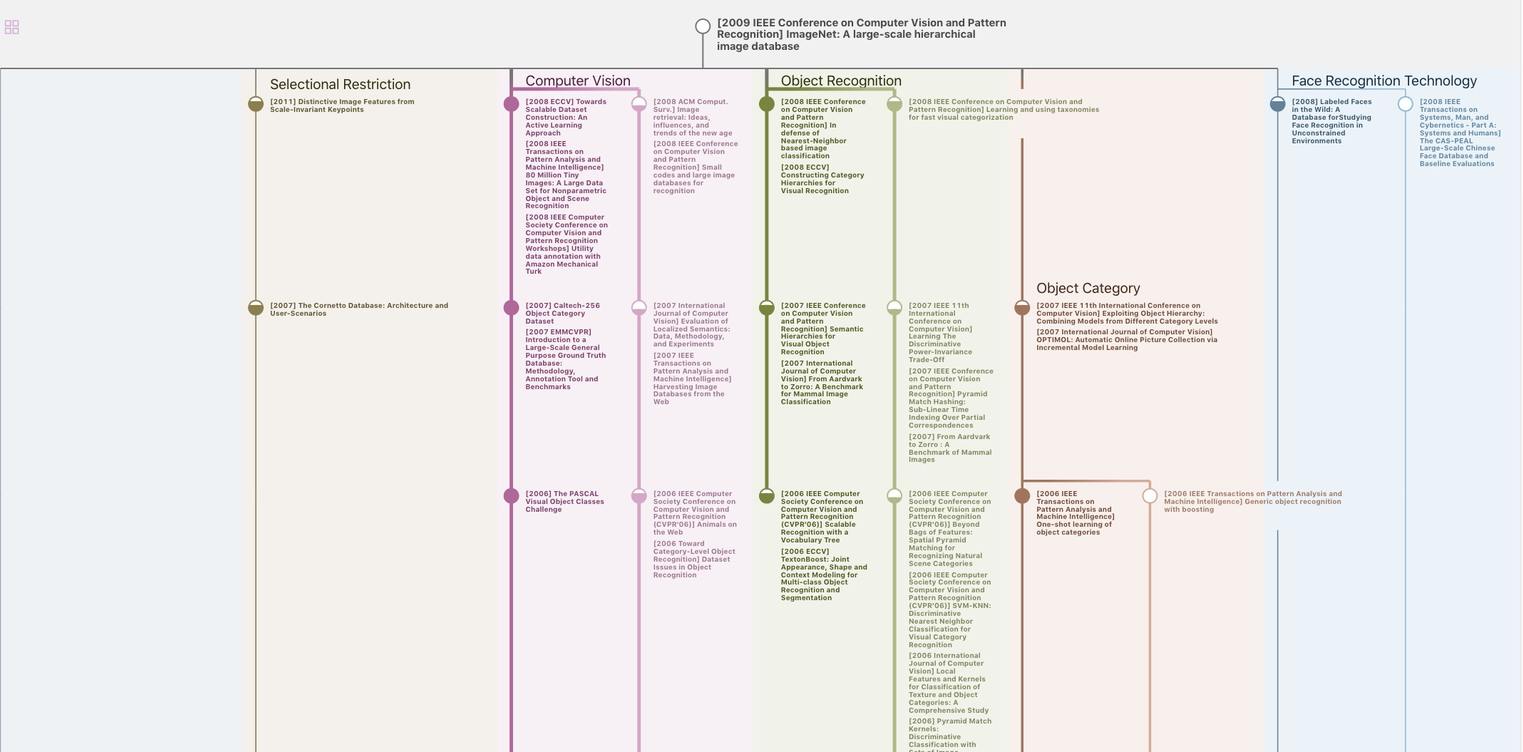
生成溯源树,研究论文发展脉络
Chat Paper
正在生成论文摘要