Efficient hyperparameters optimization through model-based reinforcement learning with experience exploiting and meta-learning
SOFT COMPUTING(2023)
摘要
Hyperparameter optimization plays a significant role in the overall performance of machine learning algorithms. However, the computational cost of algorithm evaluation can be extremely high for complex algorithm or large dataset. In this paper, we propose a model-based reinforcement learning with experience variable and meta-learning optimization method to speed up the training process of hyperparameter optimization. Specifically, an RL agent is employed to select hyperparameters and treat the k -fold cross-validation result as a reward signal to update the agent. To guide the agent’s policy update, we design an embedding representation called “experience variable” and dynamically update it during the training process. Besides, we employ a predictive model to predict the performance of machine learning algorithm with the selected hyperparameters and limit the model rollout in short horizon to reduce the impact of the inaccuracy of the model. Finally, we use the meta-learning technique to pre-train the model for fast adapting to a new task. To prove the advantages of our method, we conduct experiments on 25 real HPO tasks and the experimental results show that with the limited computational resources, the proposed method outperforms the state-of-the-art Bayesian methods and evolution method.
更多查看译文
关键词
Hyperparameters optimization, Reinforcement learning, Meta-learning, Deep learning
AI 理解论文
溯源树
样例
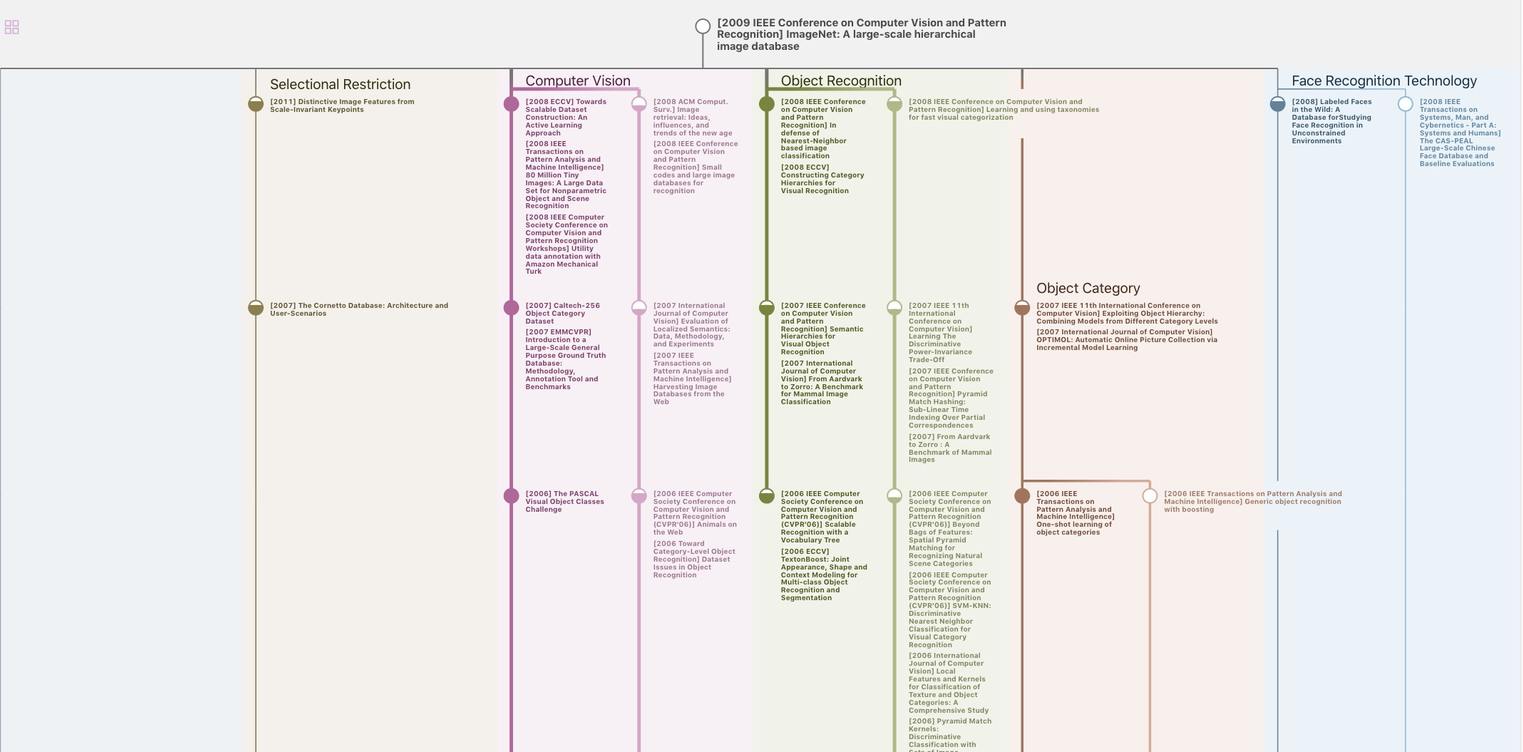
生成溯源树,研究论文发展脉络
Chat Paper
正在生成论文摘要