Hiatus: Unsupervised Generative Approach for Detection of DoS and DDoS Attacks
Security and Privacy in Communication Networks(2023)
摘要
Denial of Service (DoS) and Distributed Denial of Service (DDoS) attacks pose a serious threat to the internet community by disrupting the availability of services. The current methods for detecting DoS and DDoS attacks have several drawbacks including a high false-positive rate and are mostly supervised techniques. The datasets used lack recent attack types. To overcome these limitations, we propose Hiatus: two independent generative models as anomaly detectors: (1) Variational Auto Encoder (VAE), and (2) Generative Adversarial Network (GAN) to classify the traffic flow as either benign or DoS or DDoS. We make the following contributions: (1) two learning algorithms (VAE and GAN) are trained in an unsupervised fashion to detect DoS and DDoS traffic without the involvement of labeled data, (2) avoid external feature engineering, (3) both the learning algorithms are trained and tested on CICDDoS2019 dataset which consists of latest exploitation and reflection based attacks, and the models are benchmarked by testing them with CICIDS2017 and UNSW-NB15 dataset. With the evaluated results, the proposed approaches outperform existing state-of-the-art techniques and could be used for effective DoS and DDoS detection.
更多查看译文
关键词
unsupervised generative approach,dos,detection
AI 理解论文
溯源树
样例
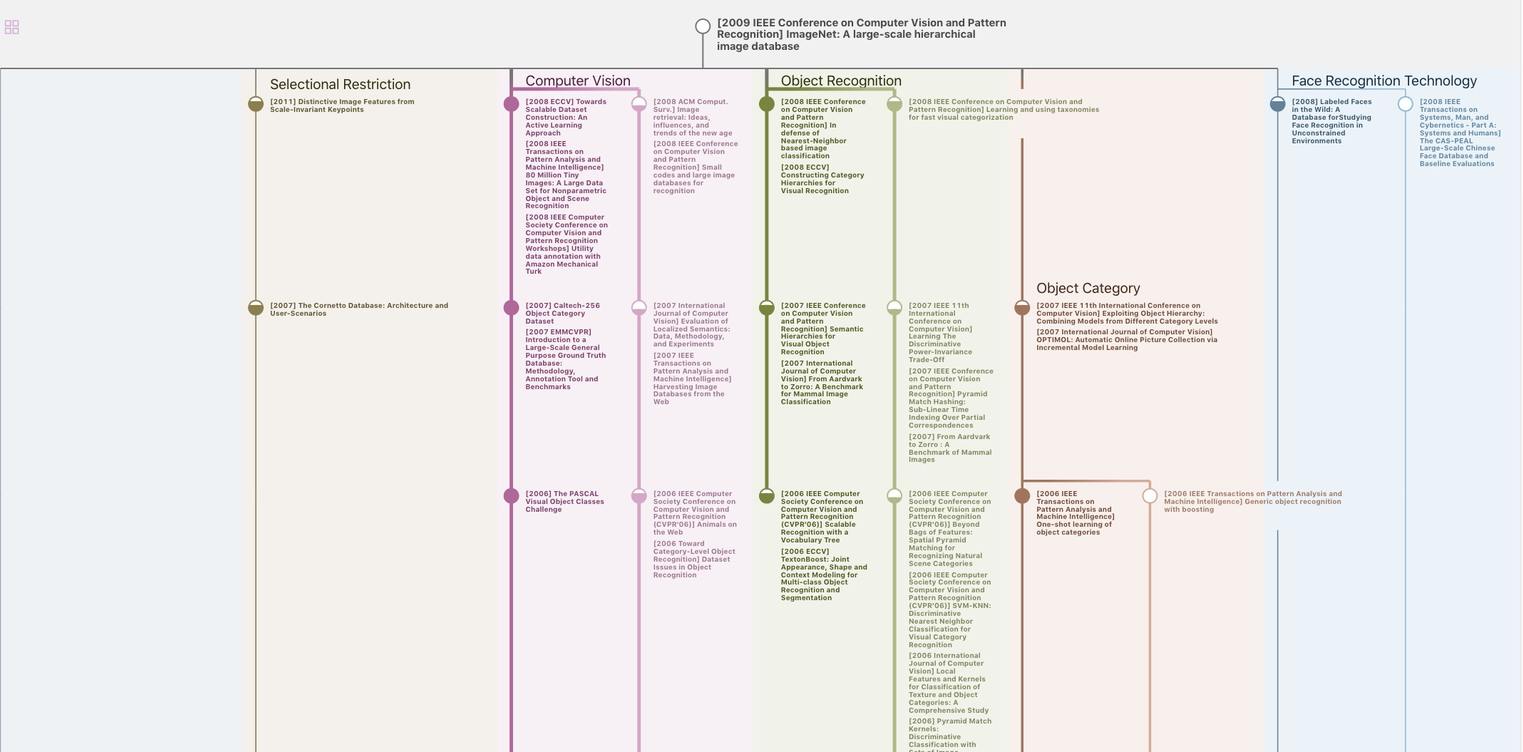
生成溯源树,研究论文发展脉络
Chat Paper
正在生成论文摘要