Common Information Dimension
CoRR(2023)
摘要
The exact common information between a set of random variables $X_1,...,X_n$ is defined as the minimum entropy of a shared random variable that allows for the exact distributive simulation of $X_1,...,X_n$. It has been established that, in certain instances, infinite entropy is required to achieve distributive simulation, suggesting that continuous random variables may be needed in such scenarios. However, to date, there is no established metric to characterize such cases. In this paper, we propose the concept of Common Information Dimension (CID) with respect to a given class of functions $\mathcal{F}$, defined as the minimum dimension of a random variable $W$ required to distributively simulate a set of random variables $X_1,...,X_n$, such that $W$ can be expressed as a function of $X_1,\cdots,X_n$ using a member of $\mathcal{F}$. Our main contributions include the computation of the common information dimension for jointly Gaussian random vectors in a closed form, with $\mathcal{F}$ being the linear functions class.
更多查看译文
关键词
common information dimension,continuous random variables,exact common information,exact distributive simulation,infinite entropy,jointly Gaussian random vectors,minimum dimension,minimum entropy
AI 理解论文
溯源树
样例
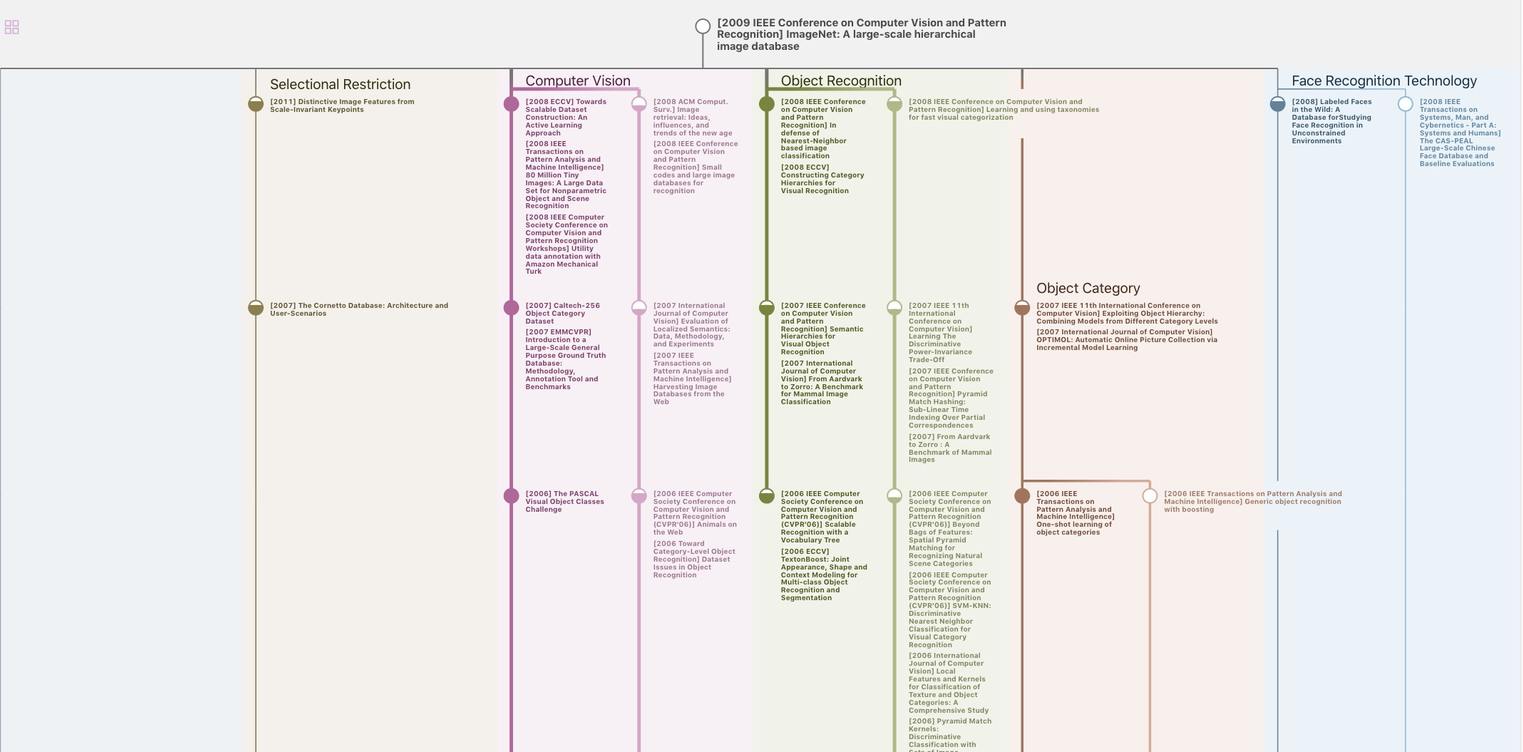
生成溯源树,研究论文发展脉络
Chat Paper
正在生成论文摘要