Evaluating multi-model and ensemble forecasts in the context of the Mpox outbreak in multiple countries, July 28th, 2022, through January 19th, 2023
medrxiv(2023)
摘要
In May 2022, public health officials noted an unprecedented surge in Mpox cases in non-endemic countries worldwide. As the epidemic accelerated, multi-model forecasts of the epidemic's trajectory were critical in guiding the implementation of public health interventions, and in determining policy. As the case levels have significantly decreased as of mid-August, evaluating model performance is essential to advance the growing field of epidemic forecasting. Using laboratory-confirmed Mpox case data from the CDC and OWID teams through the week of January 26th, 2023, we generated retrospective sequential weekly forecasts (e.g., 1-week, 2-week, 3-week, and 4-week) for Brazil, Canada, England, France, Germany, Spain, the USA, and at the global scale using models that require minimal input data including the auto-regressive integrated moving average (ARIMA), general additive model (GAM), simple linear regression (SLR) as well as the spatial-wave and n-sub-epidemic modeling frameworks. Forecast performance was assessed using MSE, MAE, WIS, 95% PI coverage metrics as well as skill scores. Overall, the spatial-wave sub-epidemic modeling framework outcompeted other models across most locations and forecasting horizons in terms of the average MSE, MAE, and WIS. It was followed closely in success by the n-sub-epidemic top-ranked and weighted ensemble (2) models. Regarding average 95% PI coverage, the n-sub-epidemic unweighted (equally weighted top-models) ensemble (2) model performed best across all forecasting horizons for most locations. However, many locations had multiple models performing equally well in terms of the average 95% PI coverage. Both the n-sub-epidemic and spatial-wave frameworks improved considerably in average MSE, MAE, and WIS, and minimally (<10%) in average 95% PI coverage relative to the ARIMA model. Findings lend further support to sub-epidemic models for short-term forecasting epidemics of emerging and re-emerging infectious diseases.
### Competing Interest Statement
The authors have declared no competing interest.
### Funding Statement
A.B. is supported by a 2CI fellowship from Georgia State University. G.C. is partially supported from NSF grants 1610429 and 1633381 and R01 GM 130900. The funders had no role in study design, data collection and analysis, decision to publish, or preparation of the manuscript.
### Author Declarations
I confirm all relevant ethical guidelines have been followed, and any necessary IRB and/or ethics committee approvals have been obtained.
Yes
I confirm that all necessary patient/participant consent has been obtained and the appropriate institutional forms have been archived, and that any patient/participant/sample identifiers included were not known to anyone (e.g., hospital staff, patients or participants themselves) outside the research group so cannot be used to identify individuals.
Yes
I understand that all clinical trials and any other prospective interventional studies must be registered with an ICMJE-approved registry, such as ClinicalTrials.gov. I confirm that any such study reported in the manuscript has been registered and the trial registration ID is provided (note: if posting a prospective study registered retrospectively, please provide a statement in the trial ID field explaining why the study was not registered in advance).
Yes
I have followed all appropriate research reporting guidelines, such as any relevant EQUATOR Network research reporting checklist(s) and other pertinent material, if applicable.
Yes
All original data sources are stated within the paper. The source code and forecasts used to produce results for GAM, SLR, and ARIMA are available upon request to the authors. Source code for producing and evaluating the ensemble n-sub-epidemic forecasts is publicly available at: https://github.com/gchowell/ensemble\_n-subepidemic\_framework. Source code for producing and evaluating spatial-wave forecasts is publicly available at: https://github.com/gchowell/spatial\_wave\_subepidemic_framework.
[https://github.com/gchowell/ensemble\_n-subepidemic\_framework][1]
[https://github.com/gchowell/spatial\_wave\_subepidemic_framework][2]
[1]: https://github.com/gchowell/ensemble_n-subepidemic_framework
[2]: https://github.com/gchowell/spatial_wave_subepidemic_framework
更多查看译文
AI 理解论文
溯源树
样例
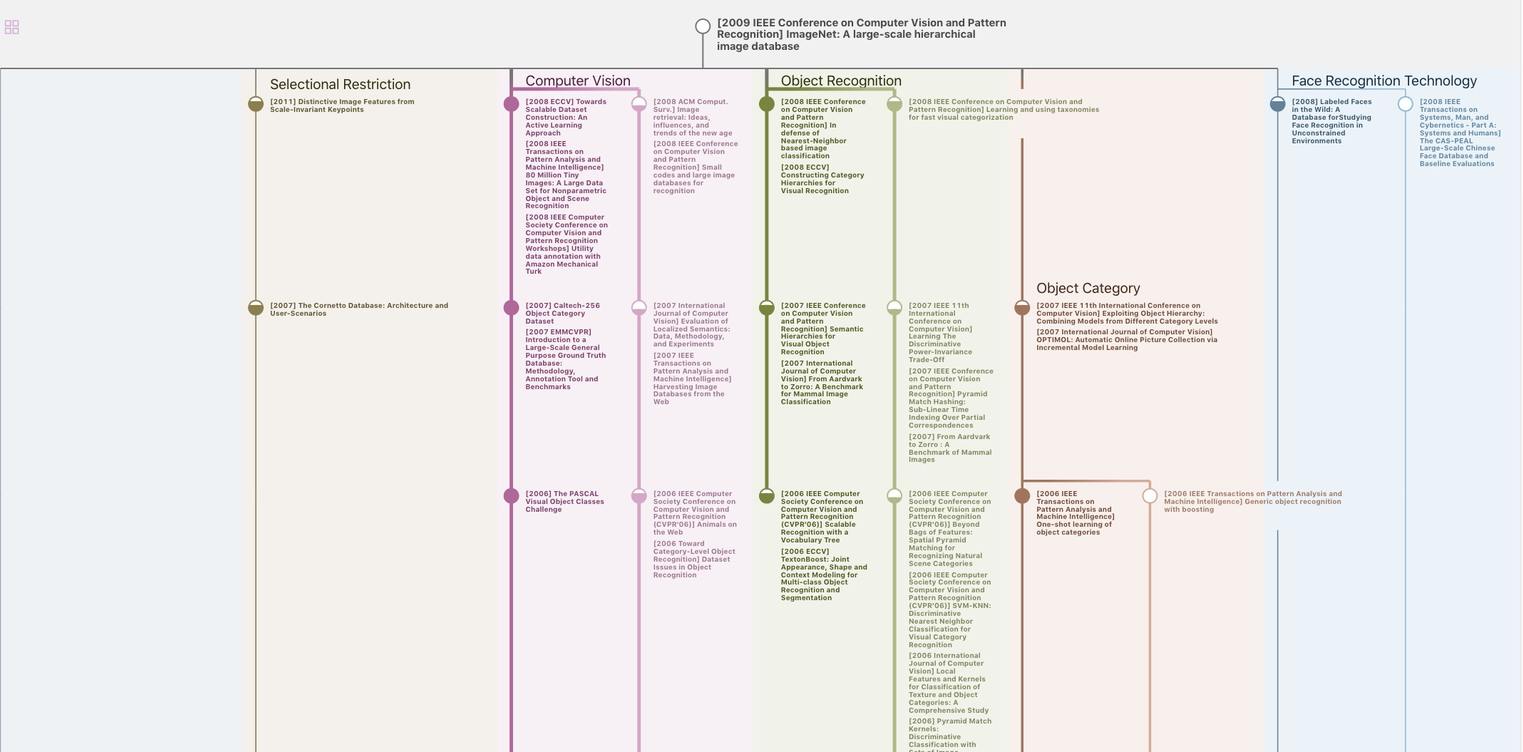
生成溯源树,研究论文发展脉络
Chat Paper
正在生成论文摘要