Evaluating Rotation Invariant Strategies for Mitosis Detection Through YOLO Algorithms
Wireless Mobile Communication and Healthcare(2023)
摘要
Cancer diagnosis is of major importance in the field of human medical pathology, wherein a cell division process known as mitosis constitutes a relevant biological pattern analyzed by professional experts, who seek for such occurrence in presence and number through visual observation of microscopic imagery. This is a time-consuming and exhausting task that can benefit from modern artificial intelligence approaches, namely those handling object detection through deep learning, from which YOLO can be highlighted as one of the most successful, and, as such, a good candidate for performing automatic mitoses detection. Considering that low sensibility for rotation/flip variations is of high importance to ensure mitosis deep detection robustness, in this work, we propose an offline augmentation procedure focusing rotation operations, to address the impact of lost/clipped mitoses induced by online augmentation. YOLOv4 and YOLOv5 were compared, using an augmented test dataset with an exhaustive set of rotation angles, to investigate their performance. YOLOv5 with a mixture of offline and online rotation augmentation methods presented the best averaged F1-score results over three runs.
更多查看译文
关键词
mitosis detection,evaluating rotation invariant strategies,algorithms
AI 理解论文
溯源树
样例
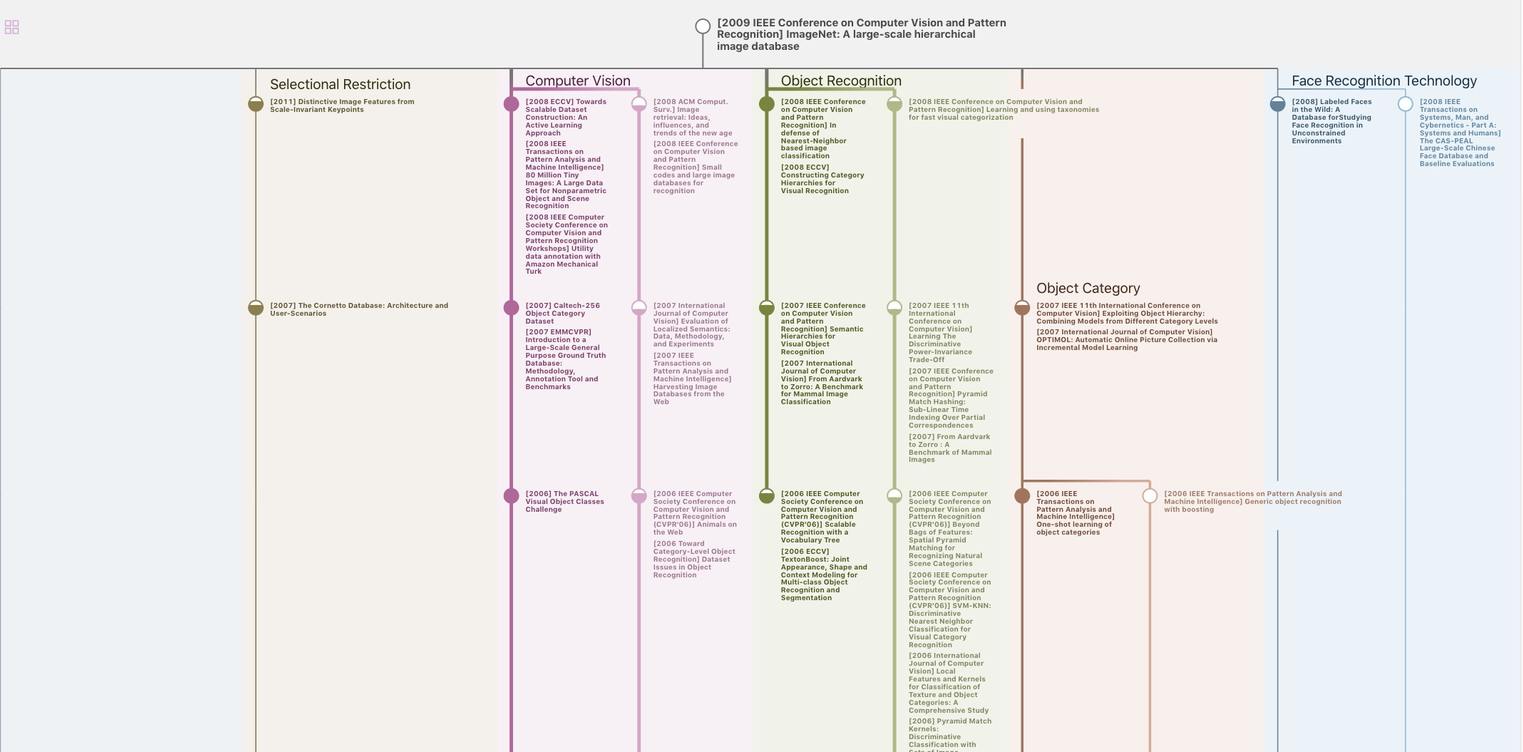
生成溯源树,研究论文发展脉络
Chat Paper
正在生成论文摘要