AINoC: New Interconnect for Future Deep Neural Network Accelerators
Design and Architecture for Signal and Image Processing(2023)
摘要
Data exchanges can be significant in the Deep Neural Network (DNN) algorithms. The interconnection between computing resources can quickly have a substantial impact on the overall performance of the architecture and its energy efficiency. Similarly, access to the different memories of the system, with potentially high data sharing, is a critical point. To overcome these problems, in this paper, we propose a new interconnect network, called AINoC, for future DNN accelerators, which require more flexibility and less power consumption to facilitate their integration into artificial intelligence (AI) edge systems. AINoC is based on (1) parallel routing that ensures communication/computation overlap to improve performance and (2) data reuse (filters, image inputs, and partial sums) to reduce multiple memory accesses. In the experiment section, AINoC can speedup LeNet5 convolution layers by up to 71.74
$$\times $$
in latency performance w.r.t. a RISC-V-based CPU and also speedup MobileNetV2 convolution layers by up to 2.35
$$\times $$
in latency performance w.r.t. a dataflow architecture featuring row-stationary execution style. AINoC provides any-to-any data exchange with wide interfaces (up to 51.2 GB/s) to support long bursts (up to 384 flits/cycle with packed data, i.e., 3 * 8-bit data in a 32-bit wide datapath) while executing LeNet5 and MobileNetV2. AINoC supports flexible communication with many multicast/broadcast requests with non-blocking transfers. Parallel communication in AINoC can provide up to 128
$$\times $$
more throughput (flits/cycle) and bandwidth (GB/s), using parallel routing with respect to single-path routing while executing convolution layers of LeNet5 and MobiletNetV2.
更多查看译文
关键词
new interconnect,neural network
AI 理解论文
溯源树
样例
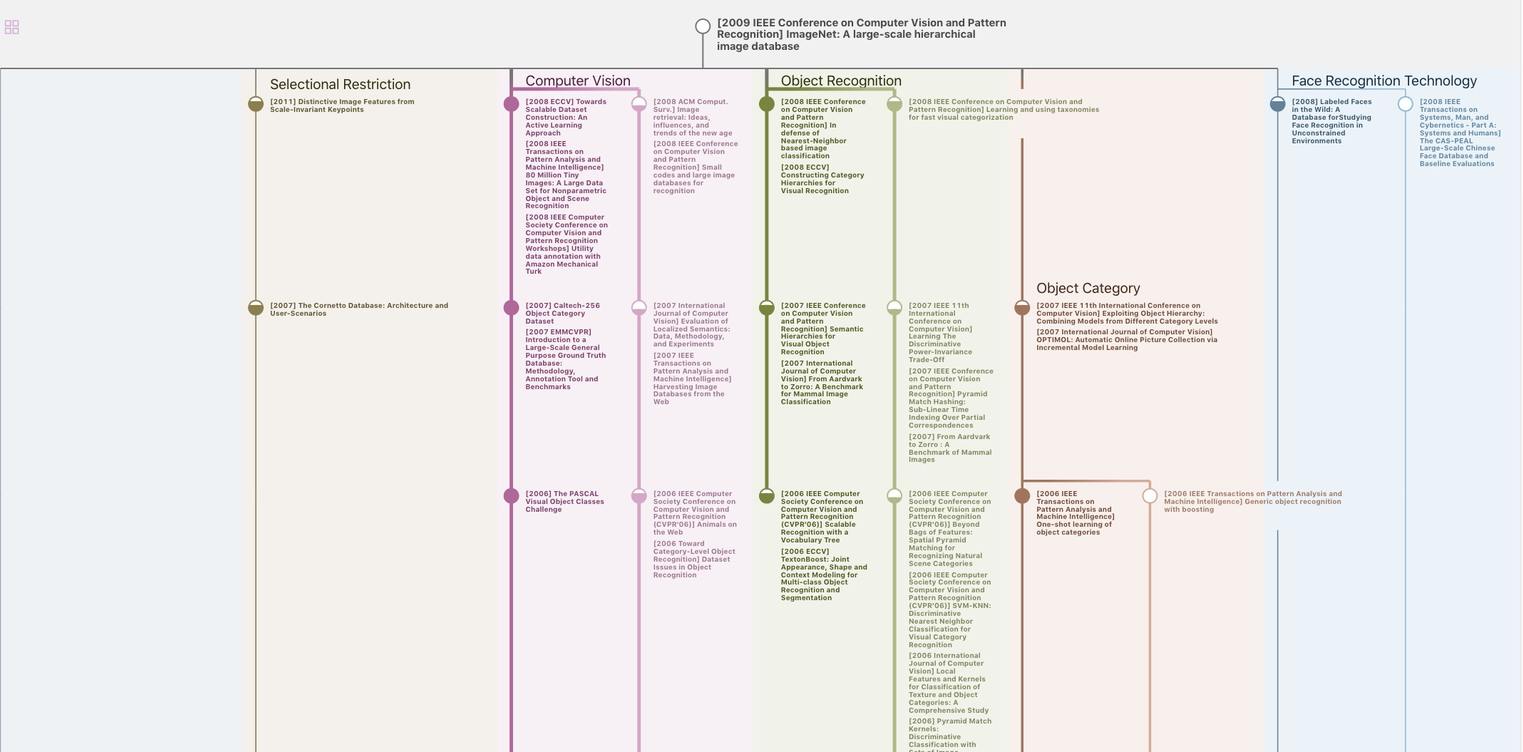
生成溯源树,研究论文发展脉络
Chat Paper
正在生成论文摘要