Machine Learning for Soft Sensors and an Application in Cement Production
Smart, Sustainable Manufacturing in an Ever-Changing World(2023)
摘要
Soft Sensors are predictors for measurements that are difficult or impossible to obtain by means of a physical sensor. A soft sensor delivers virtual measurements based on several or many physical measurements and a mathematical or numerical model that incorporates the physical knowledge about the interdependencies. In industrial processes, control, optimization, monitoring, and maintenance can benefit from the application of soft sensors. In recent years, Machine Learning (ML) has proven to be very effective for building soft sensors for industrial applications. We work on modelling dynamical processes for which the measurements of the physical variables are available as time series over long periods. In this article we present work on the comparison of different ML methods for modelling dynamic processes with the objective of predicting certain output variables. The main application focus of our work is on the optimization of the cement production process. We compare approaches using Recurrent Neural Networks (RNN) and Convolutional Neural Networks (CNN) using real process data from a cement production. The cement sector is the third-largest industrial energy consumer. There is a high potential for energy savings and for the reduction of CO2 emissions by optimizations of the production process. We present first results of our research that primarily is aimed to improve the robustness of a soft sensor for grain size in the cement production process.
更多查看译文
关键词
soft sensors,machine learning
AI 理解论文
溯源树
样例
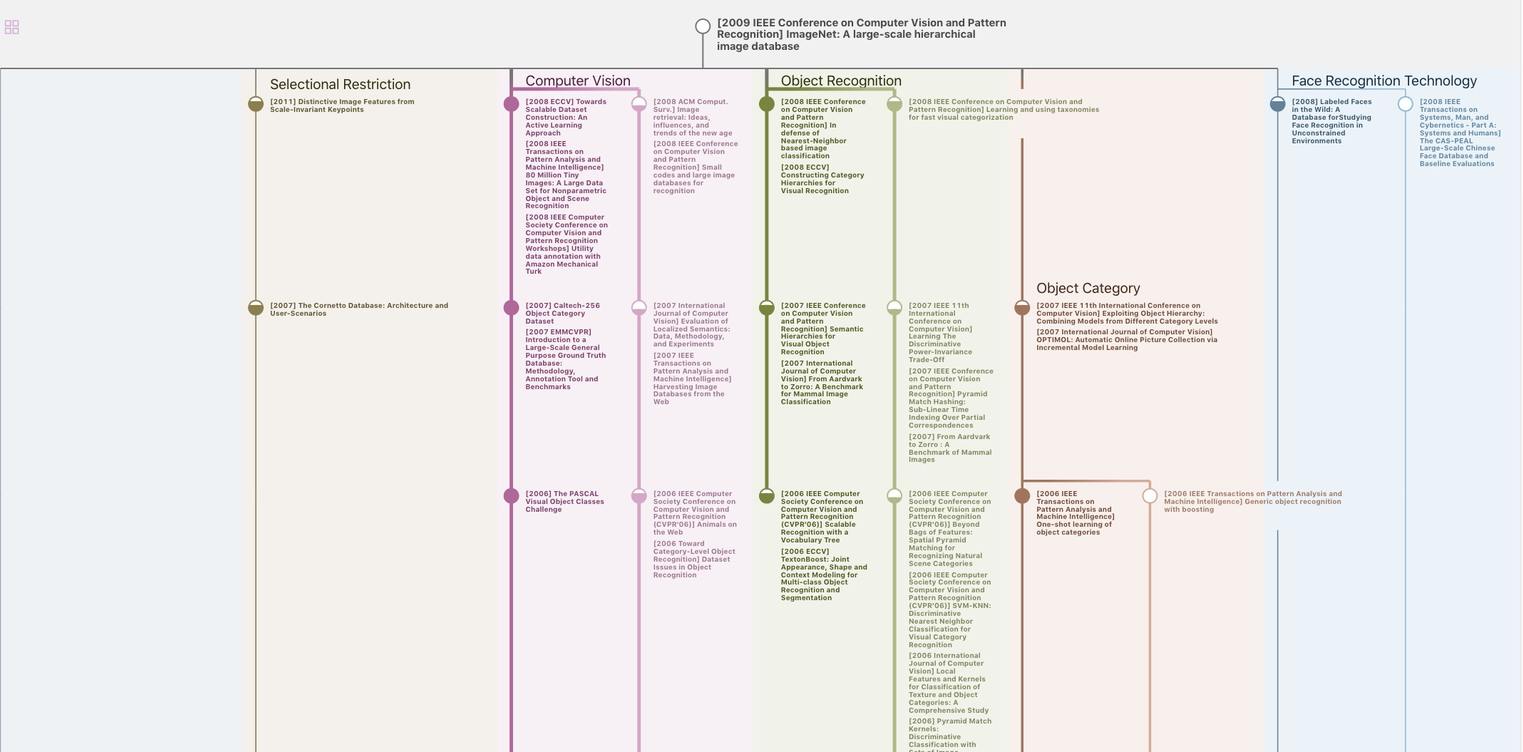
生成溯源树,研究论文发展脉络
Chat Paper
正在生成论文摘要