Backpropagation-free 4D continuous ant-based neural topology search
CoRR(2023)
摘要
Continuous Ant-based Topology Search (CANTS) is a previously introduced novel nature-inspired neural architecture search (NAS) algorithm that is based on ant colony optimization (ACO). CANTS utilizes a continuous search space to indirectly-encode a neural architecture search space. Synthetic ant agents explore CANTS' continuous search space based on the density and distribution of pheromones, strongly inspired by how ants move in the real world. This continuous search space allows CANTS to automate the design of artificial neural networks (ANNs) of any size, removing a key limitation inherent to many current NAS algorithms that must operate within structures of a size that is predetermined by the user. This work expands CANTS by adding a fourth dimension to its search space representing potential neural synaptic weights. Adding this extra dimension allows CANTS agents to optimize both the architecture as well as the weights of an ANN without applying backpropagation (BP), which leads to a significant reduction in the time consumed in the optimization process: at least an average of 96% less time consumption with very competitive optimization performance, if not better. The experiments of this study - using real-world data - demonstrate that the BP-Free CANIS algorithm exhibits highly competitive performance compared to both CANTS and ANTS while requiring significantly less operation time.(c) 2023 Elsevier B.V. All rights reserved.
更多查看译文
关键词
NeuroEvolution,Neural architecture search,Swarm intelligence,Genetic evolution,Ant colony optimization,Time-series prediction
AI 理解论文
溯源树
样例
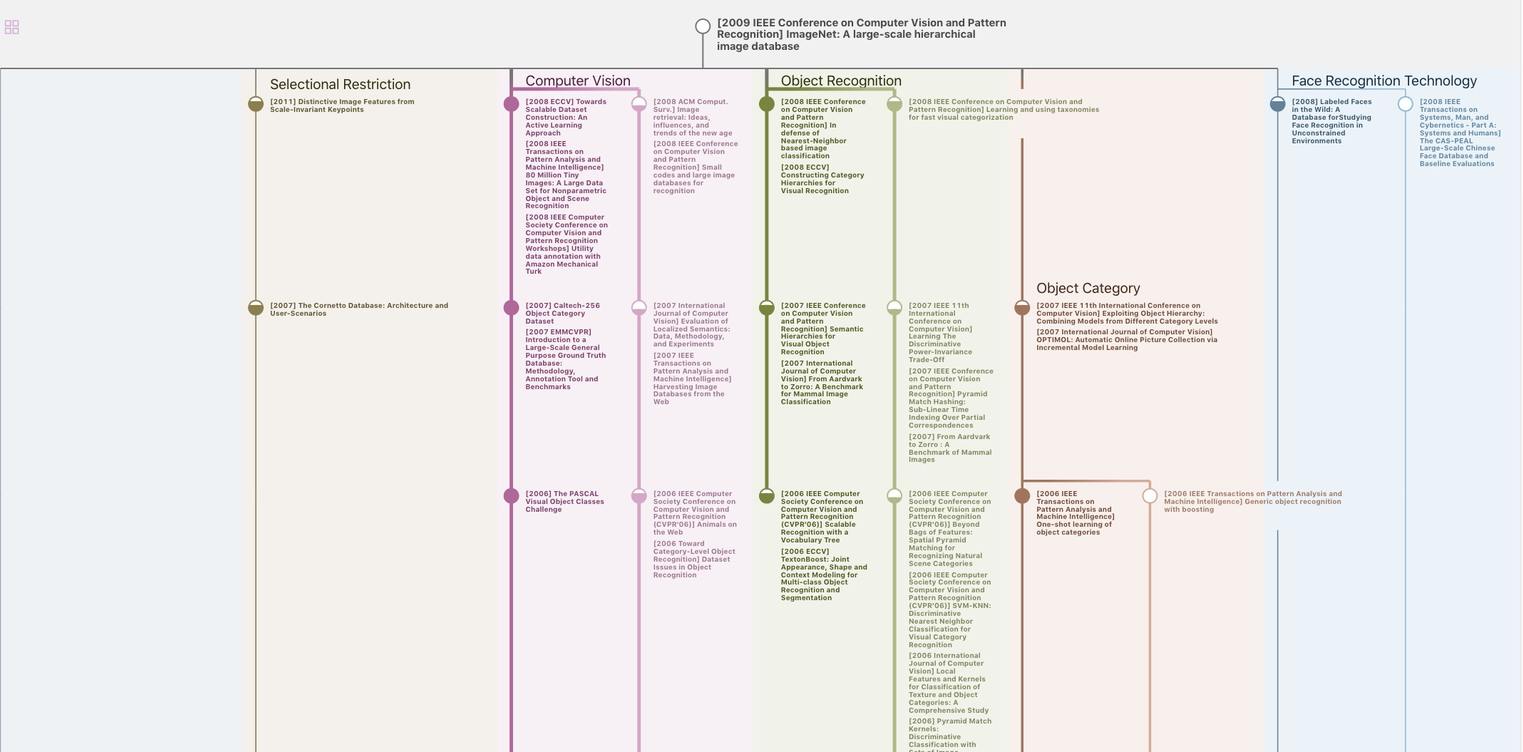
生成溯源树,研究论文发展脉络
Chat Paper
正在生成论文摘要