Kernel-based Early Fusion of Structure and Attribute Information for Detecting Communities in Attributed Networks
Artificial Life and Evolutionary Computation(2023)
摘要
Early fusion methods are a category of community detection methods for attributed networks that merge attributes and structure before the method is executed. Typically, a weighted network where the edge between two nodes includes both the structure information (i.e. the weight of the existing link) and the similarity of the attributes is obtained and then, classical community detection algorithms can be applied. In this paper, we investigate the role of kernels on a method based on a Genetic Algoritm (GA). When measuring similarity, kernels are able to provide a more suitable and meaningful form for the similarity matrix in order to facilitate data analysis. Through simulations on both synthetic and real-world attributed networks, we first apply different kernels to @NetGA, a genetic algorithm we proposed for attributed networks which embeds into edges both the structure and the attribute information through a unified distance measure. We compare this novel approach named K@NetGA to @NetGA showing that the Free-Energy kernel is able to sensibly improve the community detection performance. We finally compare our method to other kernel-based community detection methods on a wider set of networks finding that K@NetGA is the best-performing.
更多查看译文
关键词
detecting communities,networks,attribute information,kernel-based
AI 理解论文
溯源树
样例
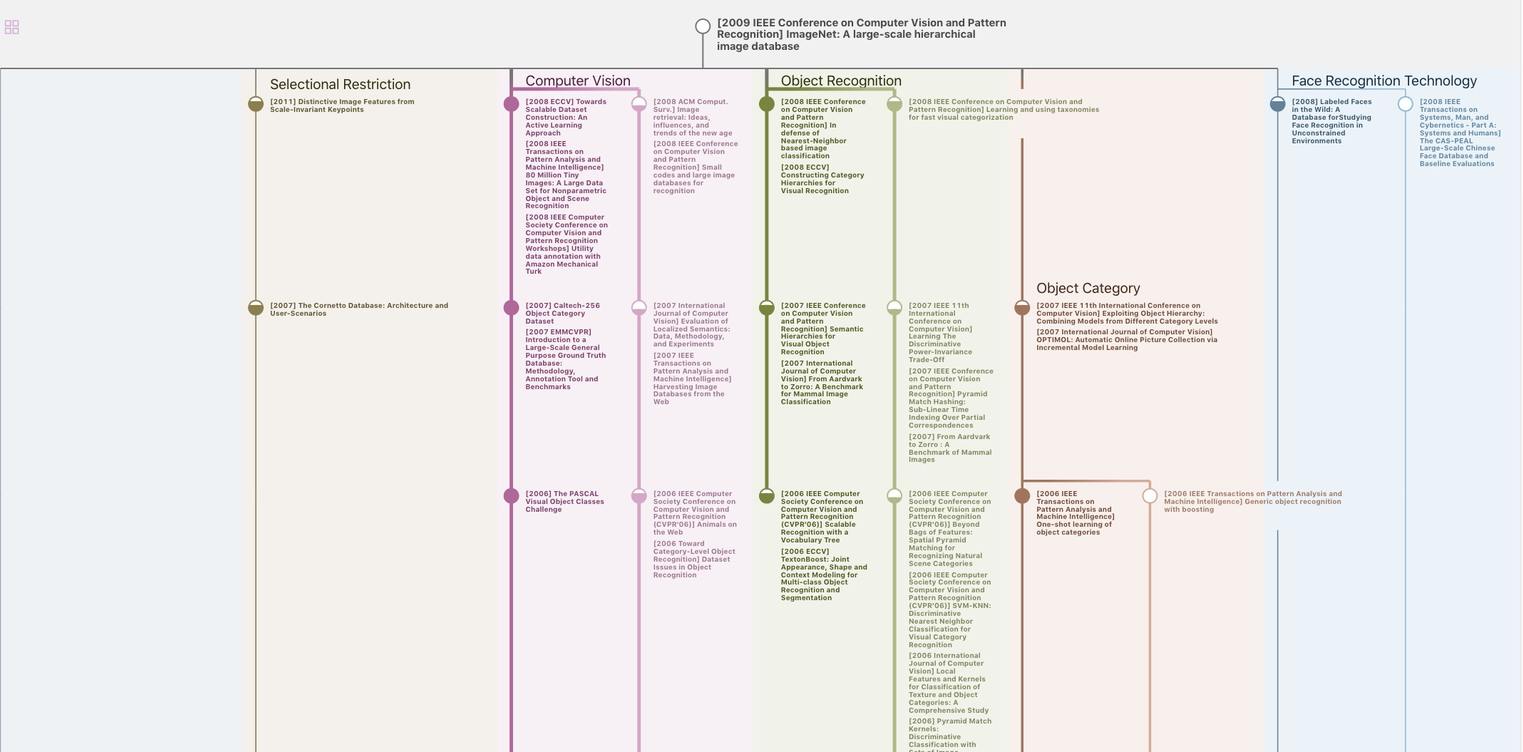
生成溯源树,研究论文发展脉络
Chat Paper
正在生成论文摘要