RepCL: Exploring Effective Representation for Continual Text Classification
CoRR(2023)
摘要
Continual learning (CL) aims to constantly learn new knowledge over time while avoiding catastrophic forgetting on old tasks. In this work, we focus on continual text classification under the class-incremental setting. Recent CL studies find that the representations learned in one task may not be effective for other tasks, namely representation bias problem. For the first time we formally analyze representation bias from an information bottleneck perspective and suggest that exploiting representations with more class-relevant information could alleviate the bias. To this end, we propose a novel replay-based continual text classification method, RepCL. Our approach utilizes contrastive and generative representation learning objectives to capture more class-relevant features. In addition, RepCL introduces an adversarial replay strategy to alleviate the overfitting problem of replay. Experiments demonstrate that RepCL effectively alleviates forgetting and achieves state-of-the-art performance on three text classification tasks.
更多查看译文
关键词
continual text
AI 理解论文
溯源树
样例
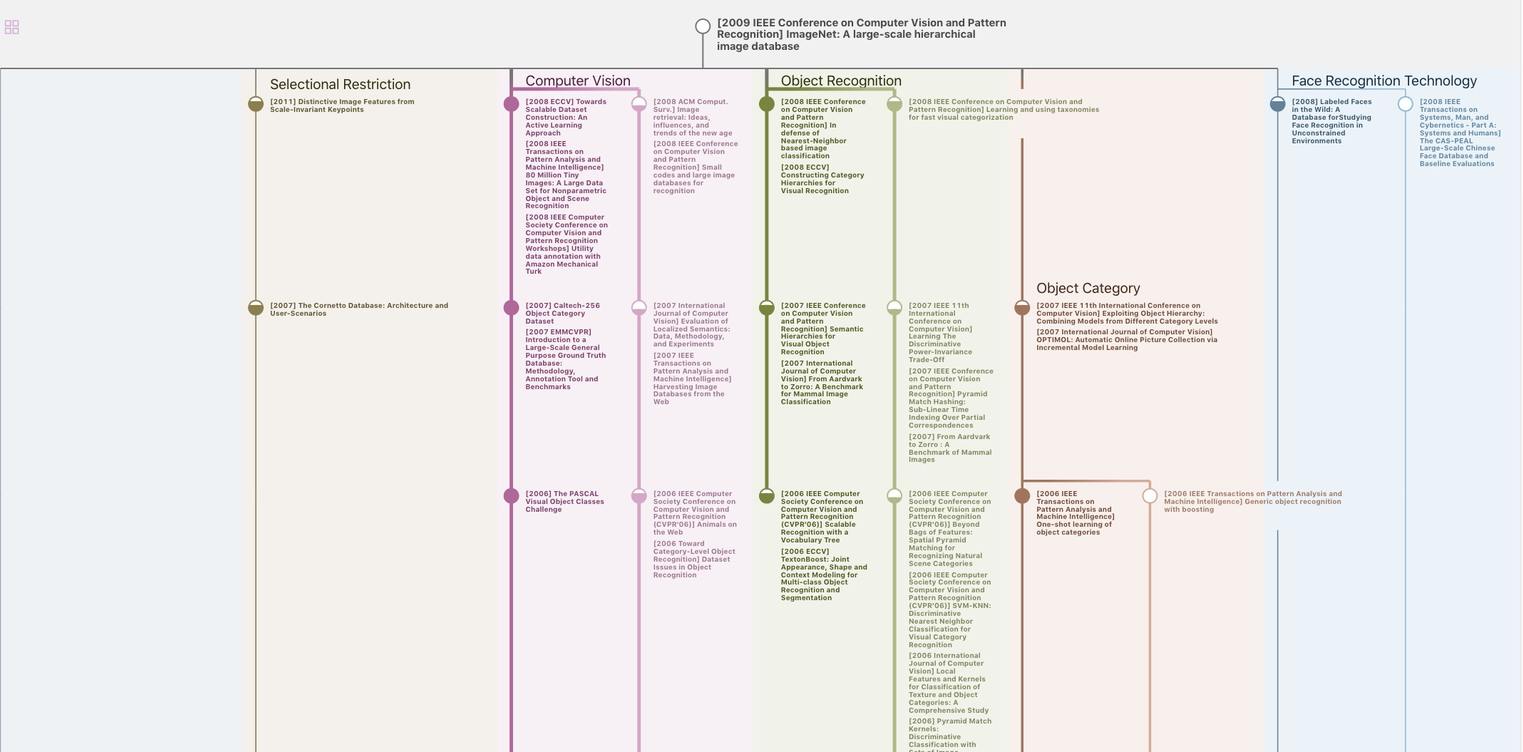
生成溯源树,研究论文发展脉络
Chat Paper
正在生成论文摘要