Study of Language Models for Fine-Grained Socio-Political Event Classification
Machine Learning and Computational Intelligence Techniques for Data Engineering(2023)
摘要
Since the introduction of inductive transfer learning methods and transformers models in natural language processing, we have witnessed a state of the art results in various tasks like machine translation, text summarization, image captioning, sentiment analysis, etc. The cornerstone of our study was to analyze various language modeling architectures and use various techniques for the task of event classification in order to classify short text snippets, which consist of news reportings of socio-political events with 25 fine-grained event types. We used four different fine-tuned language modeling architectures: RoBERTa, XLNet, ELMo, and BERT for our research. The corpus used for the model preparation was a re-sampled subset of the ACLED event dataset consisting of all 25 event sub-classes. Our best-performing model achieved an F1 score of 0.80 on the final unseen test set. For the overall result evaluation, we also conducted an error analysis of the consistently misclassified event types.
更多查看译文
关键词
Transfer learning, Transformer, Fine-Grained event classification, BERT, RoBERTa, XLNet, ELMo, ACLED
AI 理解论文
溯源树
样例
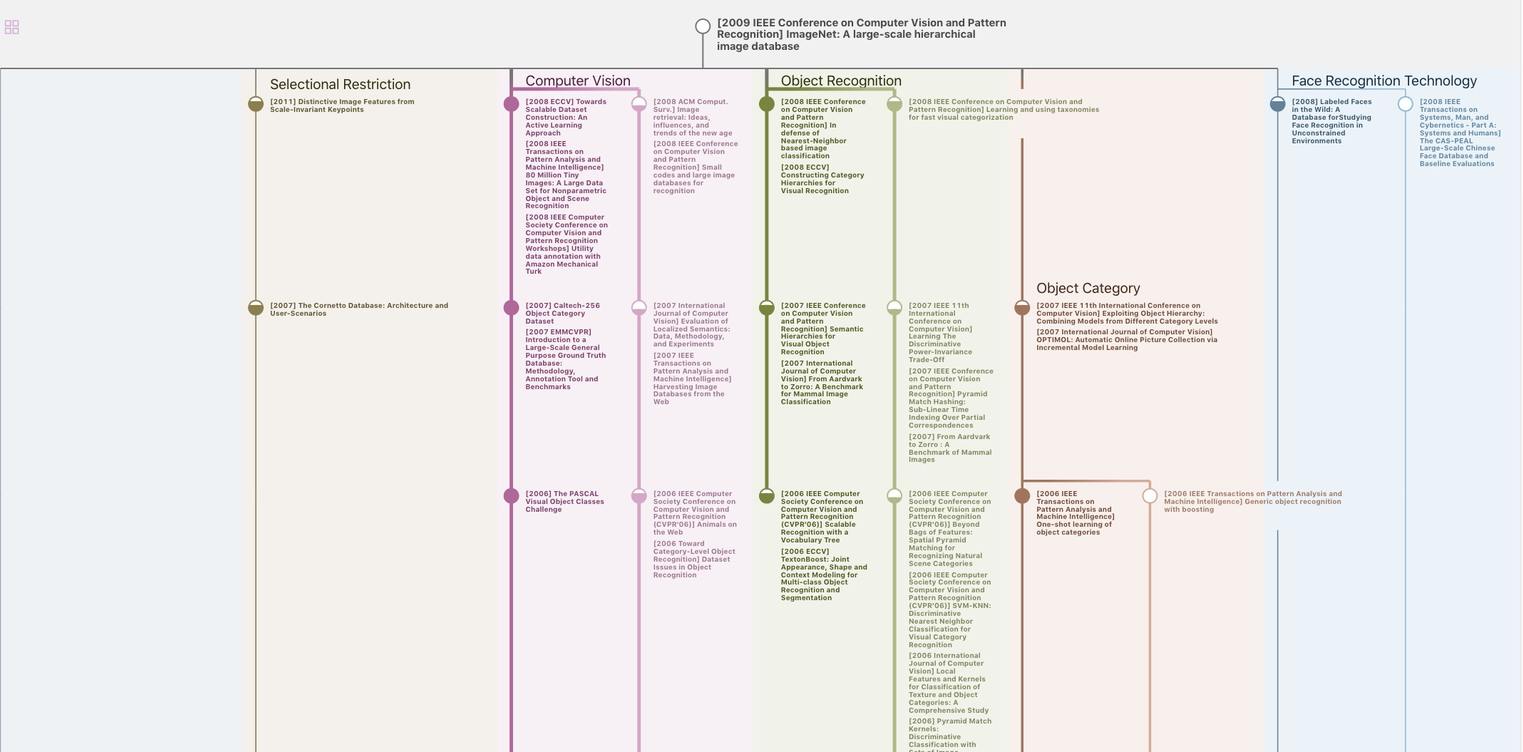
生成溯源树,研究论文发展脉络
Chat Paper
正在生成论文摘要