Community-Aware Centrality Measures Under the Independent Cascade Model
Complex Networks and Their Applications XI(2023)
摘要
Network topology, diffusion process, and node centrality are the key elements driving the diffusion dynamics in networks. Classical centrality measures do not exploit the community structure, although it is a ubiquitous property of natural and man-made real-world networks. Recent works have shown that community-aware centrality measures can be more effective. However, in their investigation, these works generally focus on popular diffusion processes such as the Susceptible-Infected-Recovered (SIR) and the Linear Threshold (LT) models on real-world networks. This work performs an extensive comparative analysis of eight popular community-aware centrality measures using the Independent Cascade (IC) model. Besides real-world networks, we also consider artificial networks with controlled properties to better understand the influence of the network topology in the diffusion process. Results show that targeting the nodes bridging the communities or highly inter-linked nodes results in a higher diffusion when a low fraction of nodes are initially involved in the diffusion process. In contrast, when the initial budget of nodes is high, it is more effective to target distant hubs as initial spreaders. Moreover, setting a uniform threshold and a weak community structure strength hinders the diffusive power of the community-aware centrality measures.
更多查看译文
AI 理解论文
溯源树
样例
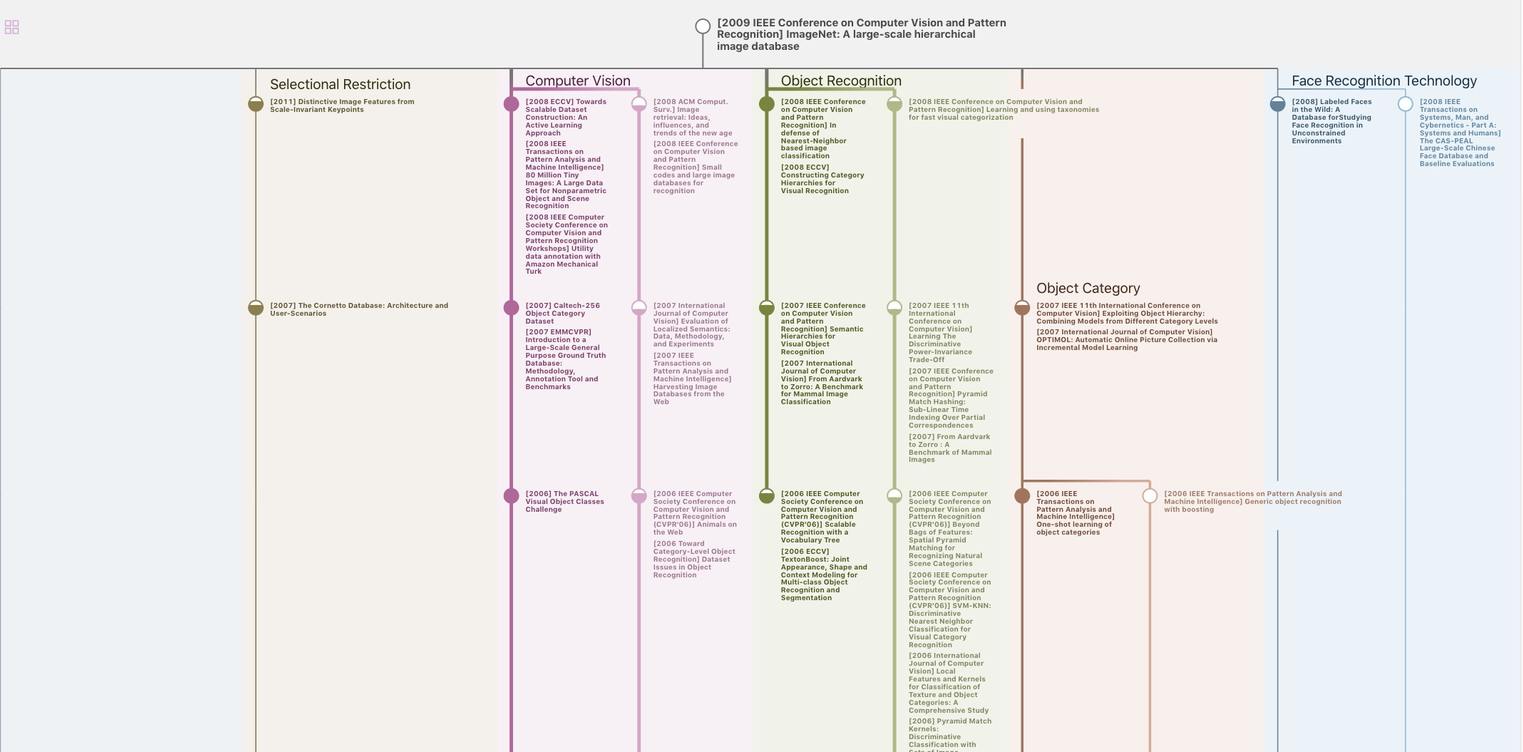
生成溯源树,研究论文发展脉络
Chat Paper
正在生成论文摘要