A tight bound of modified iterative hard thresholding algorithm for compressed sensing
Applications of Mathematics(2023)
摘要
We provide a theoretical study of the iterative hard thresholding with partially known support set (IHT-PKS) algorithm when used to solve the compressed sensing recovery problem. Recent work has shown that IHT-PKS performs better than the traditional IHT in reconstructing sparse or compressible signals. However, less work has been done on analyzing the performance guarantees of IHT-PKS. In this paper, we improve the current RIP-based bound of IHT-PKS algorithm from δ _3s - 2k < 1 √(32)≈ 0.1768 to δ _3s - 2k < √(5) - 1 4 , where δ 3 s −2 k is the restricted isometric constant of the measurement matrix. We also present the conditions for stable reconstruction using the IHT μ -PKS algorithm which is a general form of IHT-PKS. We further apply the algorithm on Least Squares Support Vector Machines (LS-SVM), which is one of the most popular tools for regression and classification learning but confronts the loss of sparsity problem. After the sparse representation of LS-SVM is presented by compressed sensing, we exploit the support of bias term in the LS-SVM model with the IHT μ -PKS algorithm. Experimental results on classification problems show that IHT μ -PKS outperforms other approaches to computing the sparse LS-SVM classifier.
更多查看译文
关键词
sensing,algorithm
AI 理解论文
溯源树
样例
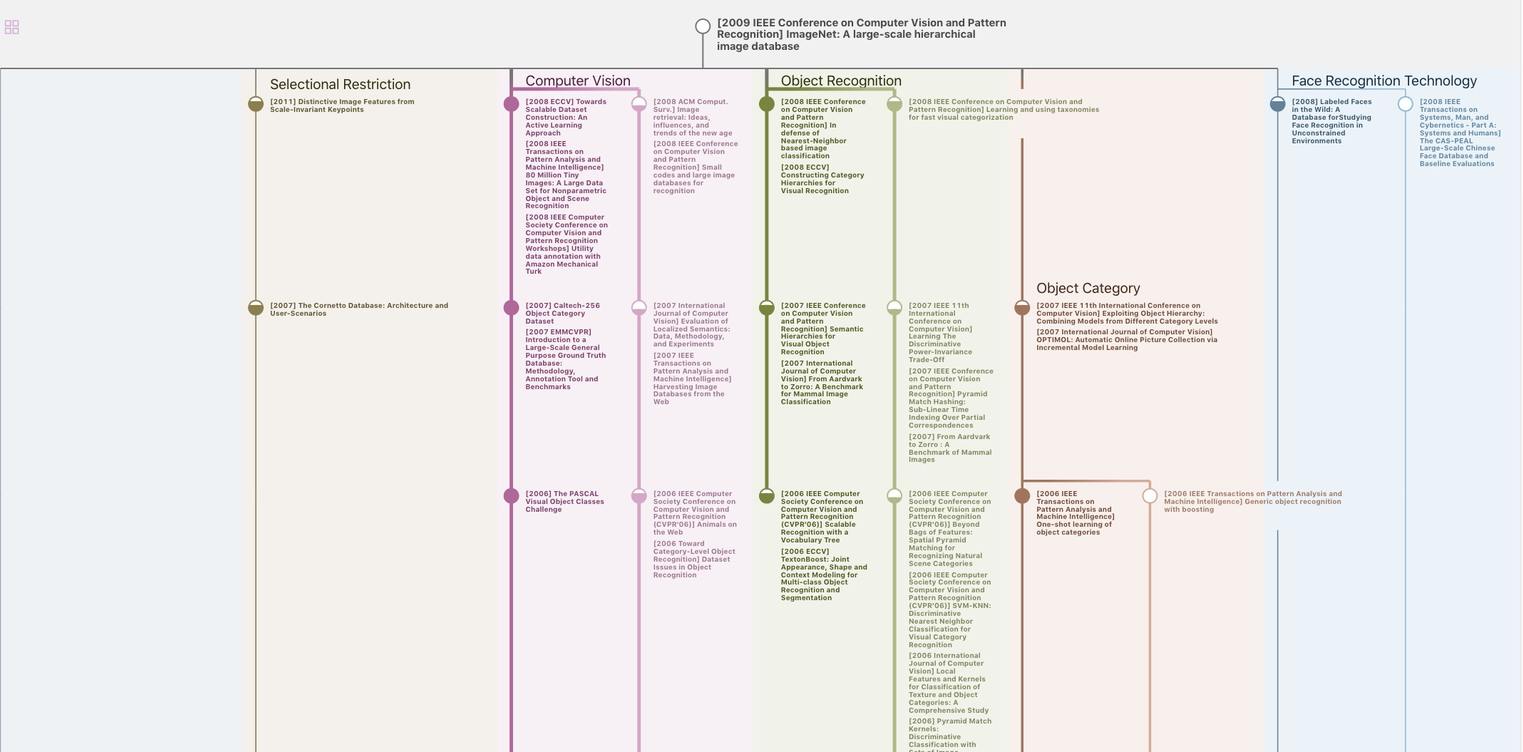
生成溯源树,研究论文发展脉络
Chat Paper
正在生成论文摘要