Machine learning enabled fusion of CAE data and test data for vehicle crashworthiness performance evaluation by analysis
STRUCTURAL AND MULTIDISCIPLINARY OPTIMIZATION(2023)
摘要
Evaluation and potential certification by analysis (CBA) has emerged as a promising tool to reduce costly crashworthiness tests of prototype, by using physics-based simulation and analysis. One of the major obstacles in the CBA implementation for vehicle crashworthiness certification is the discrepancy between computer-aided engineering (CAE) model prediction and the actual response of vehicle crash tests. This paper aims to improve the prediction accuracy of the crashworthiness CAE model under new test speed by fusing CAE data and a small number of crash test data using machine learning models. Two novel approaches are developed in the time domain and the displacement domain to achieve the goal of data fusion for the correction of the CAE model. More specifically, in the time domain (time vs. deceleration), a low-fidelity temporal convolutional network (TCN) is first trained using a large volume of simulation data generated from a CAE model. The low-fidelity TCN model is then fine-tuned to a multi-fidelity TCN through transfer learning using a small number of test data. In the displacement domain (displacement vs. deceleration), the deceleration response as a function of time is first converted into a function of displacement. A Gaussian process regression (GPR) model is then utilized to capture the model bias of the nonlinear spring constant under a dynamic analysis scheme. The learned GPR model finally is integrated into vehicle deceleration dynamic analysis along with the original CAE model to predict vehicle deceleration under a new crash speed. A real-world case study of vehicle crash tests is employed to validate the proposed two approaches. The results show that both proposed approaches in general can recover model bias of the CAE model and give a noticeable improvement in CAE model prediction accuracy.
更多查看译文
关键词
CAE, Model bias, Model updating, Temporal convolutional network, Gaussian process
AI 理解论文
溯源树
样例
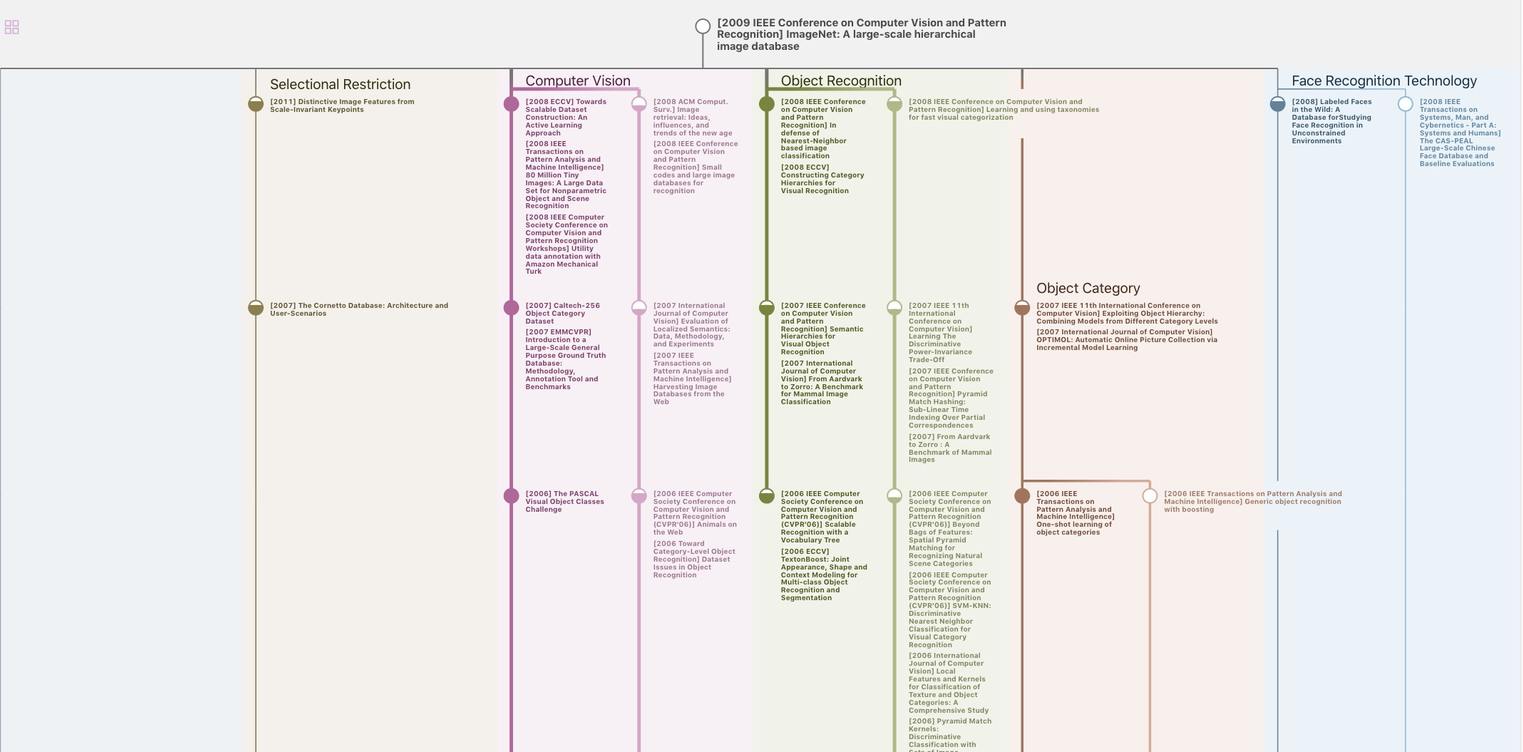
生成溯源树,研究论文发展脉络
Chat Paper
正在生成论文摘要