Rapid Adaptation in Online Continual Learning: Are We Evaluating It Right?
CoRR(2023)
摘要
We revisit the common practice of evaluating adaptation of Online Continual Learning (OCL) algorithms through the metric of online accuracy, which measures the accuracy of the model on the immediate next few samples. However, we show that this metric is unreliable, as even vacuous blind classifiers, which do not use input images for prediction, can achieve unrealistically high online accuracy by exploiting spurious label correlations in the data stream. Our study reveals that existing OCL algorithms can also achieve high online accuracy, but perform poorly in retaining useful information, suggesting that they unintentionally learn spurious label correlations. To address this issue, we propose a novel metric for measuring adaptation based on the accuracy on the near-future samples, where spurious correlations are removed. We benchmark existing OCL approaches using our proposed metric on large-scale datasets under various computational budgets and find that better generalization can be achieved by retaining and reusing past seen information. We believe that our proposed metric can aid in the development of truly adaptive OCL methods. We provide code to reproduce our results at https://github.com/drimpossible/EvalOCL.
更多查看译文
关键词
online continual learning,adaptation
AI 理解论文
溯源树
样例
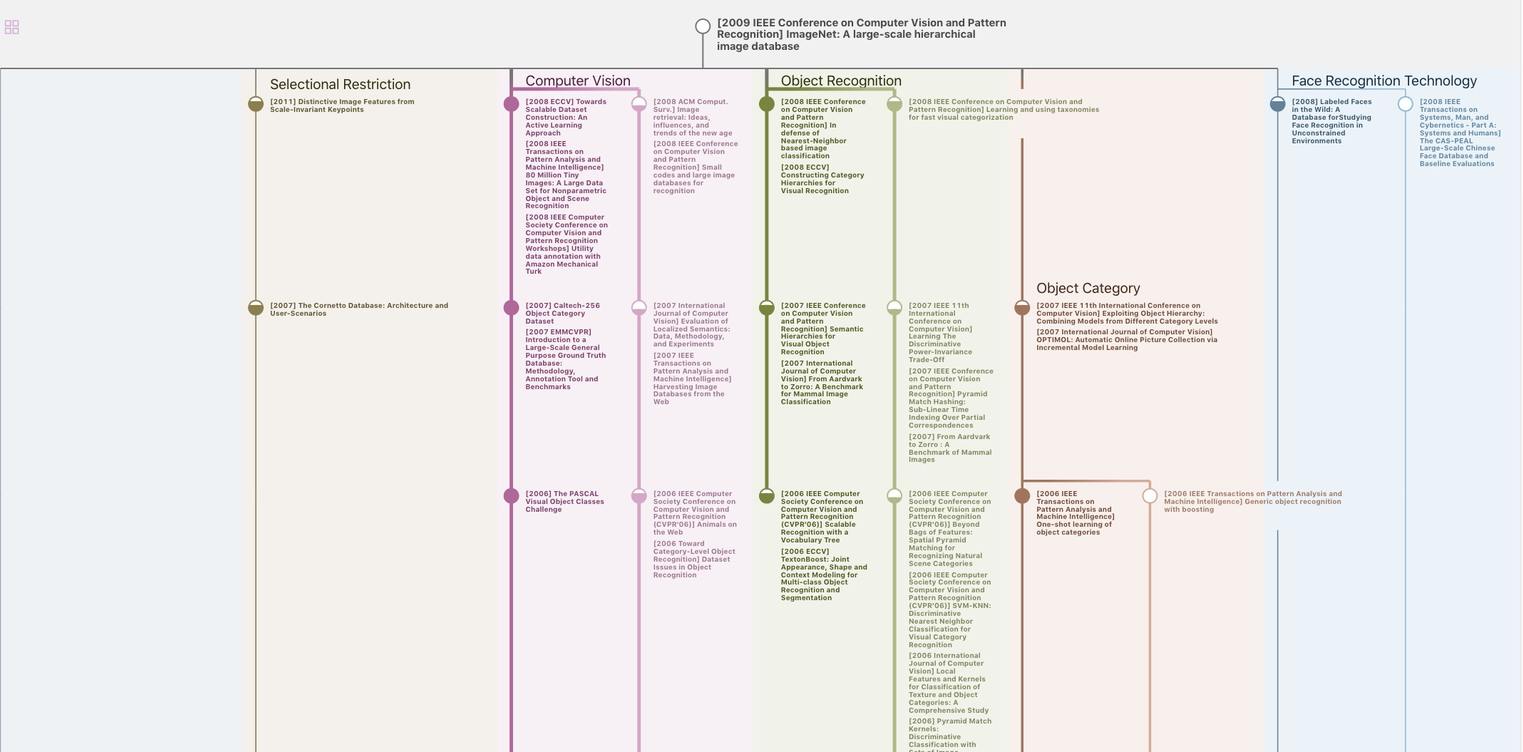
生成溯源树,研究论文发展脉络
Chat Paper
正在生成论文摘要