Unsupervised Noise Reductions for Gravitational Reference Sensors or Accelerometers Based on the Noise2Noise Method
Sensors (Basel, Switzerland)(2023)
摘要
Onboard electrostatic suspension inertial sensors are important applications for gravity satellites and space gravitational-wave detection missions, and it is important to suppress noise in the measurement signal. Due to the complex coupling between the working space environment and the satellite platform, the process of noise generation is extremely complex, and traditional noise modeling and subtraction methods have certain limitations. With the development of deep learning, applying it to high-precision inertial sensors to improve the signal-to-noise ratio is a practically meaningful task. Since there is a single noise sample and unknown true value in the measured data in orbit, odd-even sub-samplers and periodic sub-samplers are designed to process general signals and periodic signals, and adds reconstruction layers consisting of fully connected layers to the model. Experimental analysis and comparison are conducted based on simulation data, GRACE-FO acceleration data, and Taiji-1 acceleration data. The results show that the deep learning method is superior to traditional data smoothing processing solutions.
更多查看译文
关键词
Noise2Noise,deep learning,denoising,accelerometer,inertial sensor
AI 理解论文
溯源树
样例
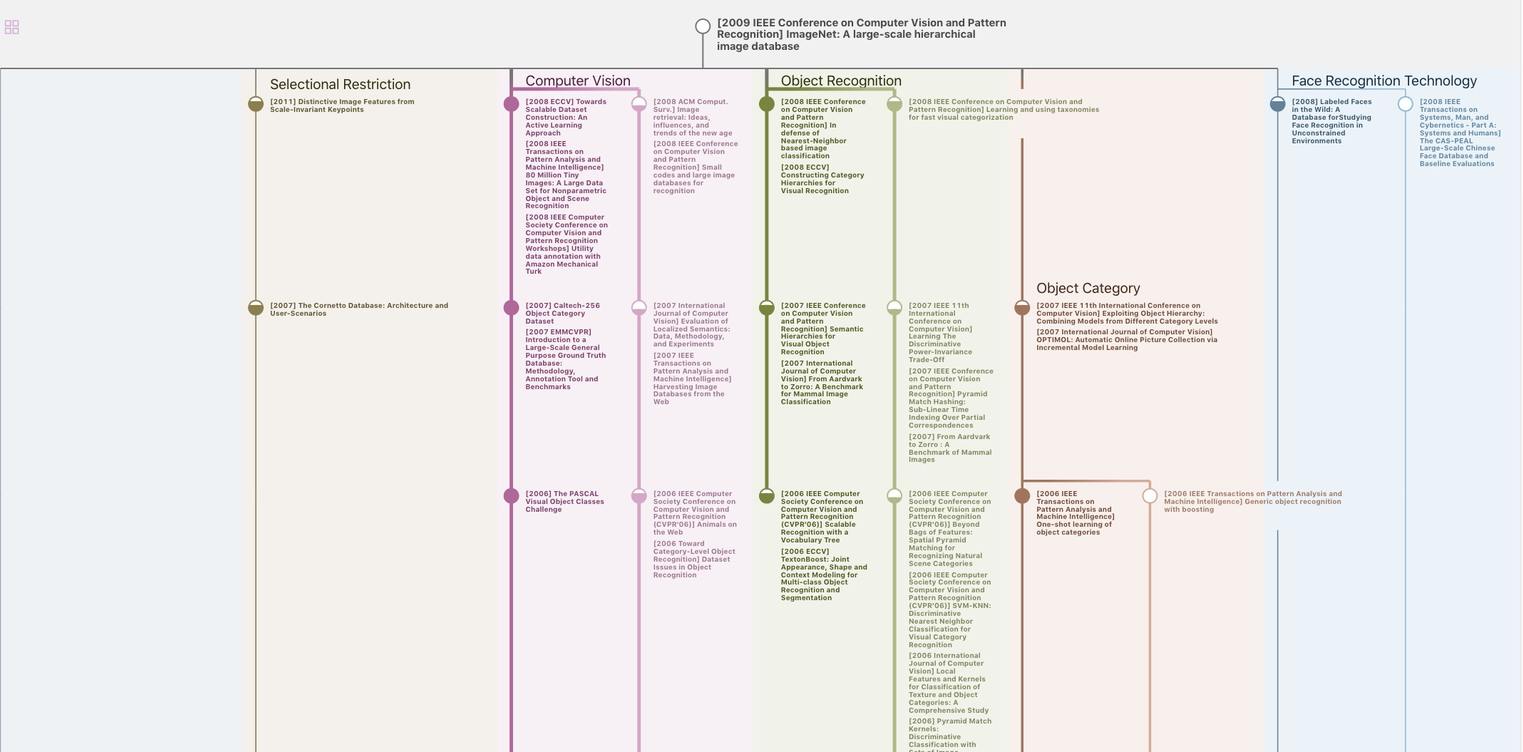
生成溯源树,研究论文发展脉络
Chat Paper
正在生成论文摘要